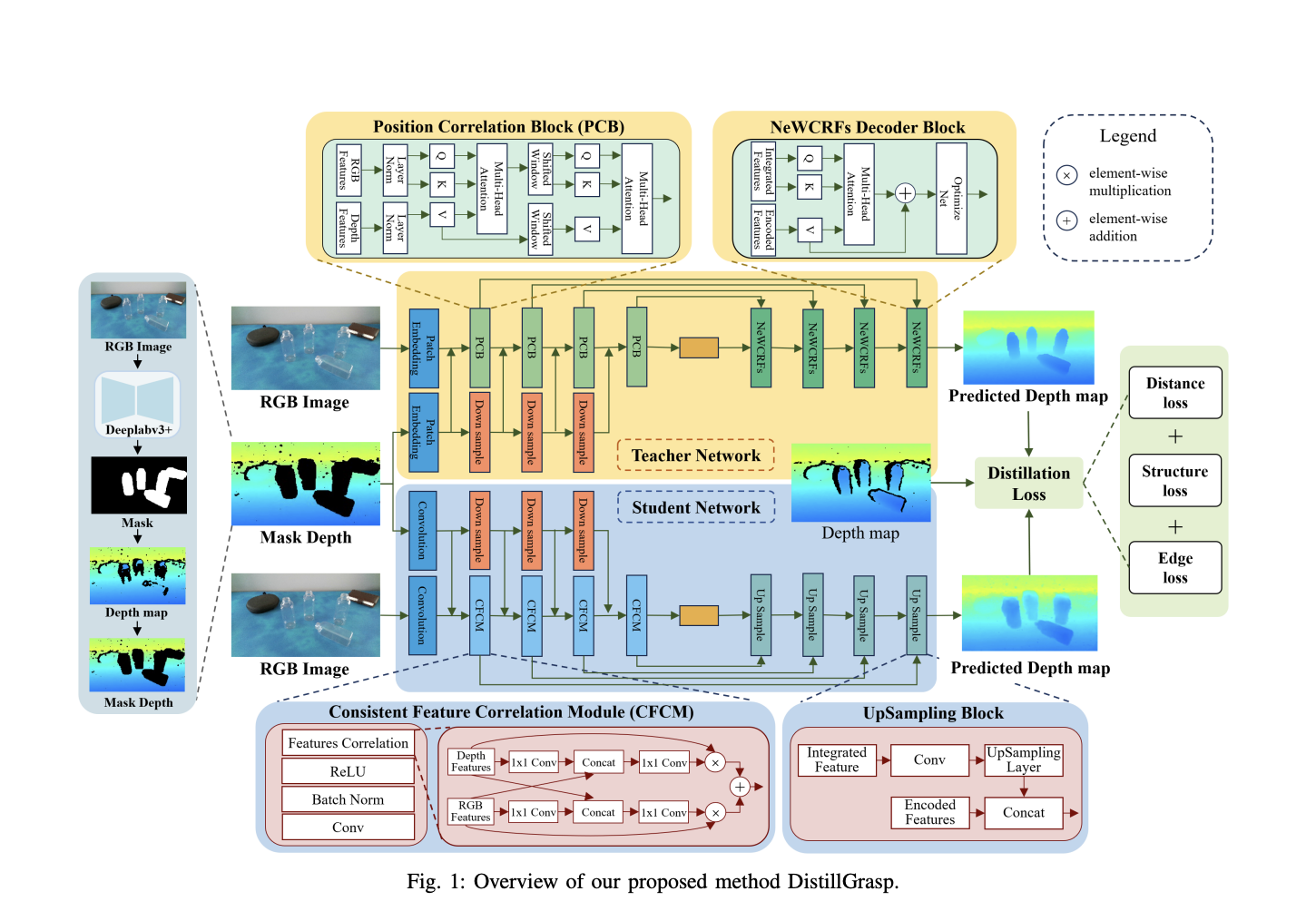
DistillGrasp: A Unique AI Method for Integrating Features Correlation with Knowledge Distillation for Depth Completion of Transparent Objects
Practical Solutions and Value
RGB-D cameras struggle with accurately capturing the depth of transparent objects due to optical effects, leading to inaccurate or missing depth maps. DistillGrasp offers a unique method to efficiently complete depth maps by transferring information from a high-capacity teacher network to a more efficient student network. This approach improves accuracy, generalization, and computational efficiency, making it useful for robotic grasping systems.
Key Contributions
The method applies knowledge distillation to enhance depth completion precision, trains a more effective student network, and implements unique approaches to maintain efficiency without losing performance. It also uses a composite distillation loss to close the performance difference between the student and teacher networks.
Application and Impact
Extensive testing on the ClearGrasp dataset has shown that the teacher network outperforms state-of-the-art techniques in accuracy and generalization, while the student network operates at a rapid 48 frames per second (FPS) and produces competitive results. The successful application of DistillGrasp on a UR10e robot for gripping transparent objects demonstrates its effectiveness.
Evolve Your Company with AI
If you want to evolve your company with AI, stay competitive, and use DistillGrasp to redefine your way of work. Identify automation opportunities, define KPIs, select an AI solution, and implement gradually to benefit from AI. For AI KPI management advice and continuous insights into leveraging AI, connect with us at hello@itinai.com and stay tuned on our Telegram t.me/itinainews or Twitter @itinaicom.