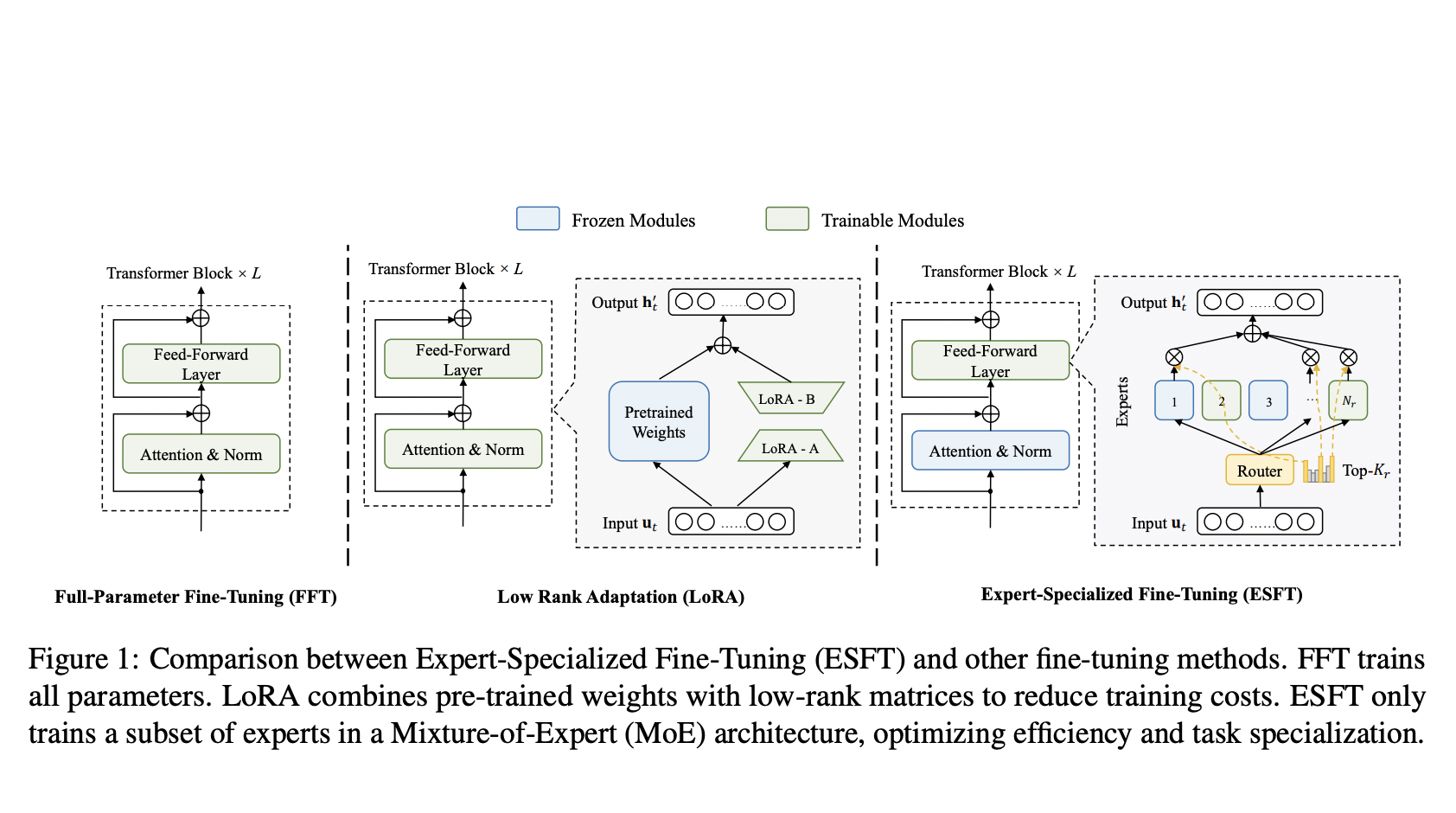
Natural Language Processing Advancements
Optimizing Large Language Models for Specific Tasks
Natural language processing is rapidly advancing, with a focus on optimizing large language models (LLMs) for specific tasks.
Parameter-Efficient Fine-Tuning
The challenge lies in developing innovative approaches to parameter-efficient fine-tuning (PEFT) to maximize performance while minimizing resource usage.
Practical Solutions and Value
ESFT reduces memory by up to 90% and time by up to 30%, demonstrating significant improvements in training efficiency and reduced computational costs.
Expert-Specialized Fine-Tuning (ESFT)
Enhancing Tuning Efficiency
ESFT fine-tunes only the most relevant experts for a given task while freezing the other experts and model components, enhancing tuning efficiency and maintaining expert specialization.
Selective Fine-Tuning
ESFT involves selecting a subset of experts with the highest relevance to the task, significantly reducing computational costs associated with fine-tuning.
Future Developments in Customizing Large Language Models
Promise of ESFT
ESFT is a promising approach for future developments in customizing large language models, enabling superior results with reduced computational costs.
AI Solutions for Business Transformation
Identify Automation Opportunities
Locate key customer interaction points that can benefit from AI.
Define KPIs
Ensure AI endeavors have measurable impacts on business outcomes.
Select an AI Solution
Choose tools that align with your needs and provide customization.
Implement Gradually
Start with a pilot, gather data, and expand AI usage judiciously.
AI KPI Management Advice
Connect with Us
For AI KPI management advice, connect with us at hello@itinai.com.
Leveraging AI for Sales Processes and Customer Engagement
Explore Solutions
Explore solutions for redefining sales processes and customer engagement at itinai.com.