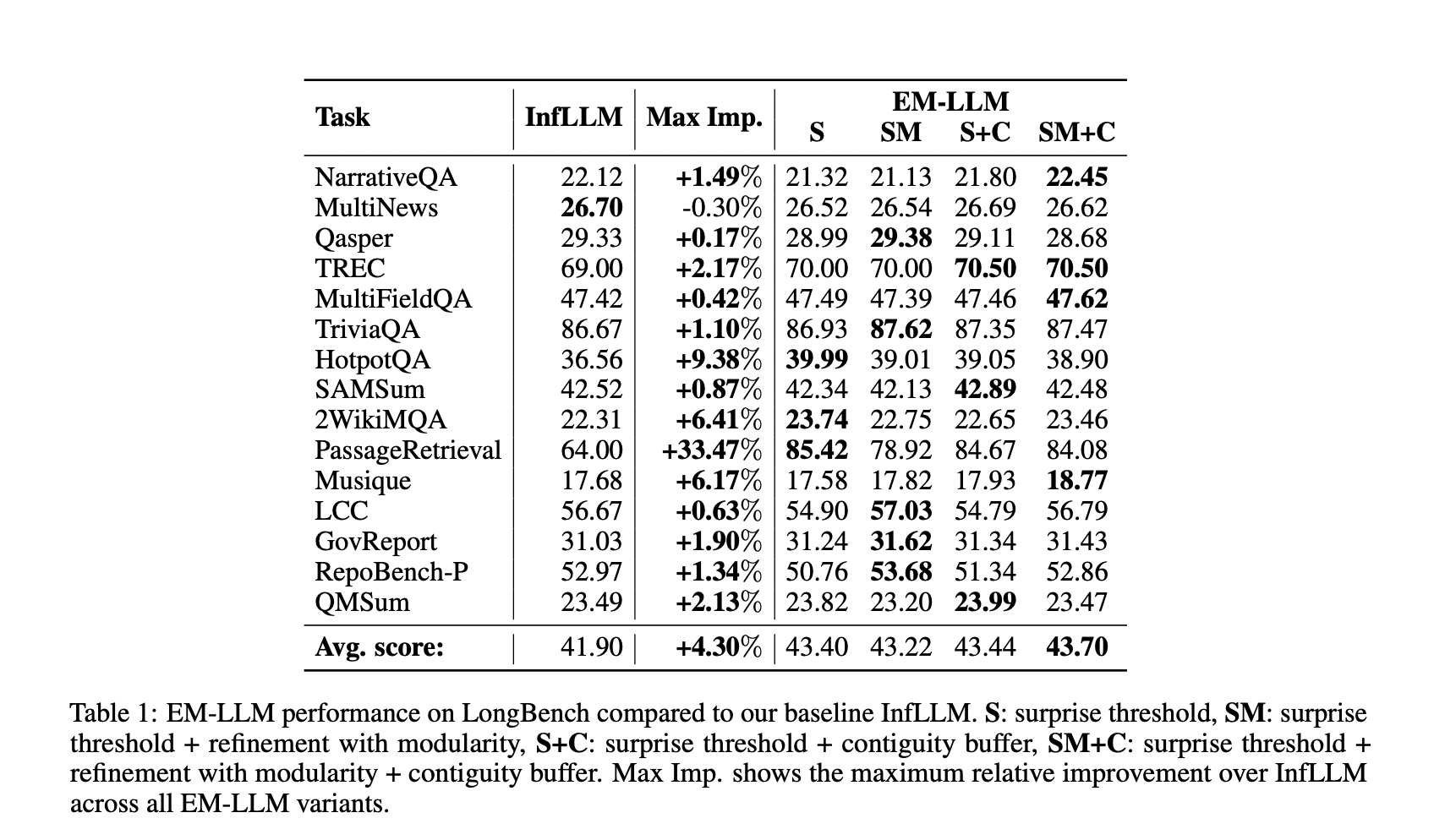
Practical Solutions and Value
Extending Language Models’ Context Windows
Large language models (LLMs) face limitations in processing extensive contexts due to their Transformer-based architectures. These constraints hinder their ability to incorporate domain-specific, private, or up-to-date information effectively.
Improving Long-Context Tasks
Researchers have explored various approaches to extend LLMs’ context windows, focusing on improving softmax attention, reducing computational costs, and enhancing positional encodings. Retrieval-based methods, particularly group-based k-NN retrieval, have shown promise by retrieving large token groups and functioning as hierarchical attention.
Integration of Episodic Memory into LLMs
EM-LLM is a unique architecture that integrates episodic memory into Transformer-based LLMs, enabling them to handle significantly longer contexts. This approach mimics human episodic memory, enhancing the model’s ability to process extended contexts and perform complex temporal reasoning tasks efficiently.
Performance Improvement
EM-LLM demonstrated improved performance on long-context tasks compared to the baseline InfLLM model. It showed significant gains on tasks such as PassageRetrieval and HotpotQA, highlighting its enhanced ability to recall detailed information from large contexts and perform complex reasoning over multiple supporting documents.
Revolutionizing LLM Interactions
EM-LLM offers a path towards virtually infinite context windows, potentially revolutionizing LLM interactions with continuous, personalized exchanges. This flexible framework serves as an alternative to traditional techniques and provides a scalable computational model for testing human memory hypotheses.
AI Transformation and KPI Management
Discover how AI can redefine your way of work, redefine sales processes, and customer engagement. Identify automation opportunities, define KPIs, select an AI solution, and implement gradually. For AI KPI management advice and continuous insights into leveraging AI, connect with us at hello@itinai.com or stay tuned on our Telegram t.me/itinainews and Twitter @itinaicom.