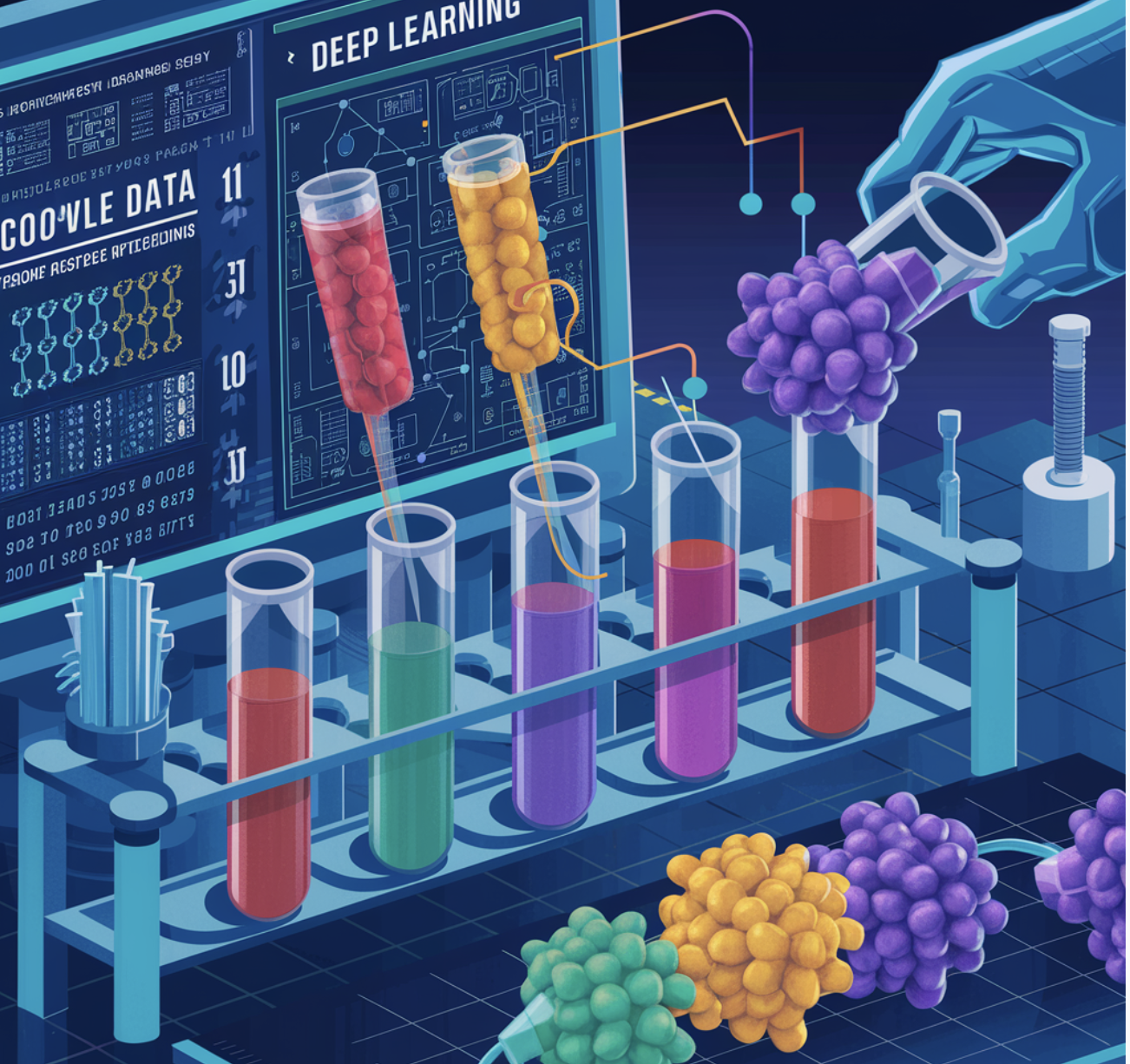
Practical Solutions in Protein Design with Deep Learning
Transforming Protein Design with Deep Learning
Recent advances in deep learning, particularly with tools like AlphaFold2, have transformed protein design by enabling accurate prediction and exploration of vast sequence spaces. This has led to stable proteins with novel functions and intricate structures.
Developing a Deep Learning Pipeline for Protein Design
Researchers have developed a deep learning pipeline to design complex protein folds and soluble analogs of membrane proteins. This approach uses AlphaFold2 and ProteinMPNN to create stable protein structures, including those mimicking membrane proteins like GPCRs, without parametric restraints or extensive experimental optimization.
Integrating AF2seq and ProteinMPNN for Complex Protein Folds
Researchers have developed a deep learning-based pipeline that integrates AF2seq and ProteinMPNN to design complex protein folds, including soluble analogs of membrane proteins. This approach successfully designed intricate structures without traditional parametric constraints, highlighting its potential for exploring new protein topologies and integrating functionalities from membrane proteins.
Designing Soluble Analogues of Membrane Protein Folds
Researchers explored designing soluble analogs of membrane protein folds, aiming to solubilize complex folds like claudin, rhomboid protease, and GPCRs. They achieved soluble, thermally stable proteins with accurate structural alignments for these challenging folds, demonstrating their potential for diverse biotechnological applications.
Preserving Functional Capabilities in Soluble Analogues
The study extended the design of soluble analogs of membrane proteins to include functional capabilities. Researchers preserved specific functional motifs while solubilizing transmembrane segments, creating soluble versions of human claudin-1 and claudin-4 that retained their natural ability to bind Clostridium perfringens enterotoxin, mimicking their membrane-bound counterparts. They also designed chimeric soluble GPCR analogs incorporating functional domains from the ghrelin receptor and adenosine A2A receptor.
Breakthrough in Computational Protein Design
The study showcases a deep learning-based computational approach for designing complex protein folds, overcoming traditional challenges. It successfully generated high-quality protein backbones across various topologies without fold-specific retraining, achieving significant experimental success in producing soluble and properly folded designs. Structural validations confirmed precise modeling accuracy, which is crucial for functional protein design.
Value of AI in Protein Engineering
If you want to evolve your company with AI, stay competitive, use for your advantage Deep Learning in Protein Engineering: Designing Functional Soluble Proteins.
AI for Business Transformation
Discover how AI can redefine your way of work. Identify Automation Opportunities, Define KPIs, Select an AI Solution, and Implement Gradually. For AI KPI management advice, connect with us at hello@itinai.com.
AI for Sales Processes and Customer Engagement
Discover how AI can redefine your sales processes and customer engagement. Explore solutions at itinai.com.