The text is a collaboration with Ankur Goyal and Karthikeyan Chokappa from PwC Australia’s Cloud & Digital business, discussing the integration of artificial intelligence and machine learning into systems and processes. It emphasizes the challenges of deploying machine learning models at scale and introduces PwC’s Machine Learning Ops Accelerator, which automates the deployment and maintenance of ML models. Built on AWS native services, the solution enables continuous integration, delivery, training, and monitoring for ML use cases. The comprehensive solution features modular architecture, infrastructure as code, and seamless integration with AWS SageMaker, enabling organizations to streamline their MLOps journey and gain more value from ML models.
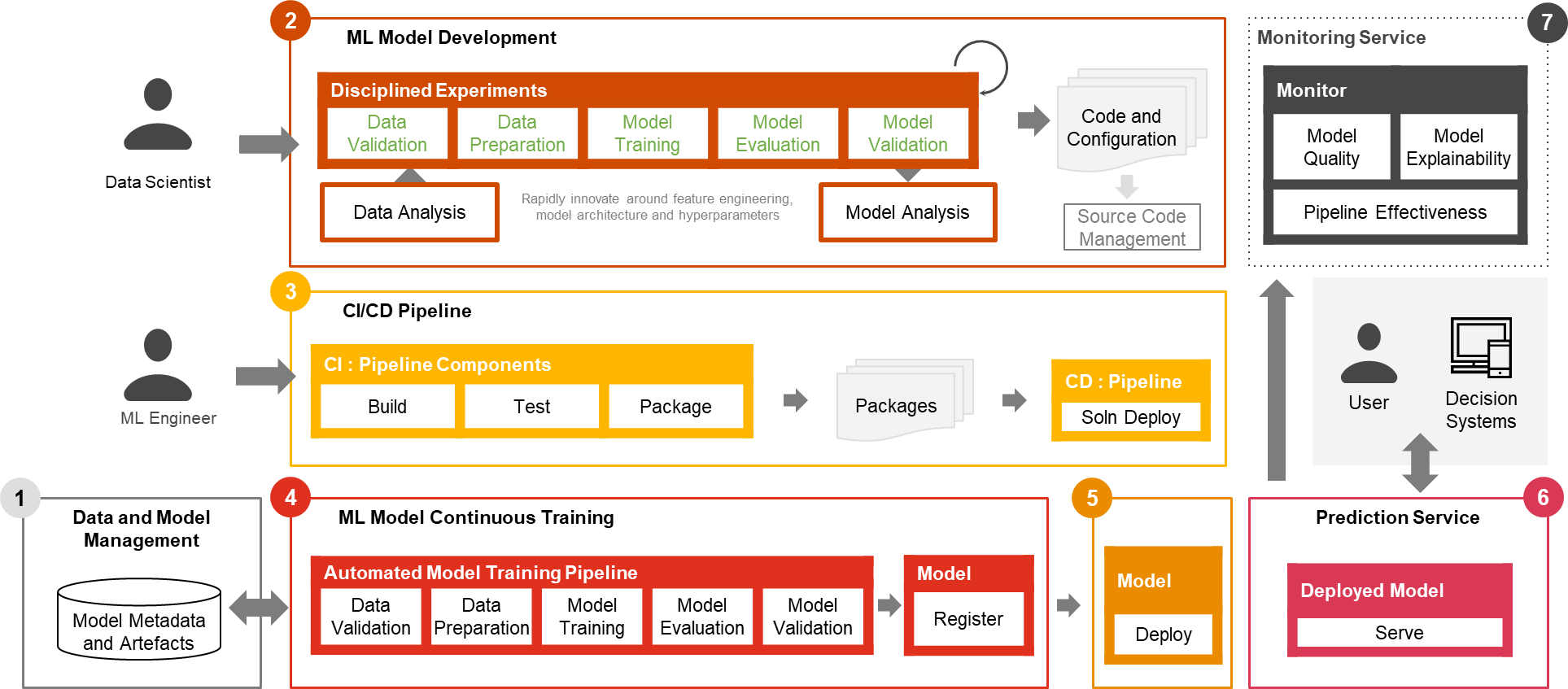
“`html
Artificial Intelligence and Machine Learning in Business
Artificial intelligence (AI) and machine learning (ML) are crucial for driving top and bottom-line improvements across organizations. However, putting ML models into production at scale is challenging. Manual workflows slow down the development process, increase costs, and compromise the quality of the final product.
Introducing Machine Learning Ops (MLOps)
Machine learning operations (MLOps) applies DevOps principles to ML systems, enabling the industrialization and streamlining of the process from development to production deployment at scale. PwC Australia’s Machine Learning Ops Accelerator provides standardized processes and technology capabilities to improve the operationalization of AI/ML models.
Solution Overview
PwC’s solution comprises seven key integrated capabilities and iterative steps, enabling continuous integration, continuous delivery, continuous training, and continuous monitoring of ML use cases. The solution is built on top of AWS-native services, delivering a fit-for-purpose solution that easily integrates into ML use cases across all industries.
PwC Machine Learning Ops Accelerator Architecture
The solution uses Amazon SageMaker and serverless technology to keep performance and scalability high and running costs low. It provides persona-driven access entitlement for ML engineers and data scientists to automate deployment of pipelines and rapidly respond to model quality changes.
Solution Walkthrough
The solution is driven from a configuration YAML file per model, containing all the details required to run the solution. ML engineers can configure the file and trigger the MLOps pipeline, automating the building, deployment, and maintenance of ML models.
Conclusion
MLOps is critical for any organization aiming to deploy ML models in production systems at scale. PwC’s solution, powered by AWS native ML services, helps adopt MLOps practices to speed up the AI journey and gain more value from ML models.
About the Authors
Meet the experts behind PwC’s Machine Learning Ops Accelerator and learn how they are driving innovation and modernization in AI and ML.
AI Solutions for Your Business
Discover how AI can redefine your way of work and redefine your sales processes and customer engagement. Connect with us to explore AI solutions and identify automation opportunities.
Spotlight on a Practical AI Solution
Consider the AI Sales Bot designed to automate customer engagement 24/7 and manage interactions across all customer journey stages.
“`