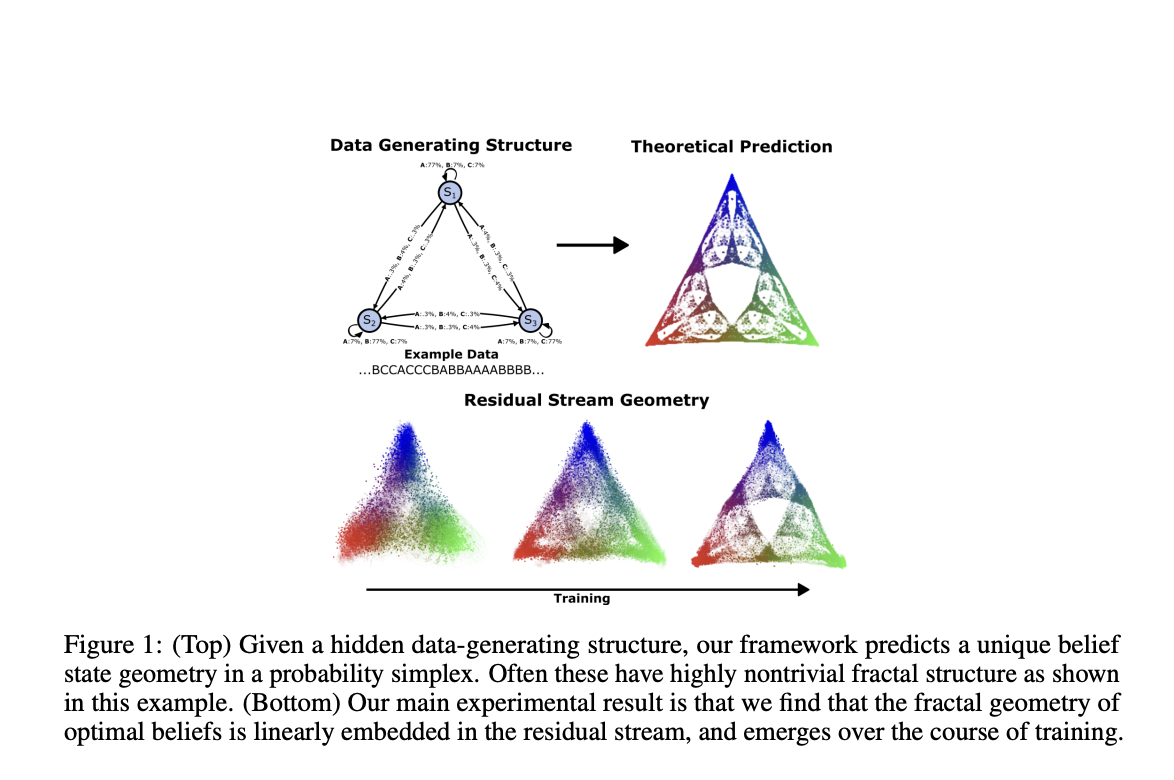
Understanding Transformer Models in AI
The Challenge
In the fast-changing world of machine learning and AI, grasping how transformer models work is essential. Researchers are trying to figure out if transformers act as simple statistical tools, complex world models, or something else entirely. The idea is that transformers may reveal hidden patterns in how data is generated, which helps in predicting the next token in a sequence.
Current Research Insights
Studies have shown that transformer models hold information about future tokens, acting like belief states. They have also been analyzed in games like Othello, where they represent possible game states. However, traditional methods struggle to analyze these complex computational representations effectively.
A New Approach
Researchers from PIBBSS, Pitzer and Scripps College, and University College London have introduced a new method to understand how large language models (LLMs) predict the next token. They focus on how belief states are represented in the model’s hidden layers. Their findings indicate that belief states can be represented linearly in the model’s residual streams, even when the data shows complex structures.
Methodology and Findings
The researchers conducted detailed experiments on transformer models trained with hidden Markov model (HMM) data. They analyzed the activations in different layers and positions, creating a dataset to understand belief states and their probabilities. By using linear regression, they established a connection between the model’s activations and belief state probabilities.
The results showed that transformers can learn to represent complex geometries of belief states, with strong correlations between these geometries and next-token predictions. For example, in the RRXOR process, the correlation was very high (R² = 0.95), indicating that transformers can predict much more than just the next token.
Conclusion and Implications
This research connects the structure of training data with the behavior of transformer models. It demonstrates that these models develop complex predictive capabilities beyond simple token prediction. This understanding can lead to better model interpretability and trustworthiness, enhancing AI applications in various fields.
Get Involved
Check out the full research paper for more details. Follow us on Twitter, join our Telegram Channel, and connect with our LinkedIn Group for updates. If you enjoy our work, subscribe to our newsletter and join our 60k+ ML SubReddit community.
Transform Your Business with AI
To stay competitive and leverage AI effectively, consider the following steps:
– **Identify Automation Opportunities:** Find customer interaction points that can benefit from AI.
– **Define KPIs:** Ensure your AI projects have measurable impacts.
– **Select an AI Solution:** Choose customizable tools that fit your needs.
– **Implement Gradually:** Start with a pilot project, gather data, and expand wisely.
For AI KPI management advice, reach out to us at hello@itinai.com. Stay updated on AI insights via our Telegram channel t.me/itinainews or Twitter @itinaicom. Explore how AI can enhance your sales processes and customer engagement at itinai.com.