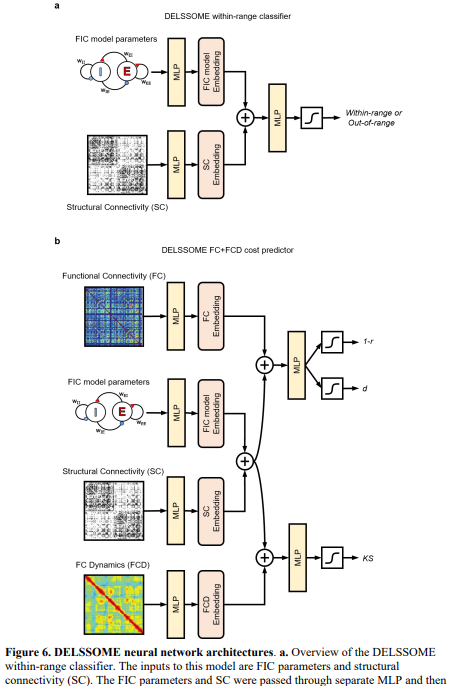
Revolutionizing Biophysical Brain Modeling with DELSSOME
Introduction to Biophysical Brain Models
Biophysical brain models are essential for understanding the intricate workings of the brain. They connect cellular neural dynamics to large-scale brain activity, using parameters that can often be measured through experiments. However, some parameters remain unknown, necessitating complex tuning processes to align simulations with real-world data, such as resting-state fMRI. Traditional optimization methods, including exhaustive searches and gradient descent, are computationally intensive and can hinder scalability, particularly in models with numerous parameters or brain regions.
Challenges in Traditional Optimization
Many researchers simplify their models by tuning only a few parameters or assuming uniform properties across brain regions, which can compromise biological realism. Recent advancements have aimed to improve this by incorporating spatial heterogeneity in cortical properties and employing advanced optimization techniques. Despite these efforts, the high computational cost associated with integrating differential equations during optimization remains a significant bottleneck.
Introducing DELSSOME
Researchers from the National University of Singapore, the University of Pennsylvania, and Universitat Pompeu Fabra have developed DELSSOME (Deep Learning Surrogate Statistics Optimization in Mean Field Modeling). This innovative framework replaces traditional numerical integration with a deep learning model that predicts biologically realistic brain dynamics based on specific parameters.
Key Features of DELSSOME
- Speed Improvement: DELSSOME achieves a remarkable 2000× speedup in computations while maintaining accuracy.
- Integration with Evolutionary Optimization: When combined with evolutionary optimization techniques, DELSSOME provides an additional 50× speedup.
- Generalization Across Datasets: The framework can be applied to various datasets, such as HCP and PNC, without requiring further tuning.
Case Study: Application of DELSSOME
The study utilized neuroimaging data from the Human Connectome Project (HCP) and the Pediatric Neuroimaging Consortium (PNC). By processing resting-state fMRI and diffusion MRI scans, researchers computed functional connectivity (FC), functional connectivity dynamics (FCD), and structural connectivity (SC) matrices. DELSSOME was designed with two components: a classifier to predict if firing rates are biologically plausible and a cost predictor to estimate discrepancies between simulated and empirical data.
Impact on Neuroscience Research
The FIC model, which simulates the activity of excitatory and inhibitory neurons, was optimized using the CMA-ES algorithm. By introducing DELSSOME, researchers significantly reduced the computational costs associated with evaluating numerous parameter sets, achieving a 2000× speedup in evaluation and a 50× speedup in optimization while maintaining accuracy comparable to traditional methods.
Conclusion
In summary, DELSSOME represents a groundbreaking advancement in biophysical brain modeling. By leveraging deep learning to predict surrogate statistics rather than relying on time series data, this framework offers a scalable solution for population-level neuroscience studies. With its ability to generalize across datasets and maintain model accuracy, DELSSOME paves the way for more efficient and biologically grounded research in neuroscience.
For businesses looking to harness the power of artificial intelligence, consider exploring how similar technologies can streamline operations, enhance customer interactions, and improve decision-making processes. Start small, measure effectiveness, and gradually expand your AI initiatives for maximum impact.