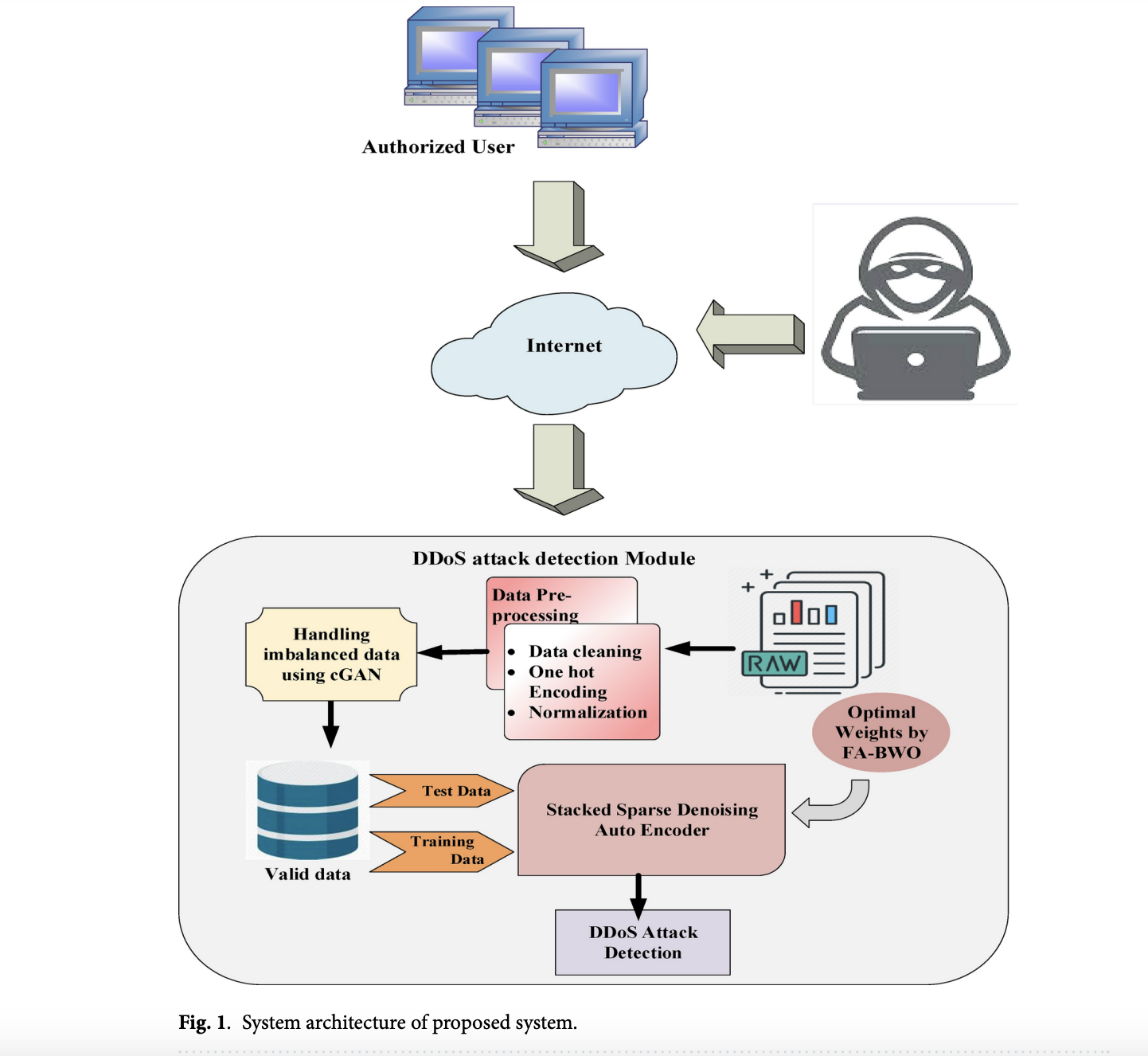
The Rise of Cybersecurity Threats
With the growing number of websites, cybersecurity threats are increasing significantly. Cyber-attacks are becoming more complex and frequent, putting network infrastructure and digital systems at risk. Unauthorized access and intrusive actions are common, threatening the security of networks.
Importance of Network Intrusion Detection Systems (NIDS)
Network Intrusion Detection Systems (NIDS) are crucial for tackling these challenges. DDoS (Distributed Denial of Service) attacks are particularly concerning, as they can quickly overwhelm network resources, making systems inaccessible to legitimate users. This highlights the need for strong and adaptable cybersecurity solutions.
Innovative Techniques for Intrusion Detection
Researchers are developing various techniques to improve intrusion detection:
- BAT Method: Combines attention mechanisms with Bidirectional Long Short-term Memory (BLSTM) to identify key traffic data.
- Multi-Architectural Modular Deep Neural Networks: Reduces false positives in anomaly detection.
- Hybrid Systems: Integrates CNN, fuzzy C-means clustering, genetic algorithms, and classifiers for better detection.
- Semantic Re-encoding Deep Learning Model (SRDLM): Enhances traffic distinguishability and algorithm performance.
Addressing Data Imbalance
Handling imbalanced datasets remains a challenge, often leading to biased results. Advanced feature extraction and classification methods are needed to overcome this issue.
Research Breakthroughs in DDoS Detection
Researchers from various institutions have proposed a hybrid optimization-based deep belief network for detecting DDoS attacks. This method uses a Stacked Sparse Denoising Autoencoder (SSDAE) to learn complex features, improving detection accuracy and speed.
Key Features of the Proposed Model
- Data Preprocessing: Cleans and normalizes data for better analysis.
- Imbalance Processing: Uses a conditional Generative Adversarial Network (cGAN) to create a balanced dataset.
- Classification Decision: Employs SSDAE to extract features and classify data effectively.
Performance Metrics
The proposed model has shown outstanding results:
- Initial experiment (imbalanced data): 99.89% accuracy, 99.24% precision, 99.02% recall.
- After balancing data (using cGAN): 99.99% accuracy, 99.81% precision, 99.26% recall.
The Future of Cybersecurity with AI
This research demonstrates the potential of deep learning in enhancing intrusion detection systems against DDoS attacks. The method achieved remarkable accuracy rates and could be expanded to include multi-attack classification and explainability techniques for better cybersecurity strategies.
Join the Conversation
Check out the research paper for more details. Follow us on Twitter, join our Telegram Channel, and connect with our LinkedIn Group for updates. If you appreciate our work, consider subscribing to our newsletter and joining our 55k+ ML SubReddit.
Upcoming Event: SmallCon
Don’t miss our free virtual conference, SmallCon, on Dec 11th. Learn from AI leaders at Meta, Mistral, Salesforce, and more about building big with small models.
Transform Your Business with AI
Utilize AI to stay competitive and redefine your operations:
- Identify Automation Opportunities: Find key areas for AI implementation.
- Define KPIs: Measure the impact of your AI initiatives.
- Select AI Solutions: Choose tools that fit your needs.
- Implement Gradually: Start small, gather data, and expand wisely.
For AI KPI management advice, contact us at hello@itinai.com. Stay updated on AI insights via our Telegram and Twitter.
Explore how AI can enhance your sales processes and customer engagement at itinai.com.