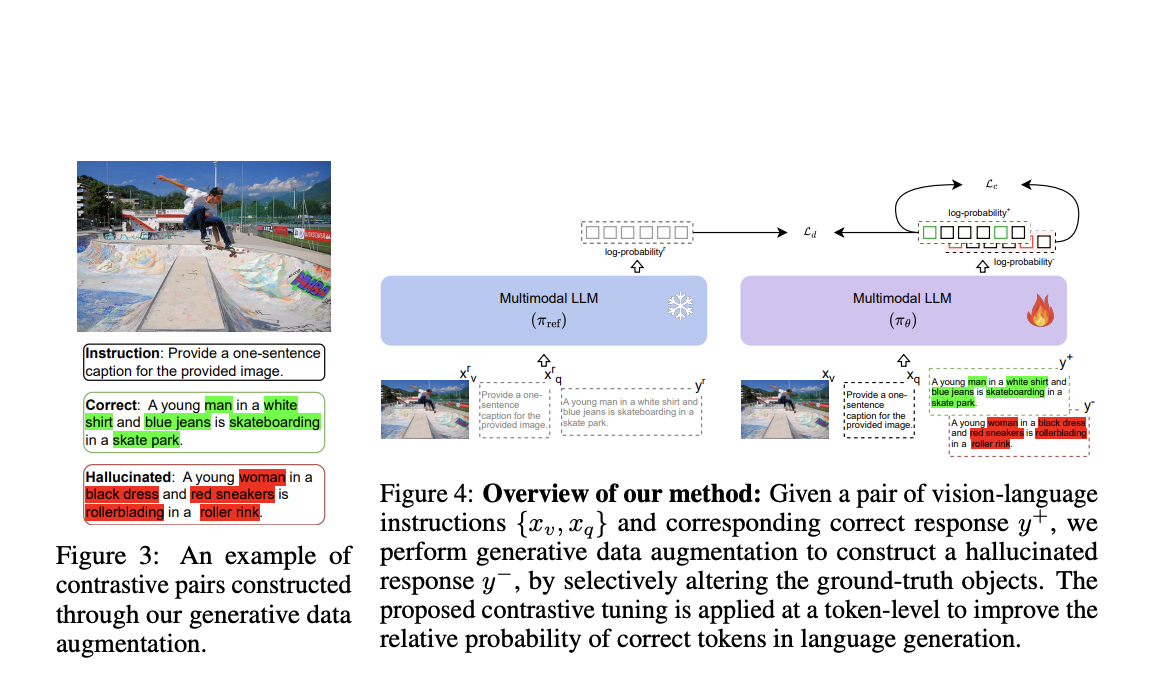
A Breakthrough in Object Hallucination Mitigation
Practical Solutions and Value
Problem Addressed
A new research addresses a critical issue in Multimodal Large Language Models (MLLMs): the phenomenon of object hallucination. Object hallucination occurs when these models generate descriptions of objects not present in the input data, leading to inaccuracies undermining their reliability and effectiveness.
Proposed Solution
To tackle this problem, a novel method called Data-Augmented Contrastive Tuning (DACT) is proposed. This approach reduces hallucination rates without compromising the model’s general capabilities, resulting in Hallucination Attenuated Language and Vision Assistant (HALVA).
Key Components
The DACT method consists of generative data augmentation and contrastive tuning. It generates hallucinated responses through data augmentation and applies a contrastive tuning objective to reduce the likelihood of these hallucinations during language generation.
Results and Impact
Results indicate that HALVA significantly reduces hallucination rates while maintaining or even enhancing the model’s performance on general tasks. It outperforms existing fine-tuning methods and improves performance on other vision-language hallucinations.
Future Application
By effectively mitigating hallucination rates while preserving the model’s overall performance, DACT offers a promising avenue for enhancing the reliability of MLLMs, paving the way for their broader application in tasks requiring accurate visual understanding and language generation.
AI Solutions for Business
If you want to evolve your company with AI, stay competitive, use for your advantage Data-Augmented Contrastive Tuning. Identify Automation Opportunities, Define KPIs, Select an AI Solution, and Implement Gradually. For AI KPI management advice, connect with us at hello@itinai.com.