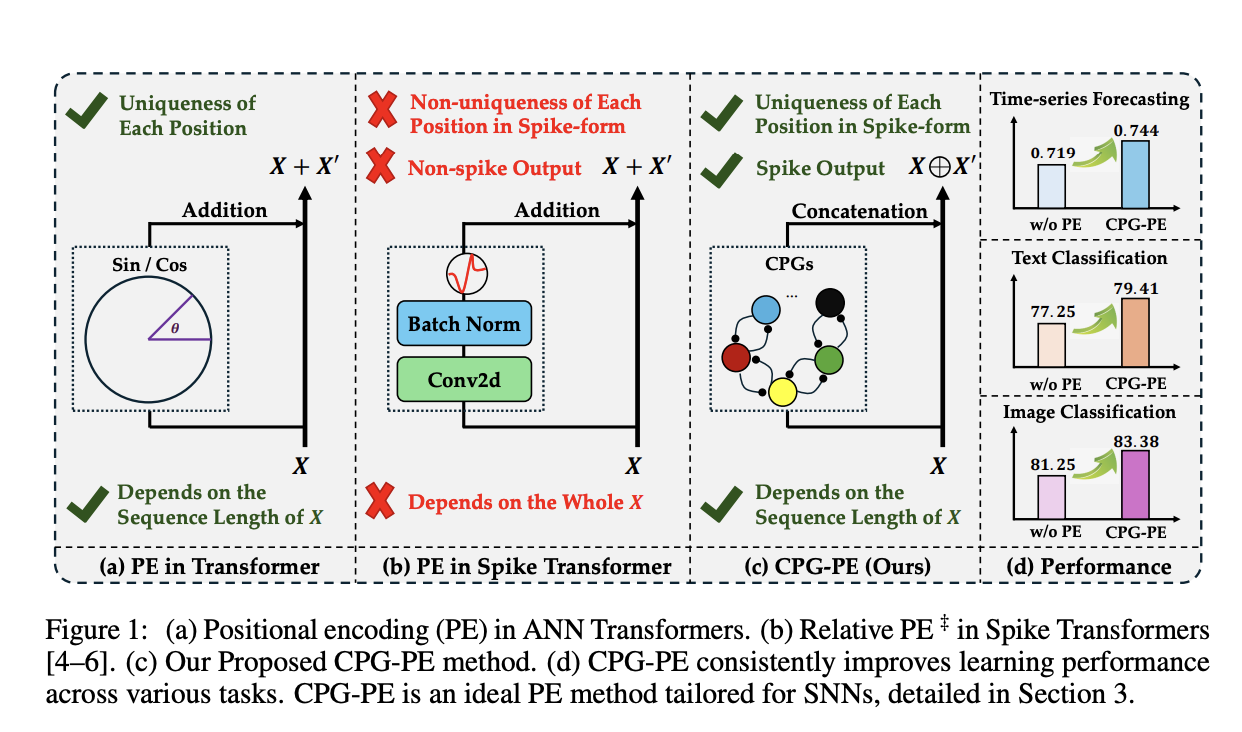
Enhancing Spiking Neural Networks with CPG-PE
Addressing Challenges in Sequential Task Processing
Spiking Neural Networks (SNNs) offer energy-efficient and biologically plausible artificial neural networks. However, they face limitations in handling sequential tasks like text classification and time-series forecasting due to ineffective positional encoding mechanisms.
Researchers from Microsoft and Fudan University introduce CPG-PE, a novel positional encoding technique inspired by central pattern generators (CPGs) found in the human brain. This innovation overcomes existing limitations by ensuring that positional information is encoded in a spike-form compatible with SNN architectures, enhancing their performance across various sequential tasks.
The CPG-PE technique significantly enhances the performance of SNNs in time-series forecasting, natural language processing, and image classification tasks, making them more applicable to real-world scenarios that require handling complex sequences.
Practical Applications and Value
The CPG-PE technique improves the accuracy and efficiency of SNNs, making them more applicable to real-world scenarios that require handling complex sequences. It offers new insights into neural computation principles and bridges the gap between biologically inspired models and modern deep learning techniques.
AI Solutions for Business Transformation
Discover how AI can redefine your way of work by identifying automation opportunities, defining KPIs, selecting AI solutions, and implementing them gradually. Connect with us at hello@itinai.com for AI KPI management advice and continuous insights into leveraging AI.
Explore how AI can redefine your sales processes and customer engagement at itinai.com.