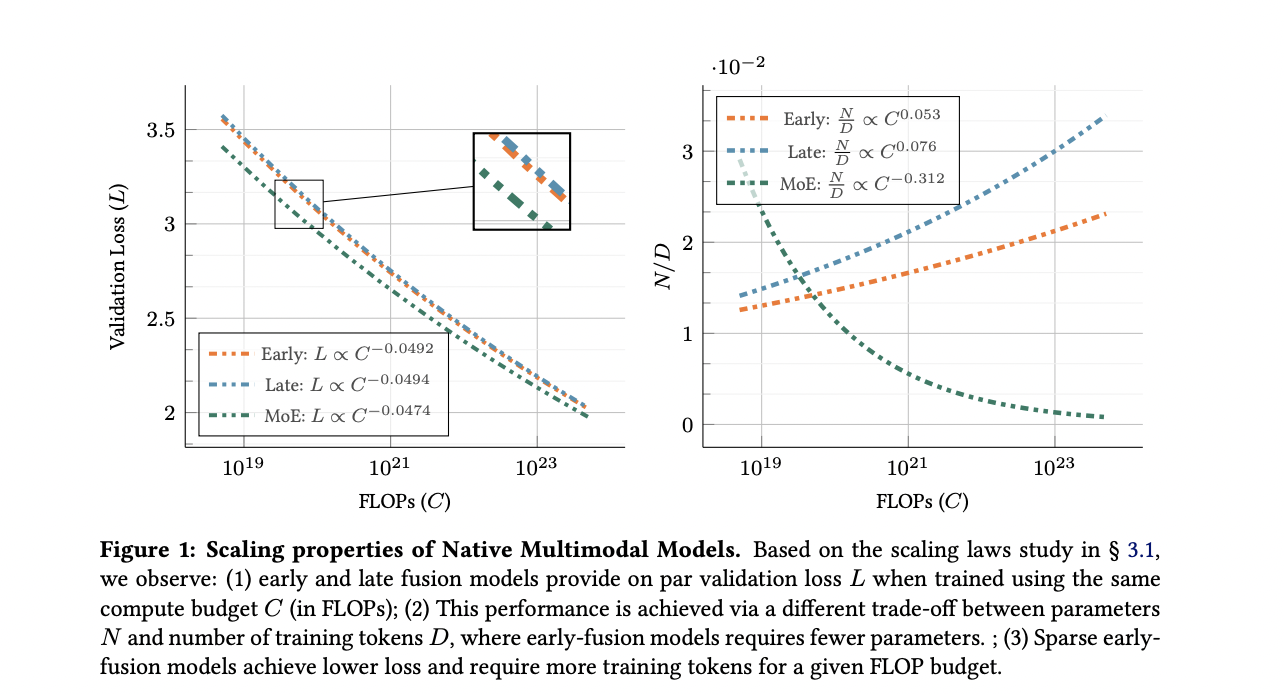
Transforming Multimodal AI: Insights from Apple Researchers
Understanding Multimodal Models
Multimodal artificial intelligence (AI) integrates various types of data, such as text and images, to enhance understanding and decision-making. However, traditional methods often rely on late-fusion strategies, where separate models for each data type are combined after they have been trained independently. This approach can limit the model’s ability to understand the relationships between different data types and complicates scaling due to the need for managing multiple components.
Challenges of Late-Fusion Strategies
Late-fusion models face several challenges:
- Bias from Unimodal Training: Pre-trained models may carry biases that hinder effective multimodal understanding.
- Complexity in Scaling: Each component requires its own hyperparameters and pre-training, complicating resource allocation.
- Performance Limitations: These models may struggle with tasks that require deep reasoning across modalities.
Exploring Early-Fusion Architectures
Recent research from Sorbonne University and Apple suggests that early-fusion architectures, which integrate data types at earlier stages, may offer significant advantages. These models are trained simultaneously on all data types, potentially leading to better performance and easier scalability.
Key Findings from the Research
The study revealed several important insights:
- Efficiency: Early-fusion models are more efficient and easier to scale than late-fusion models.
- Performance Scaling: Both architectures perform similarly when trained from scratch, but early-fusion shows advantages at lower compute budgets.
- Dynamic Parameter Allocation: Sparse architectures using Mixture of Experts (MoE) enhance performance by allowing specialization across modalities.
Case Studies and Statistical Insights
In practical applications, early-fusion models have demonstrated superior performance in various scenarios. For instance, when comparing models with 0.3 billion to 4 billion parameters, early-fusion architectures consistently outperformed their late-fusion counterparts, particularly in tasks requiring nuanced understanding of multimodal data.
Scaling Experiments
The researchers conducted comprehensive scaling experiments, employing structured training methodologies to evaluate performance across different model sizes. Their findings indicate that:
- Sparse early-fusion models follow similar scaling laws to dense models, but with lower overall loss.
- As model size increases, the performance gap between sparse and dense models narrows, yet sparse models maintain an edge in efficiency.
Practical Business Solutions
Businesses looking to leverage AI can consider the following strategies:
- Identify Automation Opportunities: Look for processes that can be automated to enhance efficiency.
- Focus on Key Performance Indicators (KPIs): Establish metrics to measure the impact of AI investments.
- Select Customizable Tools: Choose AI tools that can be tailored to meet specific business objectives.
- Start Small: Begin with pilot projects to gather data and gradually expand AI applications.
Conclusion
The research highlights the potential of early-fusion architectures in multimodal AI, suggesting they are more scalable and efficient than traditional late-fusion approaches. By adopting these innovative strategies, businesses can enhance their AI capabilities, leading to improved decision-making and operational efficiency.
For further guidance on implementing AI in your business, feel free to reach out to us at hello@itinai.ru or connect with us on Telegram, X, and LinkedIn.