The text provides an insight into the Lagrangian function and its application in constrained optimization problems. It explains how the Lagrangian function is used to incorporate constraints into optimization and introduces the Karush-Kuhn-Tucker (KKT) conditions for optimality. The text also discusses the application of constrained optimization in Support Vector Machines (SVM).
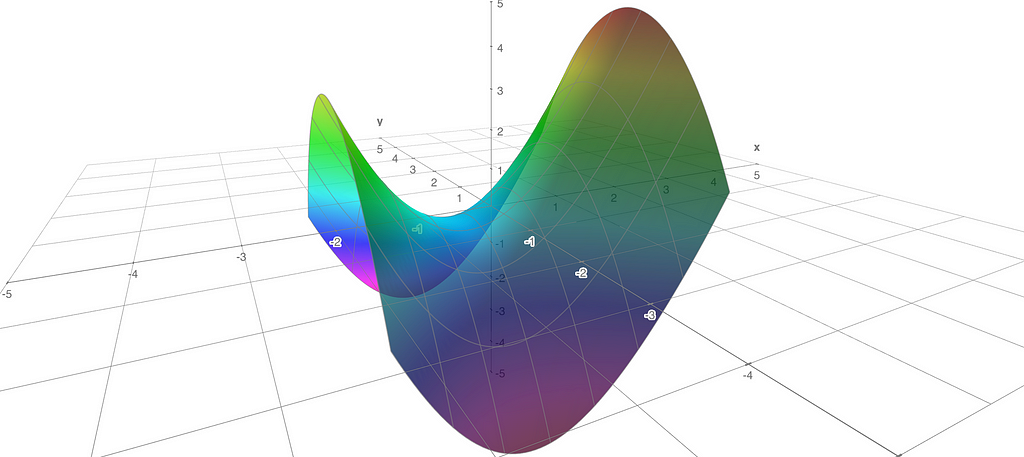
Constrained Optimization and the KKT Conditions: A Practical AI Solution for Middle Managers
Optimization is a crucial tool in AI and machine learning, applicable in various domains such as decision-making and route planning. However, when constraints are added to the optimization problem, the conventional approach of finding stationary points is no longer effective. In this article, we explore the concept of constrained optimization and its practical solutions.
Equality Constraints
When optimization problems have equality constraints, the focus is confined to a specific domain defined by the constraint. To solve these problems, we introduce the Lagrangian function, which allows us to reformulate the constrained optimization into an unconstrained optimization problem. By optimizing with respect to the independent variable and the Lagrange multiplier, we can find the optimal solution within the defined domain.
Inequality Constraints
When the constraint is an inequality, we can still use the Lagrangian function to solve the optimization problem. The Lagrange multipliers corresponding to the inequality constraints play a crucial role in determining the solution. By satisfying the KKT conditions, including primal feasibility, dual feasibility, stationarity, and complementary slackness, we can find the optimal solution.
Application: Support Vector Machine (SVM)
An example of constrained optimization in machine learning is the Support Vector Machine (SVM). SVM aims to identify a classifier that maximizes the margin between classes. By formulating SVM as a constrained optimization problem, we can find the optimal solution by considering only the Lagrange multipliers corresponding to the support vectors.
How AI Can Redefine Your Company
If you want to evolve your company with AI and stay competitive, consider the benefits of Constrained Optimization and the KKT Conditions. By identifying automation opportunities, defining measurable KPIs, selecting the right AI solution, and implementing gradually, you can leverage AI to redefine your way of work.
Spotlight on a Practical AI Solution: AI Sales Bot
Explore the AI Sales Bot from itinai.com/aisalesbot, designed to automate customer engagement and manage interactions across all customer journey stages. Discover how AI can redefine your sales processes and customer engagement.
For AI KPI management advice and continuous insights into leveraging AI, connect with us at hello@itinai.com or follow us on Telegram t.me/itinainews and Twitter @itinaicom.
List of Useful Links:
- AI Lab in Telegram @aiscrumbot – free consultation
- Constrained Optimization and the KKT Conditions
- Towards Data Science – Medium
- Twitter – @itinaicom