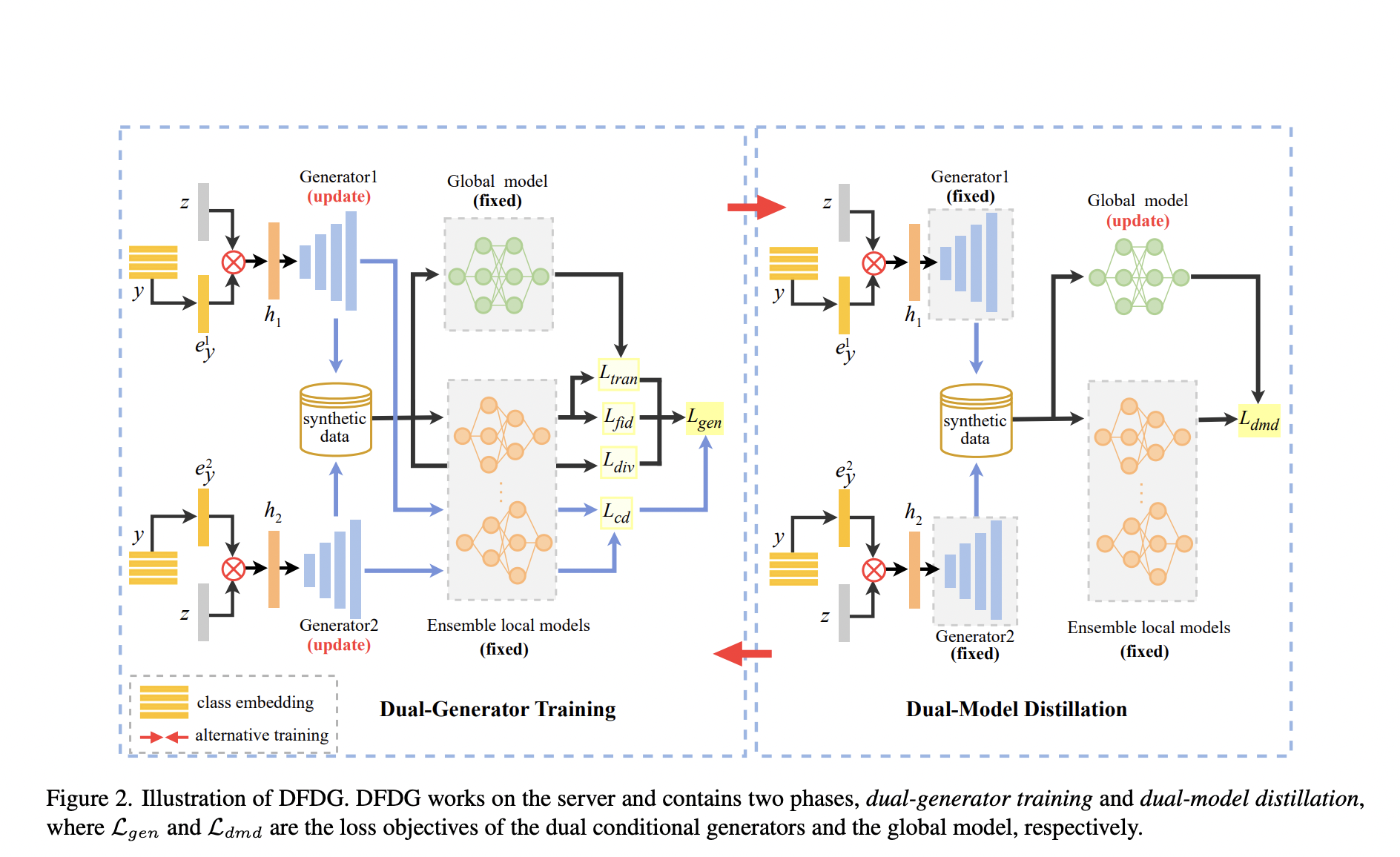
Data-Free Knowledge Distillation (DFKD) and One-Shot Federated Learning (FL) Solutions
Data-Free Knowledge Distillation (DFKD)
DFKD methods transfer knowledge without real data, using synthetic data generation. Non-adversarial methods create data resembling the original, while adversarial methods explore distribution spaces.
One-Shot Federated Learning (FL)
FL addresses communication and security challenges, enabling collaborative model training with a single round. Traditional methods face limitations like the need for public datasets and focus on homogeneous settings.
DFKD Challenges and Solutions
Existing approaches like DENSE address data heterogeneity but struggle with limited knowledge extraction. Innovative solutions like DFDG aim to enhance model training in federated settings by improving synthetic data generation quality.
DFDG Method Overview
DFDG employs dual generators to expand training space exploration, focusing on fidelity, transferability, and diversity. It minimizes generator output overlap and aims to enhance global model training in federated settings.
DFDG Experimental Results
DFDG outperforms baselines in one-shot federated learning across various scenarios, achieving accuracy improvements in image classification datasets. It effectively addresses data and model heterogeneity challenges.
Conclusion
DFDG introduces a novel method for one-shot federated learning, emphasizing generator training and distillation techniques. It enhances model performance and addresses data privacy challenges, validated through extensive experiments.
AI Implementation Tips
Identify automation opportunities, define KPIs, select suitable AI tools, implement gradually, and connect with us for AI KPI management advice.
Discover AI Solutions
Explore how AI can redefine your work processes and customer engagement. Visit itinai.com for more information.