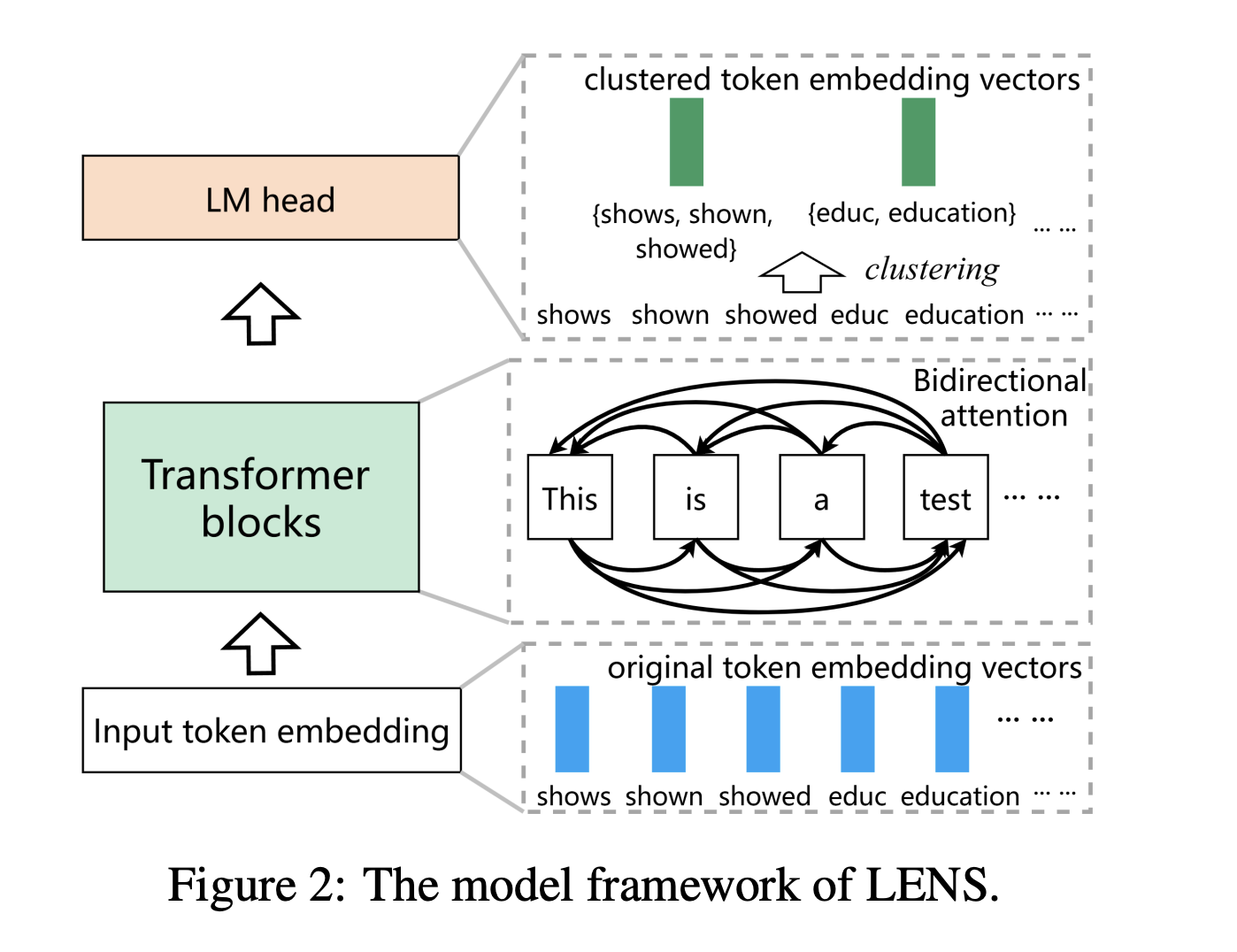
Understanding Lexicon-Based Embeddings
Lexicon-based embeddings offer a promising alternative to traditional dense embeddings, but they have some challenges that limit their use. Key issues include:
- Tokenization Redundancy: Breaking down words into subwords can lead to inefficiencies.
- Unidirectional Attention: Current models can’t fully consider the context around tokens.
These issues hinder the effectiveness of lexicon-based embeddings, especially for tasks beyond simple information retrieval.
Current Solutions and Their Limitations
Some methods have been developed to enhance lexicon-based embeddings:
- SPLADE: Uses bidirectional attention but is limited to smaller models and specific tasks.
- PromptReps: Incorporates prompt engineering but struggles with contextual understanding.
Both methods face high computational costs and inefficiencies, making them less suitable for larger tasks like clustering and classification.
Introducing LENS: A New Approach
The researchers from the University of Amsterdam, University of Technology Sydney, and Tencent IEG have developed LENS (Lexicon-based EmbeddiNgS), a new framework that addresses these limitations.
- Clustering: LENS uses KMeans to cluster similar tokens, reducing redundancy and dimensionality.
- Bidirectional Attention: This allows tokens to utilize context from both sides, enhancing understanding.
- Hybrid Embeddings: Combines features of lexicon-based and dense embeddings for better performance across various tasks.
Key Benefits of LENS
- Efficiency: Produces embeddings that are 4,000 or 8,000 dimensions, comparable to dense embeddings.
- Scalability: The model is designed to be easily scalable for different applications.
- Strong Performance: Excels in tasks like retrieval, clustering, and classification.
Proven Results
LENS has shown impressive results in various benchmarks, including:
- MTEB: Achieved the highest mean score among publicly trained models.
- Strong Performance: Outperformed dense embeddings in several tasks.
Its ability to manage tokenization noise and maintain semantic depth makes it a powerful tool for many applications.
The Future of LENS
LENS represents a significant advancement in lexicon-based embedding models, effectively addressing tokenization issues while improving contextual understanding. Its efficiency and interpretability make it suitable for a wide range of tasks, paving the way for future enhancements, including multi-lingual datasets and larger models.
Get Involved
For more details, check out the research paper. Follow us on Twitter, join our Telegram Channel, and connect with our LinkedIn Group. Join our community of over 65,000 on our ML SubReddit.
Transform Your Business with AI
Enhance your company’s capabilities with AI by:
- Identifying Automation Opportunities: Find key areas for AI implementation.
- Defining KPIs: Measure the impact of AI on your business outcomes.
- Selecting the Right AI Solution: Choose customizable tools that meet your needs.
- Implementing Gradually: Start small, gather data, and expand wisely.
For AI KPI management advice, contact us at hello@itinai.com. Stay updated on AI insights through our Telegram or Twitter.
Explore More AI Solutions
Discover how AI can transform your sales processes and customer engagement at itinai.com.