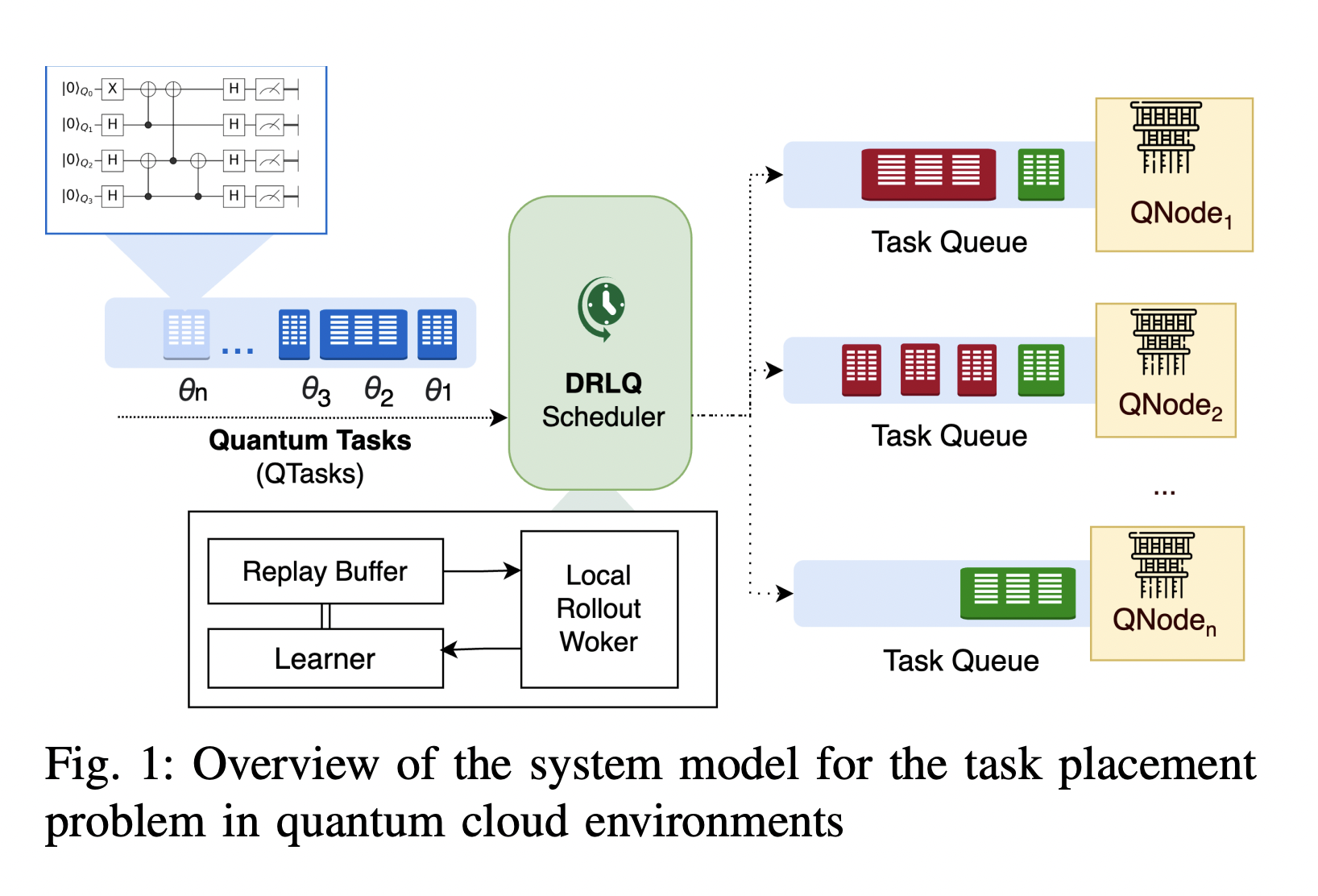
The Value of DRLQ in Quantum Cloud Computing Environments
Challenges in Quantum Computing
The traditional heuristic approach struggles to manage tasks in the evolving quantum computing landscape, leading to inefficiencies in task scheduling and resource management.
Practical Solution
DRLQ, a Deep Reinforcement Learning-based technique, offers a dynamic task placement strategy to optimize quantum task completion time and scheduling efficiency.
Enhanced Resource Management
DRLQ leverages advanced reinforcement learning techniques to continuously interact with the quantum computing environment, enhancing task completion efficiency and reducing the need for rescheduling.
Experimental Results
Experiments demonstrate that DRLQ significantly improves task execution efficiency, reducing total quantum task completion time by 37.81% to 72.93% compared to other heuristic approaches and minimizing the need for task rescheduling.
Impact and Future Prospects
DRLQ presents an innovative approach for efficient quantum cloud resource management, enabling adaptive learning and decision-making in quantum cloud computing environments.
AI Solutions for Business Transformation
Unlocking AI’s Potential
Identify automation opportunities, define KPIs, select suitable AI solutions, and implement gradually to leverage AI for business transformation.
Connect with Us
For AI KPI management advice and continuous insights into leveraging AI, connect with us at hello@itinai.com or stay tuned on our Telegram t.me/itinainews or Twitter @itinaicom.
Explore AI Solutions
Discover how AI can redefine your sales processes and customer engagement. Explore solutions at itinai.com.