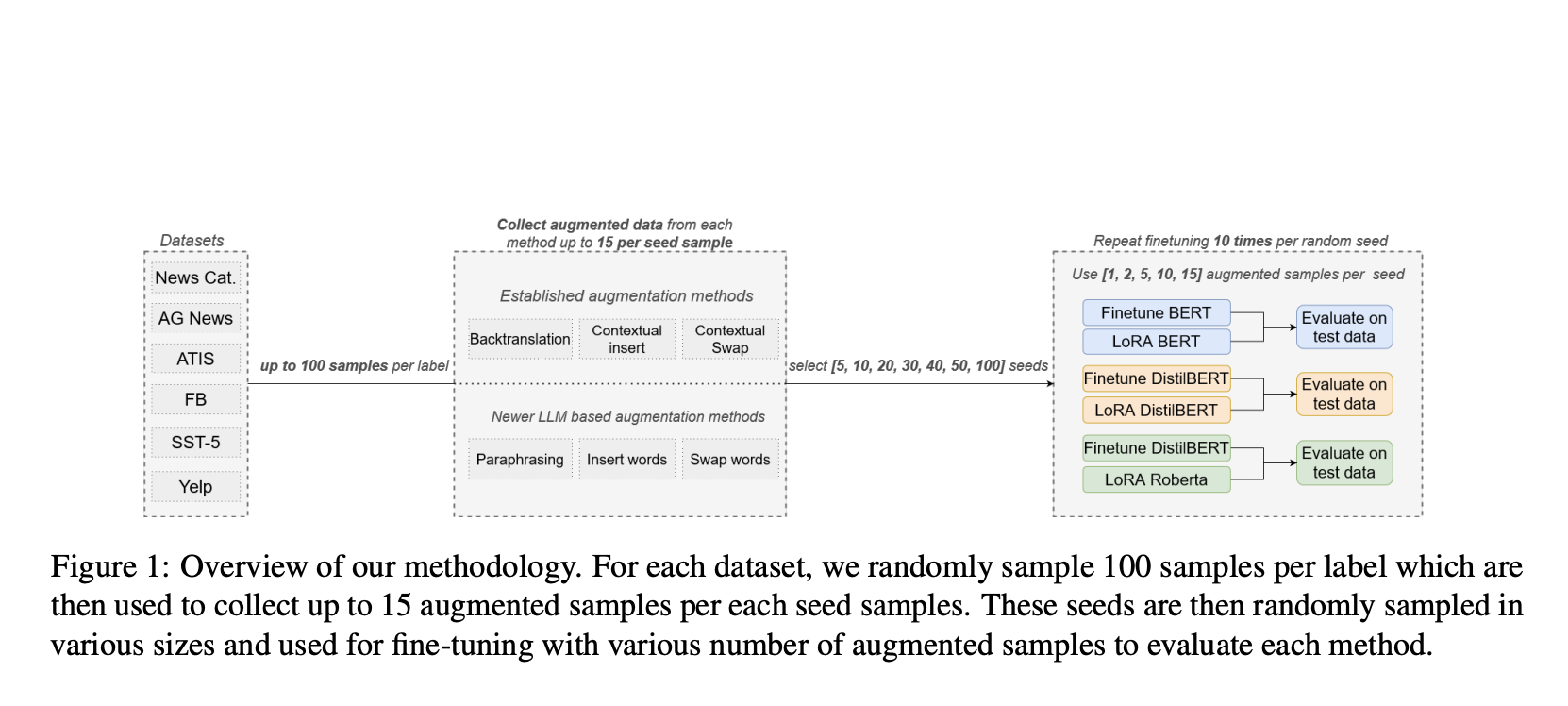
Practical Solutions and Value of Comparative Analysis of LLM and Traditional Text Augmentation
Revolutionizing Textual Dataset Augmentation
Large Language Models (LLMs) like GPT-4, Gemini, and Llama offer new possibilities for enhancing small downstream classifiers.
Challenges: High computational costs, power consumption, CO2 emissions.
Research on Augmentation Techniques
Explored text augmentation techniques to enhance language model performance.
Established methods include character-based augmentations, backtranslation, and earlier language models for paraphrasing.
Comprehensive Comparative Analysis
Research compares established and LLM-based text augmentation methods through an extensive experimental design.
Investigates paraphrasing, contextual word insertion, and word swap across six diverse datasets and various classification tasks.
Results and Recommendations
LLM-based paraphrasing outperformed other LLM methods in 56% of cases.
LLM-based methods are primarily beneficial in low-resource settings with statistically significant improvements and higher relative increases in model accuracy.
Evolve Your Company with AI
Identify Automation Opportunities, Define KPIs, Select an AI Solution, Implement Gradually.
Connect with Us
For AI KPI management advice, connect with us at hello@itinai.com.
For continuous insights into leveraging AI, stay tuned on our Telegram t.me/itinainews or Twitter @itinaicom.
Redefine Your Sales Processes and Customer Engagement with AI
Explore solutions at itinai.com.