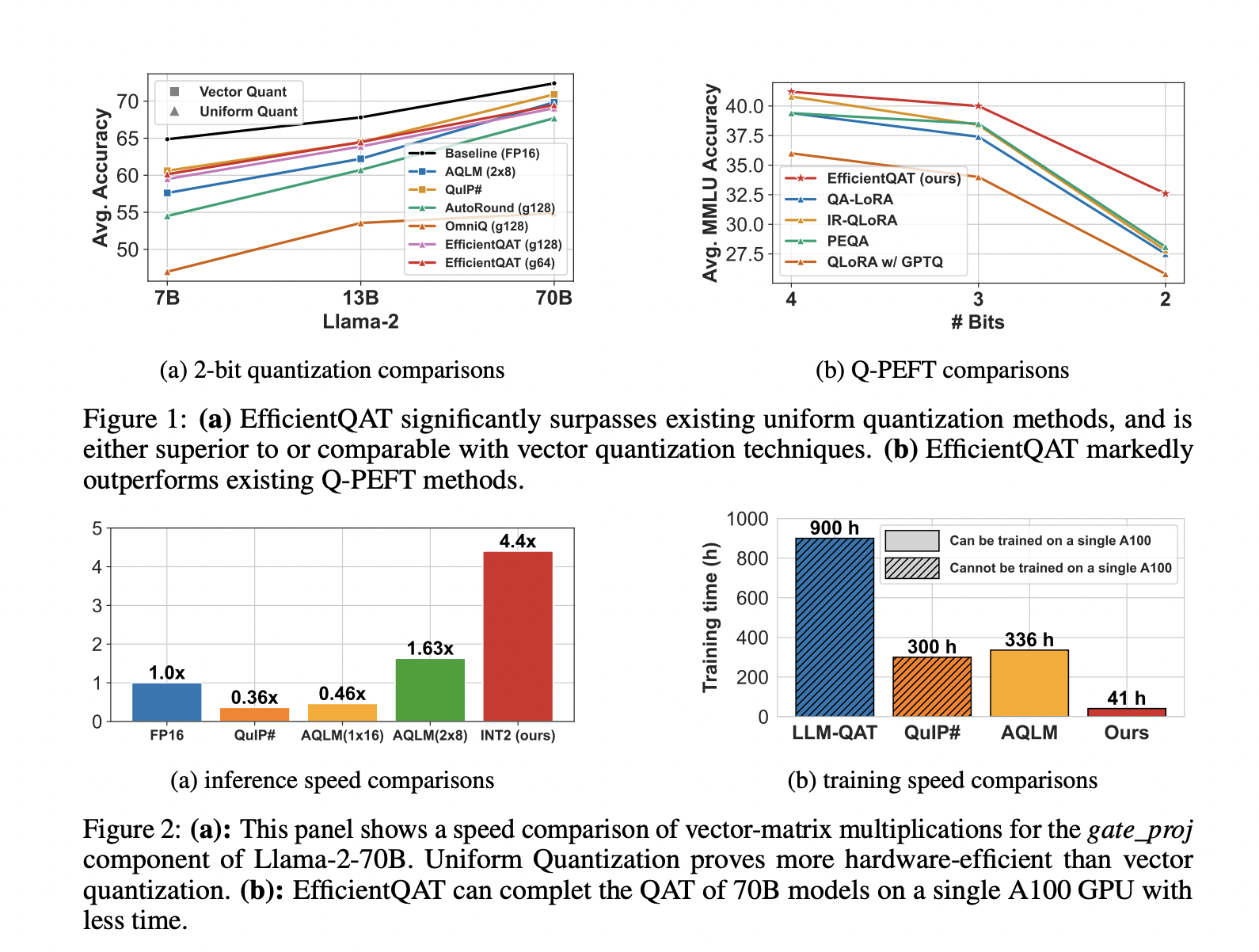
Efficient Quantization-Aware Training (EfficientQAT)
Practical Solutions and Value
As large language models (LLMs) become essential for AI tasks, their high memory requirements and bandwidth consumption pose challenges. EfficientQAT offers a solution by optimizing quantization techniques, reducing memory usage, and improving model efficiency.
EfficientQAT introduces a two-phase training approach, focusing on block-wise training and end-to-end quantization parameter optimization, reducing the resource demands of quantization-aware training while maintaining high performance.
It achieves a 2-bit quantization of a Llama-2-70B model on a single A100-80GB GPU in just 41 hours, with less than 3% accuracy degradation compared to the full-precision model. It outperforms existing methods in low-bit scenarios, providing a more hardware-efficient solution.
Practical Implementation
Identify Automation Opportunities: Locate key customer interaction points that can benefit from AI.
Define KPIs: Ensure your AI endeavors have measurable impacts on business outcomes.
Select an AI Solution: Choose tools that align with your needs and provide customization.
Implement Gradually: Start with a pilot, gather data, and expand AI usage judiciously.
Connect with Us
For AI KPI management advice, connect with us at hello@itinai.com. And for continuous insights into leveraging AI, stay tuned on our Telegram t.me/itinainews or Twitter @itinaicom.
Discover More
If you want to evolve your company with AI, stay competitive, use for your advantage Efficient Quantization-Aware Training (EfficientQAT): A Novel Machine Learning Quantization Technique for Compressing LLMs.
Discover how AI can redefine your sales processes and customer engagement. Explore solutions at itinai.com.