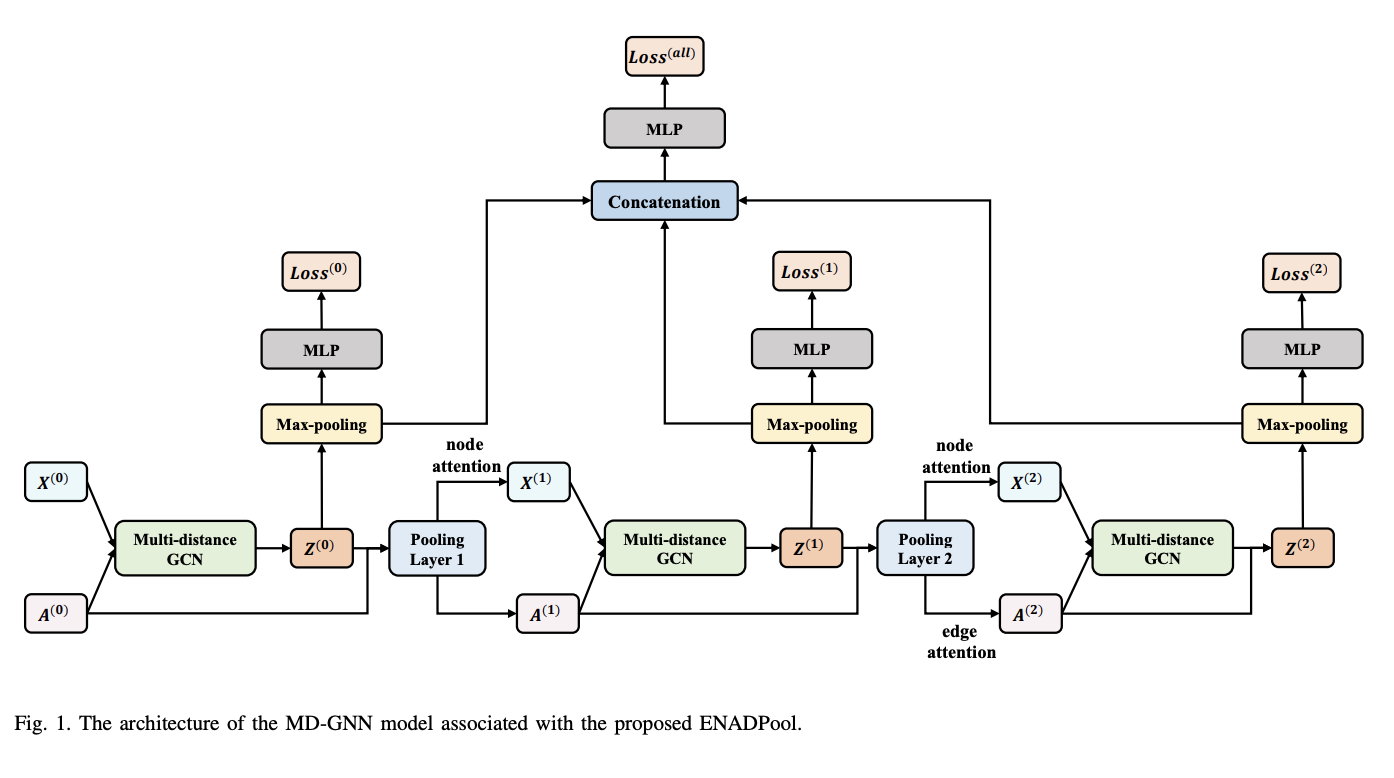
Enhancing Graph Classification with Edge-Node Attention-based Differentiable Pooling and Multi-Distance Graph Neural Networks GNNs
Graph Neural Networks (GNNs) are powerful tools for graph classification, utilizing neighborhood aggregation to update node representations and capture local and global graph structure. Effective graph pooling, essential for downsizing and learning representations, faces challenges like over-smoothing and information loss. Researchers have developed a new hierarchical pooling method called ENADPool, which compresses node features and edge strengths using hard clustering and attention mechanisms, addressing issues with uniform aggregation. The MD-GNN model reduces over-smoothing by allowing nodes to receive information from neighbors at various distances, effectively enhancing graph classification performance.
Comparative Analysis and Performance
The study compares ENADPool and MD-GNN against other graph deep learning methods using benchmark datasets, reporting superior performance due to hard node assignment, attention-based importance for nodes and edges, MD-GNN integration, and effective feature representation.
Practical Implementation and Value
ENADPool compresses node features and edge connectivity into hierarchical structures using attention mechanisms, effectively identifying the importance of nodes and edges. The MD-GNN model mitigates the over-smoothing problem by allowing nodes to receive information from neighbors at various distances.
AI Solutions for Business Transformation
Discover how AI can redefine your way of work. Identify Automation Opportunities, Define KPIs, Select an AI Solution, and Implement Gradually. For AI KPI management advice, connect with us at hello@itinai.com. Spotlight on a Practical AI Solution: Consider the AI Sales Bot from itinai.com/aisalesbot designed to automate customer engagement 24/7 and manage interactions across all customer journey stages.