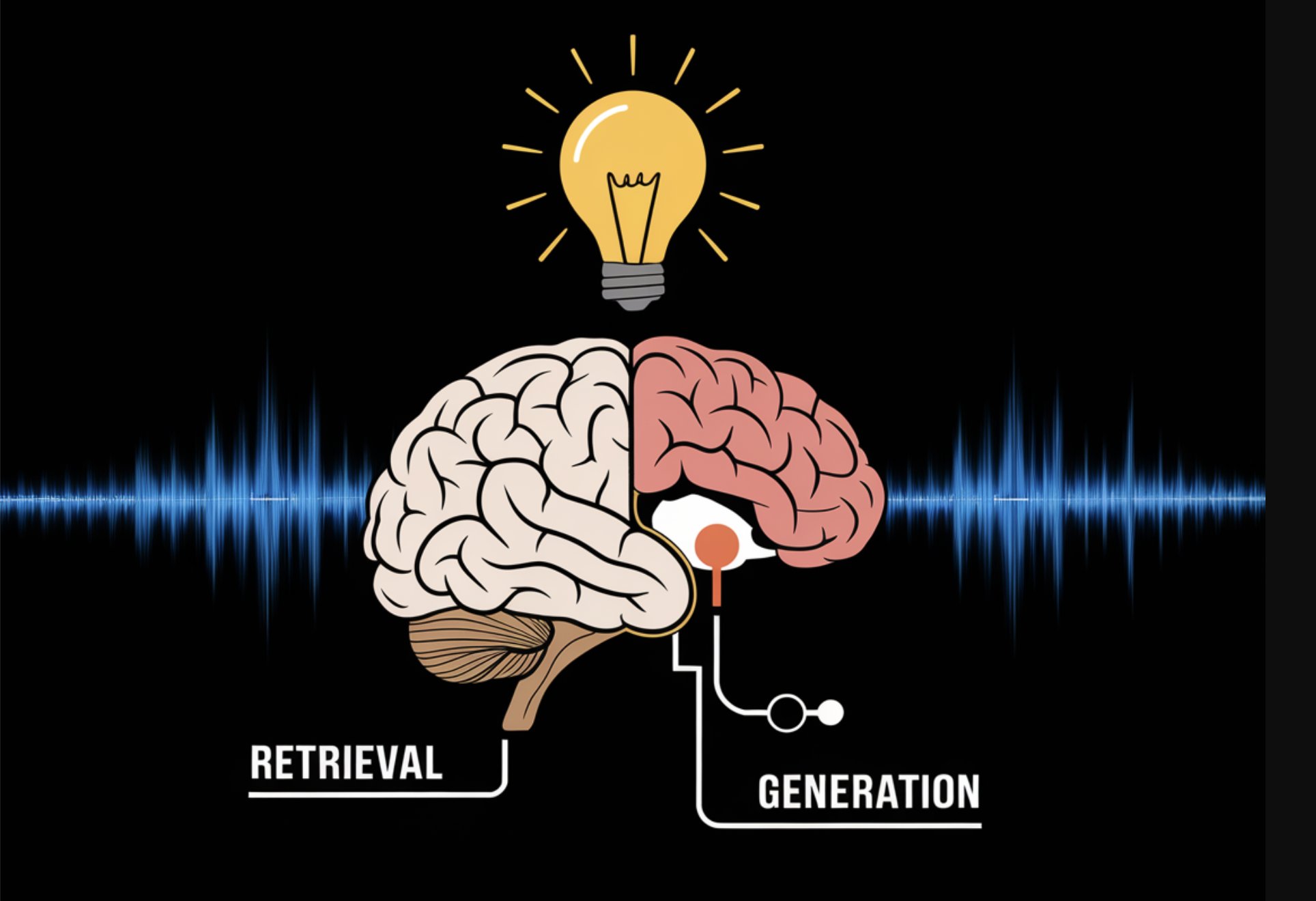
**
Retrieval Augmented Generation (RAG) in AI
**
**
Practical Solutions and Value:
**
Retrieval Augmented Generation (RAG) enhances Large Language Models (LLMs) by referencing external knowledge sources, improving accuracy and relevance of AI-generated text. By combining LLM capabilities with information retrieval systems, RAG ensures more reliable responses in various applications.
**
Architecture of RAG
**
**
Practical Workflow:
**
– RAG retrieves external data based on user query.
– Data is converted into numerical form for AI understanding.
– User query matches with data to provide accurate responses.
– RAG augments user prompt with retrieved data for better answers.
**
Use Cases of RAG in Real-world Applications
**
**
Real-world Applications:
**
– Enhances question-answering systems in healthcare.
– Streamlines content creation and generates concise summaries.
– Improves conversational agents like chatbots and virtual assistants.
– Utilized in knowledge-based search systems, legal research, and education.
**
Key Challenges
**
**
Considerations:
**
– Building and maintaining integrations with 3rd party data.
– Privacy and compliance issues with data sources.
– Latency in responses due to data size and network delays.
– Unreliable data sources leading to false or biased information.
**
Future Trends
**
**
Evolution of RAG:
**
– Multimodal RAG handling various data types.
– Multimodal LLMs improving semantic understanding for better responses.
– Widening AI applications in healthcare, education, and legal research with advanced models.
**
Evolve Your Company with AI
**
**
Advancing with AI:
**
– Identify automation opportunities and define KPIs for impactful AI integration.
– Select AI solutions aligned with business needs and customizable.
– Implement AI gradually starting with pilots and expanding usage strategically.
– Connect with itinai.com for AI KPI management advice and stay updated on leveraging AI.