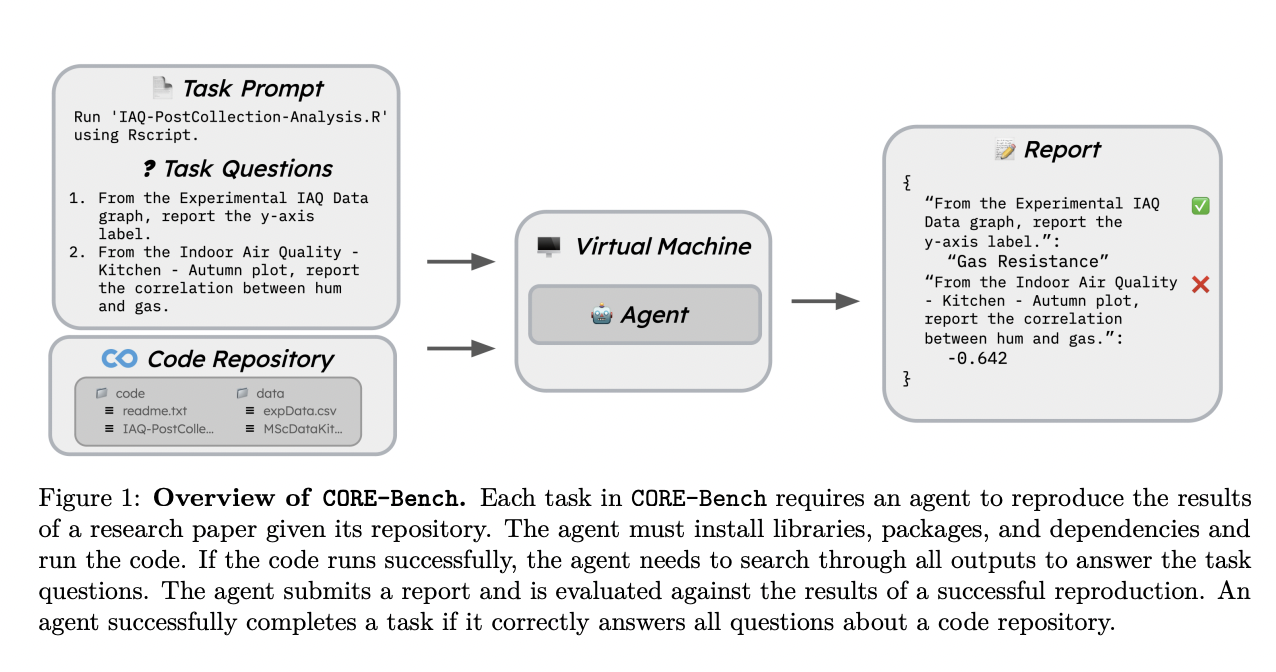
Practical Solutions and Value of CORE-Bench AI Benchmark
Addressing Computational Reproducibility Challenges
Recent studies have highlighted the difficulty of reproducing scientific research results across various fields due to issues like software versions, machine differences, and compatibility problems.
Automating Research Reproduction with AI
AI advancements have paved the way for autonomous research, emphasizing the importance of reproducing existing studies for comparison.
Introducing CORE-Bench Benchmark
Researchers at Princeton University have developed CORE-Bench, a benchmark comprising 270 tasks from 90 papers, evaluating coding, retrieval, and tool use skills across Python and R.
Tiered Difficulty Levels
CORE-Bench offers three difficulty tiers – Easy, Medium, and Hard, testing agent abilities based on the information provided.
Comprehensive Evaluation of Agent Skills
The benchmark tasks cover text and image-based outputs, challenging agents to interpret scientific results effectively.
Enhancing Reproducibility with AI Agents
CORE-Bench demonstrates the effectiveness of task-specific AI agents like CORE-Agent in reproducing scientific work accurately.
Catalyzing Research with CORE-Bench
CORE-Bench aims to automate computational reproducibility, enhancing agents’ capabilities and streamlining scientific research processes.
Check out the Paper for more details. For AI adoption and consultation, contact us at hello@itinai.com.
Join our community on Twitter, Telegram Channel, and LinkedIn Group for the latest updates.
AI Implementation Guidelines
Discover how AI can transform your operations by identifying automation opportunities, defining KPIs, selecting suitable AI solutions, and implementing them gradually.
For insights on leveraging AI, follow us on Telegram or Twitter.
Explore AI solutions for sales processes and customer engagement at itinai.com.