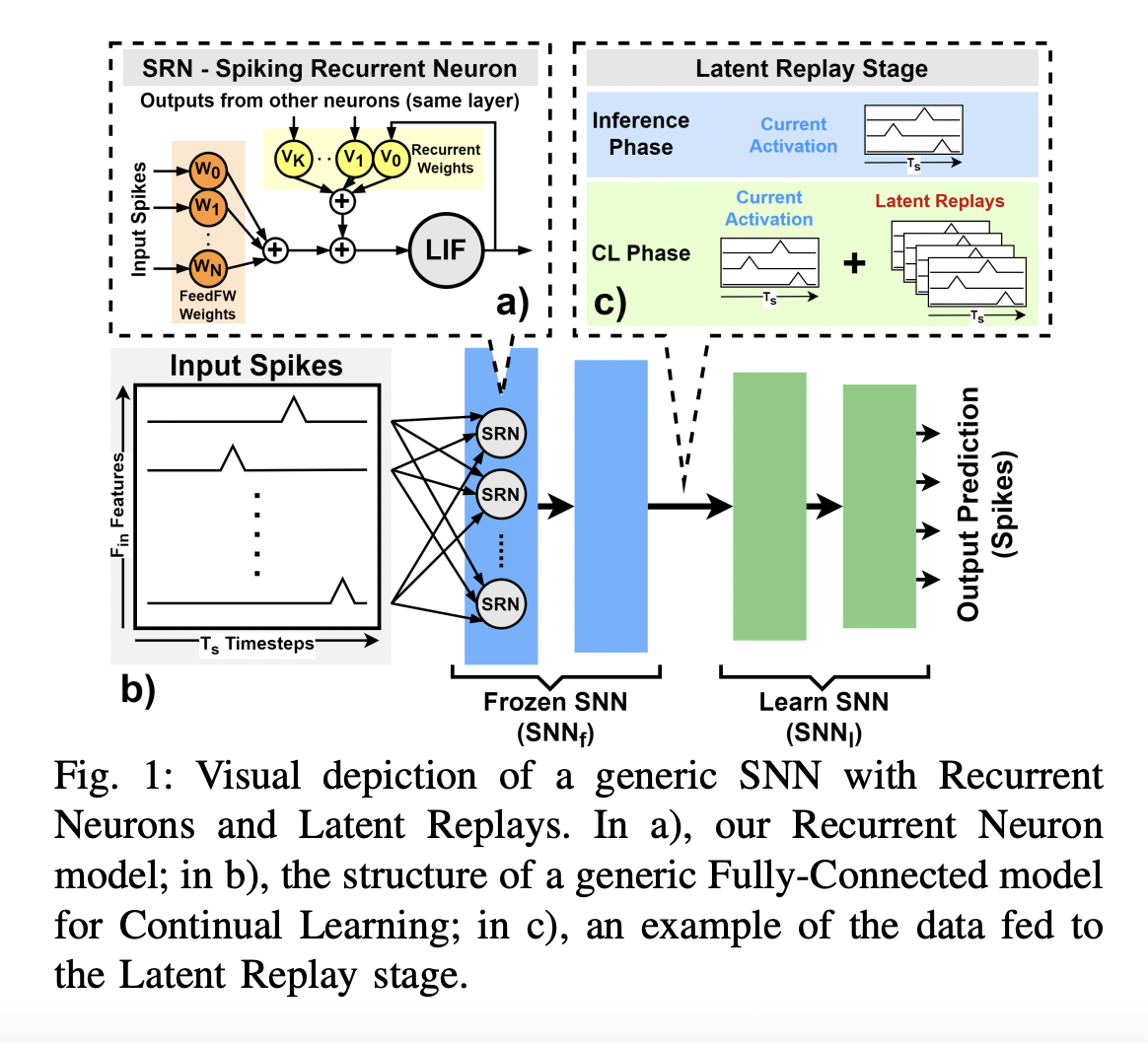
Practical Solutions for Edge AI Challenges
Continuous Learning for Edge AI
Advances in hardware and software enable AI integration into low-power IoT devices, but deploying complex models on these devices requires techniques like quantization and pruning. Shifts in data distribution between training and operational environments also pose challenges for edge AI models. Additionally, AI algorithms need to adapt to individual users while ensuring privacy and reducing internet connectivity.
Spiking Neural Networks (SNNs) for Energy-Efficient Processing
Spiking Neural Networks (SNNs) offer energy-efficient time series processing with great accuracy and efficiency. Using spikes to exchange information, SNNs mimic organic neurons’ activity and can be easily recorded as 1-bit data, opening opportunities for constructing Continuous Learning (CL) solutions. The state-of-the-art implementation of Rehearsal-based CL for SNNs is memory-efficient and designed to work seamlessly with devices with limited resources.
Efficient Continual Learning with Time-Domain Compression
A research team has introduced a state-of-the-art Rehearsal-based CL for SNNs that is memory-efficient. The approach utilizes Latent Replay (LR) to store a subset of past experiences and uses them to train the network on new tasks. This technique has proven to reach state-of-the-art classification accuracy on CNNs. The team’s approach is robust and impressively efficient, achieving high accuracy while consuming minimal memory for rehearsal data.
AI Integration for Business Transformation
Efficient Continual Learning for Spiking Neural Networks with Time-Domain Compression offers practical solutions for businesses to evolve with AI. Companies can leverage AI to redefine their work processes and customer engagement, identifying automation opportunities, defining measurable KPIs, selecting suitable AI solutions, and implementing them gradually for maximum impact on business outcomes.