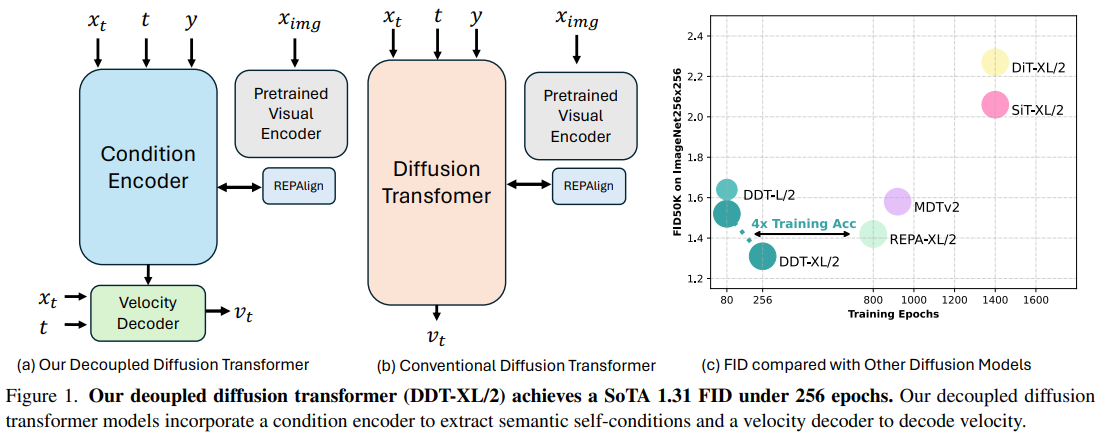
Decoupled Diffusion Transformers: A Business Perspective
Introduction to Diffusion Transformers
Diffusion Transformers have emerged as a leading technology in image generation, outperforming traditional models like GANs and autoregressive architectures. They function by introducing noise to images and then learning to reverse this process, which helps in approximating the underlying data distribution. However, their training is often slow and resource-intensive due to the architecture’s inherent limitations.
Challenges in Current Models
One significant challenge is the optimization conflict that arises when the model attempts to encode low-frequency semantic information while decoding high-frequency details simultaneously. This dual task can hinder performance and slow down the training process.
Innovative Solutions for Efficiency
Recent advancements have focused on enhancing the efficiency of Diffusion Transformers through various strategies:
- Optimized Attention Mechanisms: Techniques like linear and sparse attention reduce computational costs.
- Effective Sampling Techniques: Methods such as log-normal resampling and loss reweighting stabilize the learning process.
- Domain-Specific Inductive Biases: Approaches like REPA, RCG, and DoD improve reasoning capabilities.
- Structured Feature Learning: Masked modeling enhances the model’s ability to learn effectively.
Case Study: Decoupled Diffusion Transformer (DDT)
Researchers from Nanjing University and ByteDance Seed Vision have introduced the Decoupled Diffusion Transformer (DDT), which separates the model into two distinct components: a condition encoder for semantic extraction and a velocity decoder for detailed generation. This innovative design leads to faster convergence and improved sample quality.
In benchmarks on ImageNet, the DDT-XL/2 model achieved state-of-the-art FID scores of 1.31 and 1.28 for 256×256 and 512×512 images, respectively, with training speeds up to four times faster than previous models.
Operational Mechanism of DDT
The DDT architecture allows for separate handling of low- and high-frequency components in image generation:
- The Condition Encoder extracts semantic features from noisy inputs, timesteps, and class labels.
- The Velocity Decoder estimates the velocity field based on these features.
- A shared self-condition mechanism reduces computation by reusing semantic features across denoising steps.
- A dynamic programming approach optimizes the recomputation of features, minimizing performance loss while accelerating the sampling process.
Performance Evaluation
The DDT models were trained on ImageNet with a batch size of 256, utilizing advanced sampling techniques and performance metrics such as FID, sFID, IS, Precision, and Recall. The results showed consistent outperformance compared to prior models, particularly in larger configurations, demonstrating faster convergence and superior image quality.
Conclusion
The Decoupled Diffusion Transformer represents a significant advancement in the field of image generation. By separating the tasks of semantic encoding and high-frequency decoding, the DDT achieves remarkable performance improvements, particularly in larger models. The DDT-XL/2 model sets new benchmarks in training speed and image quality, making it a valuable asset for businesses looking to leverage AI in creative applications.
Next Steps for Businesses
To harness the potential of AI technologies like DDT, businesses should:
- Identify processes that can be automated to enhance efficiency.
- Pinpoint customer interaction moments where AI can add value.
- Establish key performance indicators (KPIs) to measure the impact of AI investments.
- Select customizable tools that align with business objectives.
- Start with small projects, analyze their effectiveness, and gradually expand AI applications.
If you need assistance in integrating AI into your business strategy, please contact us at hello@itinai.ru or connect with us on Telegram, X, and LinkedIn.