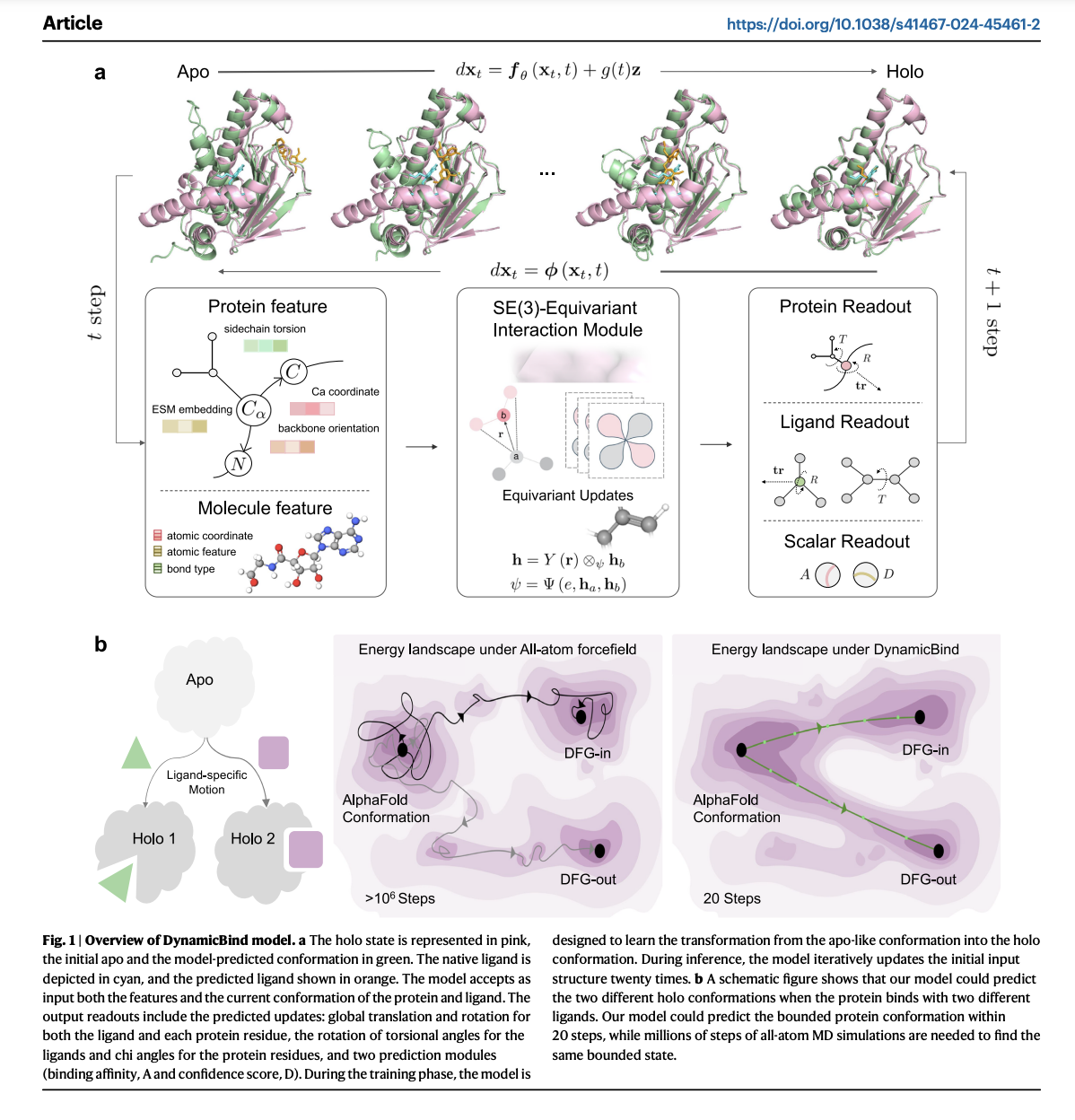
DynamicBind: A Deep Learning Approach for Dynamic Protein-Ligand Docking and Drug Discovery
Practical Solutions and Value
DynamicBind, developed by a collaboration of research institutions, is a deep learning method that accurately predicts ligand-specific protein conformations, enhancing drug discovery and computational screening processes.
It efficiently adjusts protein conformations from initial AlphaFold predictions to holo-like states, handling significant conformational changes better than traditional methods. DynamicBind achieves this by learning a funneled energy landscape that minimizes frustration during transitions between biologically relevant states.
The model is an E(3)-equivariant, diffusion-based graph neural network using a coarse-grained representation to predict protein-ligand binding conformations. It efficiently transforms input structures to account for 3D changes, outperforming traditional methods with less data.
DynamicBind is a versatile tool for predicting protein-ligand complex structures, adept at accommodating significant protein conformational changes. During inference, it gradually adjusts ligand positions and internal angles over iterations while also adapting protein conformations, particularly side-chain angles, improving upon AlphaFold-predicted structures.
DynamicBind integrates protein conformation generation and ligand pose prediction into a single, end-to-end deep learning framework, significantly faster than traditional MD simulations. It performs global docking, ideal for identifying cryptic pockets, reducing potential side effects and aiding drug discovery.
Improvements are needed for better generalization to proteins with low sequence homology. Advances in Cryo-EM and incorporating binding affinity data can enhance DynamicBind’s capabilities.
For more information, check out the Paper and GitHub.