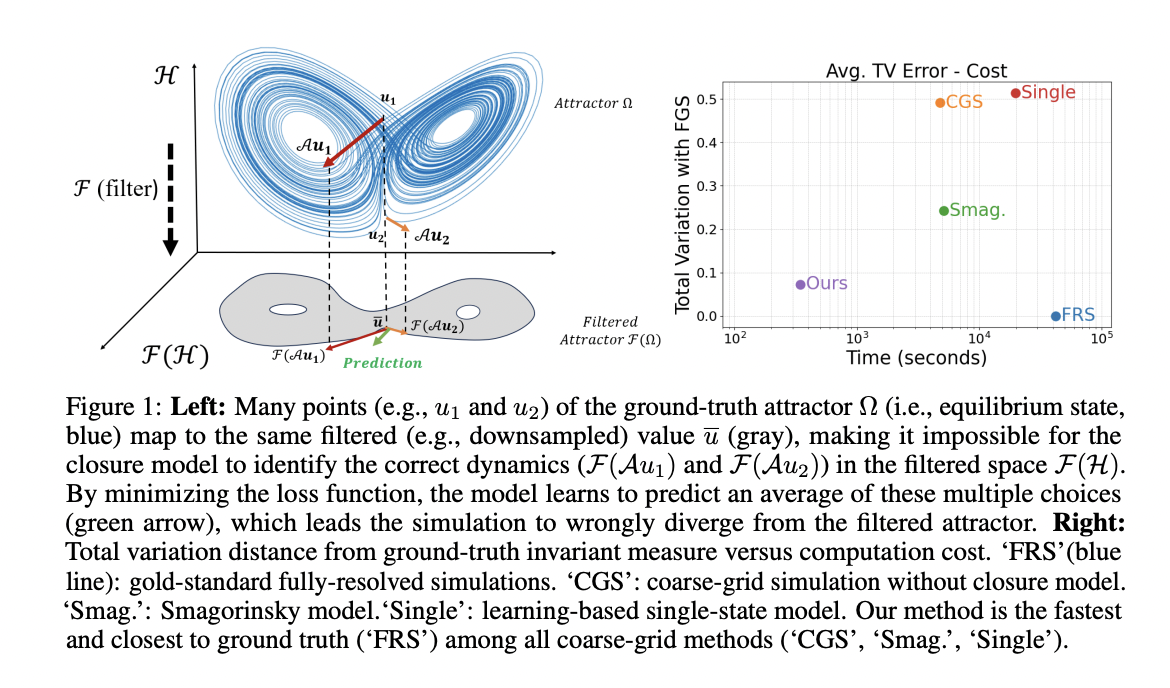
Predicting Long-Term Behavior of Chaotic Systems
Practical Solutions and Value
Predicting the behavior of chaotic systems like climate models requires significant resources. Instead of fully-resolved simulations, using coarse grids with machine learning methods can improve accuracy. Physics-informed neural operators (PINO) eliminate the need for closure models, providing accurate estimates with faster speed and minimal errors.
Efficient Long-Term Prediction of Chaotic Systems Using Physics-Informed Neural Operators
Overcoming Traditional Closure Models
Researchers introduce PINO to accurately predict chaotic system statistics without closure models. PINO offers a 120x speedup with ~5% error, outperforming traditional methods. This approach has broad applications in climate modeling and beyond.
AI Solutions for Business Evolution
Practical Implementation
Identify automation opportunities, define KPIs, select suitable AI tools, and implement gradually. Connect with us for AI KPI management advice at hello@itinai.com. Stay updated on leveraging AI via our Telegram or Twitter channels.