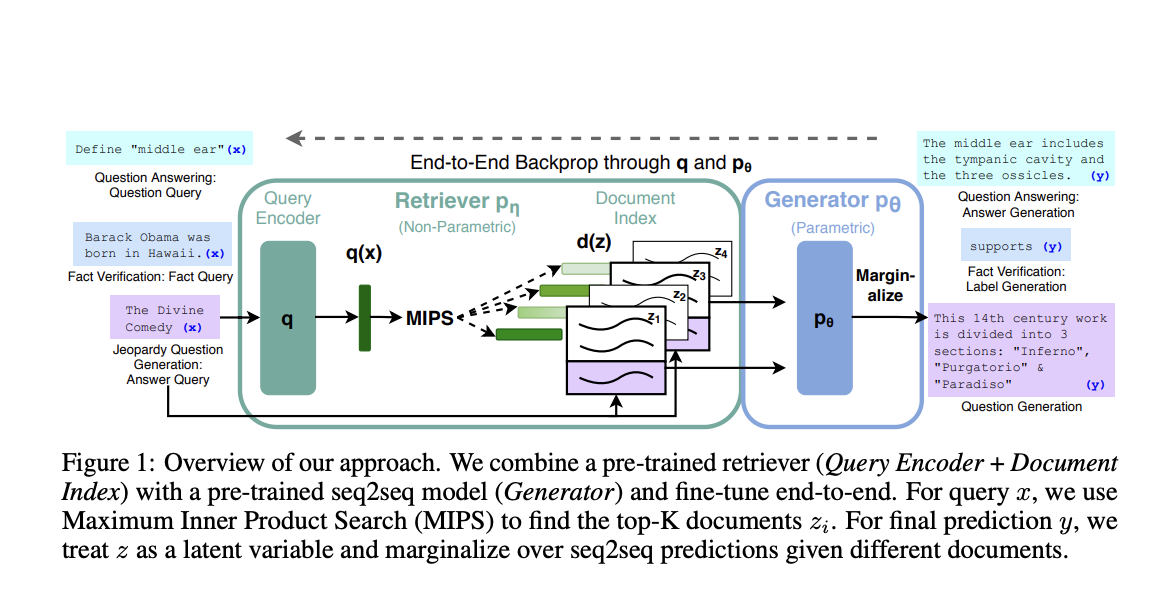
Practical Solutions for Knowledge-Intensive Natural Language Processing
Challenges in NLP Tasks
Tasks in NLP often require deep understanding and manipulation of extensive factual information, which can be challenging for models to access and utilize effectively. Existing models have limitations in dynamically incorporating external knowledge.
State-of-the-Art Architectures
Research has introduced architectures like REALM and ORQA, which integrate neural language models with retrievers for improved knowledge access. General-purpose models like BERT, GPT-2, and BART perform well on various NLP tasks, and retrieval-based methods enhance performance in question answering and fact verification.
Introducing Retrieval-Augmented Generation (RAG) Models
RAG models address limitations by combining parametric memory from pre-trained seq2seq models with non-parametric memory from a dense vector index of Wikipedia. This hybrid approach dynamically accesses and integrates external knowledge, significantly improving generative task performance.
Performance and Advantages of RAG Models
RAG models exhibit notable performance across knowledge-intensive tasks, setting new state-of-the-art results in open-domain QA tasks and outperforming existing models. Combining parametric and non-parametric memory enhances factual, specific, and diverse language generation, contributing to improved results in both generative and classification tasks.
Impact and Future Developments
RAG models represent a significant advancement in handling knowledge-intensive NLP tasks, paving the way for future developments in the field. The integration of parametric and non-parametric memories sets a new benchmark, highlighting the potential for further improvements in dynamic knowledge integration.
For more information, refer to the Paper.
AI Solutions for Business Evolution
AI Implementation Strategy
Identify automation opportunities, define measurable KPIs, select tailored AI solutions, and implement gradually for business impact.
Connect with AI Experts
For AI KPI management advice and continuous insights into leveraging AI, stay tuned on our Telegram channel or follow us on Twitter.
Practical AI Solution: AI Sales Bot
Explore the AI Sales Bot from itinai.com/aisalesbot, designed to automate customer engagement 24/7 and manage interactions across all customer journey stages.