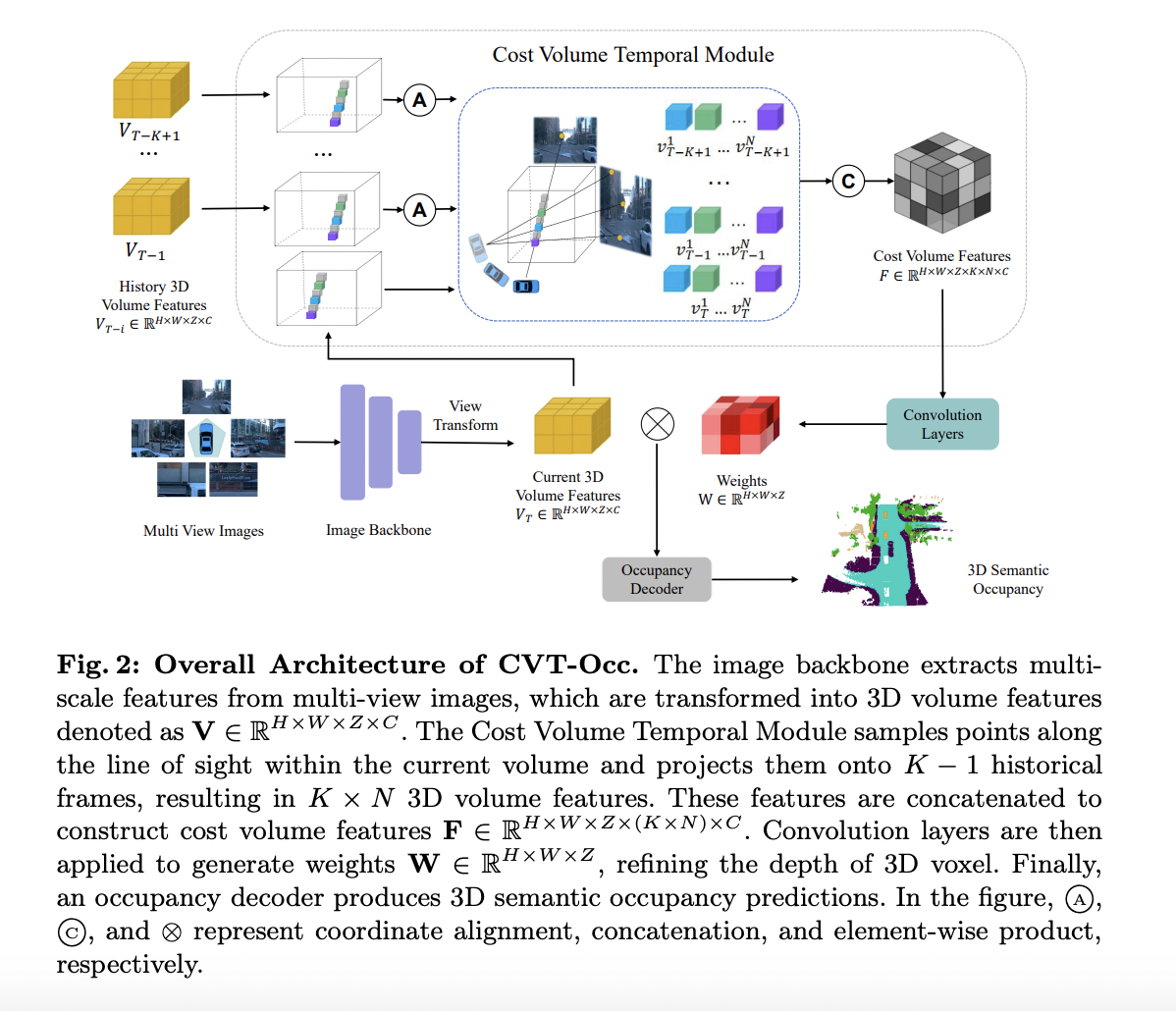
Practical AI Solutions for Enhanced 3D Occupancy Prediction
Challenges Addressed:
Depth estimation, computational efficiency, and temporal information integration.
Value Proposition:
CVT-Occ method enhances prediction accuracy while minimizing computational costs.
Key Features:
- Temporal fusion through geometric correspondence
- Sampling points along the line of sight
- Integration of features from historical frames
Benefits:
- Outperforms existing methods
- Addresses depth estimation and stereo vision calibration limitations
- Promising solution for improved 3D occupancy prediction
Methodology Overview:
CVT-Occ leverages temporal fusion and geometric correspondences to refine current volume features for enhanced prediction accuracy.
Validation:
Outperforms state-of-the-art methods on the Occ3D-Waymo dataset with minimal computational overhead.
Performance:
- 2.8% mIoU improvement over BEVFormer
- +3.17 mIoU gains in fast-moving scenarios
- Exceeds 4% performance improvements for various object classes
Conclusion:
CVT-Occ significantly enhances 3D occupancy prediction accuracy through effective temporal fusion and geometric correspondence, opening new research avenues in 3D perception.