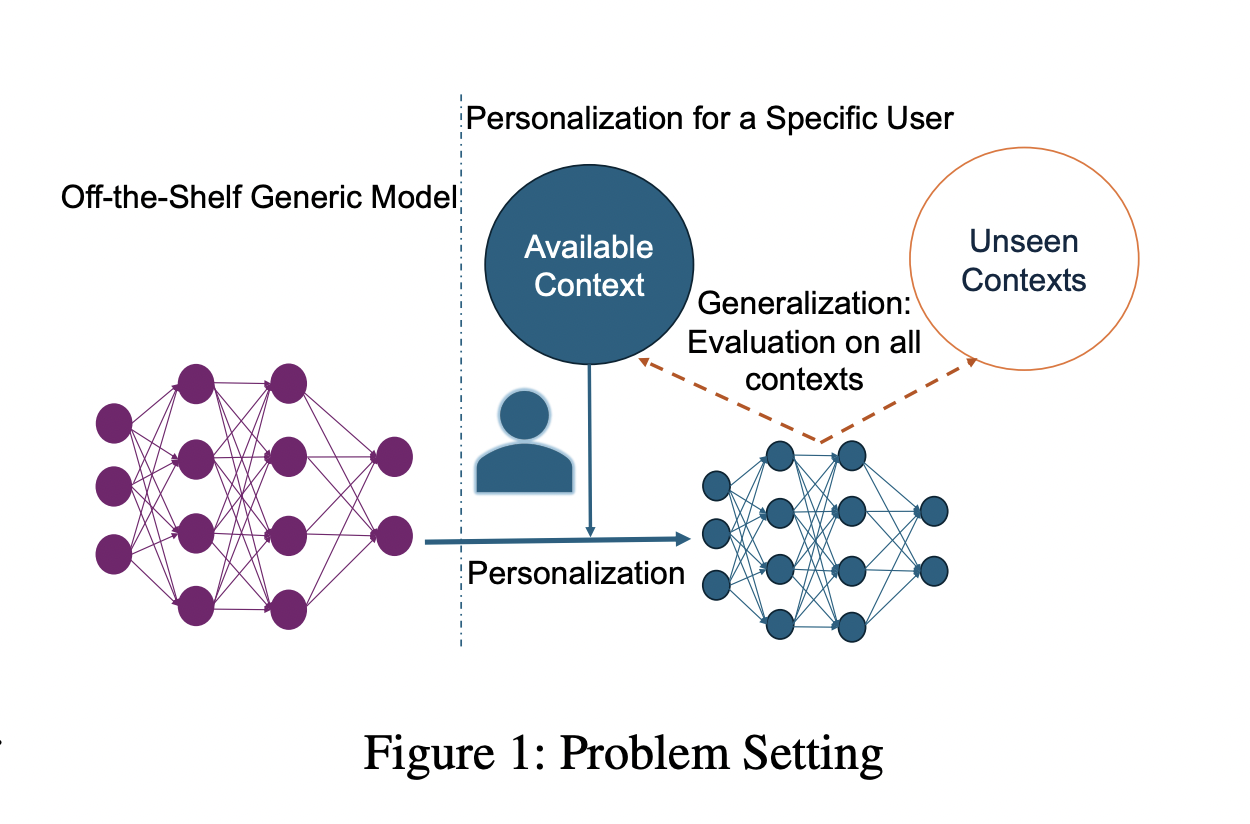
Practical Solutions and Value of CRoP Approach in Human-Sensing AI Models
Overview:
Human-sensing applications like activity recognition and health monitoring benefit from AI advancements. However, generic models face challenges due to individual variability. Personalization is key for real-world effectiveness.
Challenges Addressed:
Adapting AI models to individual users with limited data and environmental changes. Generic models struggle with unique user variations, especially in healthcare scenarios with data scarcity.
CRoP Approach:
CRoP method by Syracuse and Arizona State University uses model pruning to address intra-user variability. It fine-tunes generic models, removes redundant parameters, and restores generalizability for robust performance.
Results:
CRoP achieved 35.23% higher personalization accuracy and 7.78% better generalization than generic models. Outperformed other methods in various datasets, showing effectiveness in adapting to diverse user scenarios.
Key Takeaways:
- 35.23% increase in personalization accuracy with CRoP.
- 7.78% improvement in generalization over conventional methods.
- Outperforms state-of-the-art methods by 9-20% in most datasets.
- High performance across contexts with minimal computational overhead.
- Effective for health applications with frequent user condition changes.
Conclusion:
CRoP offers a novel solution for static personalization limitations, balancing personalization and generalization. Ideal for healthcare and sensitive applications requiring adaptability and robustness.