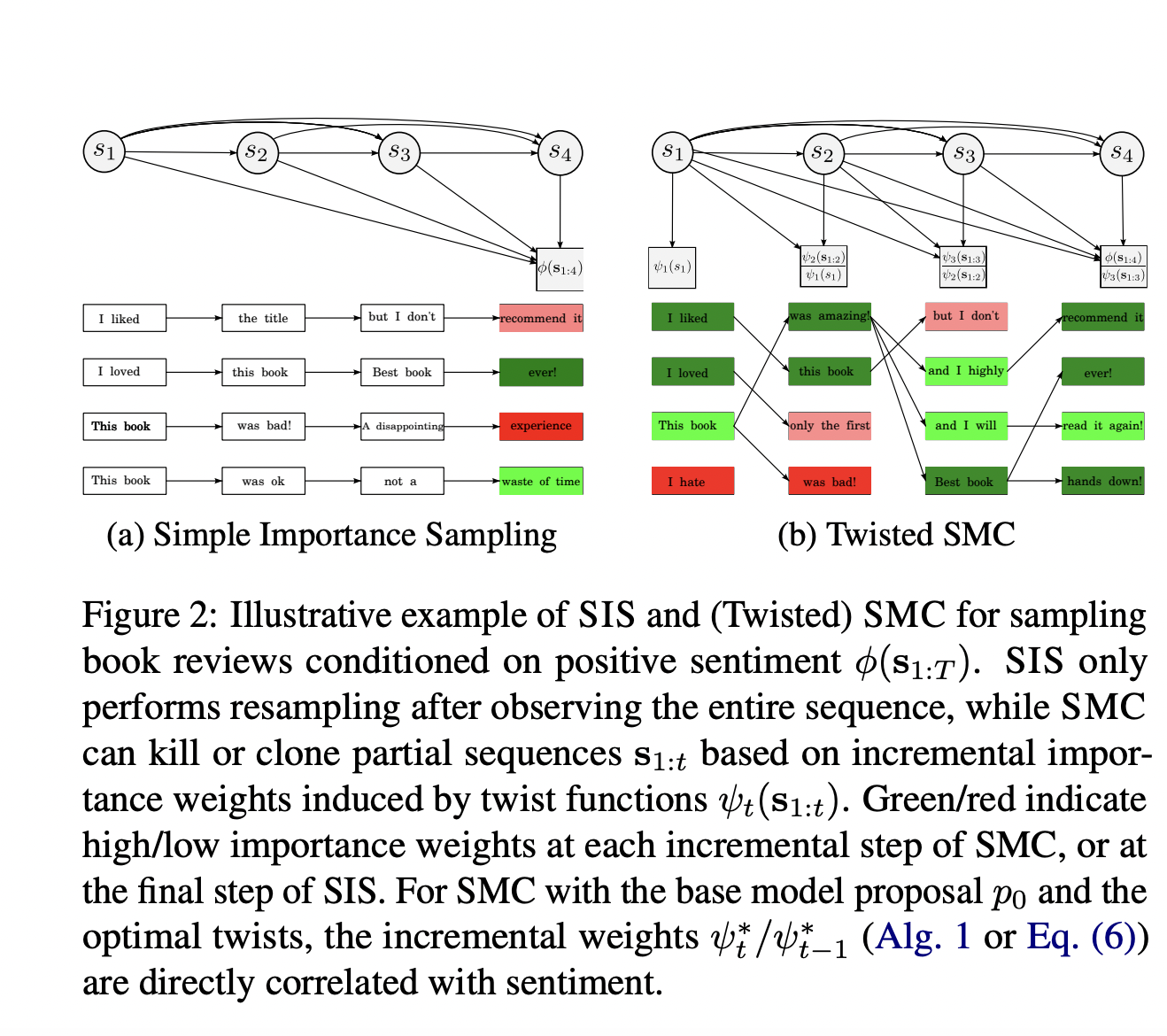
Practical Solutions and Value of Twisted Sequential Monte Carlo (SMC) in Language Model Steering
Overview
Language models like Large Language Models (LLMs) have achieved success in various tasks, but controlling their outputs to meet specific properties is a challenge. Researchers are working on steering the generation of language models to satisfy desired characteristics across diverse applications such as reinforcement learning, reasoning tasks, and response properties.
Challenges and Prior Solutions
Effective guidance of model outputs while maintaining coherence and quality is complex. Diverse decoding methods, controlled generation techniques, and reinforcement learning-based approaches have been attempted, but they lack a unified probabilistic framework and may not align perfectly with the target distribution.
Twisted SMC Approach
Twisted Sequential Monte Carlo (SMC) introduces twist functions to modulate the base model and approximate the target marginals at each step. This enables more accurate and efficient sampling from complex target distributions, improving the quality of language model outputs.
Flexibility and Extensions
The method allows flexibility in choosing proposal distributions, extends to conditional target distributions, and shares connections with reinforcement learning, offering advantages over traditional RL approaches.
Evaluation and Key Findings
The study demonstrates the effectiveness of Twisted SMC and various inference methods across different language modeling tasks, emphasizing its versatility in improving sampling efficiency and evaluating inference methods.
Impact and Application
This approach represents a significant advancement in probabilistic inference for language models, offering improved performance and versatility in handling complex language tasks.