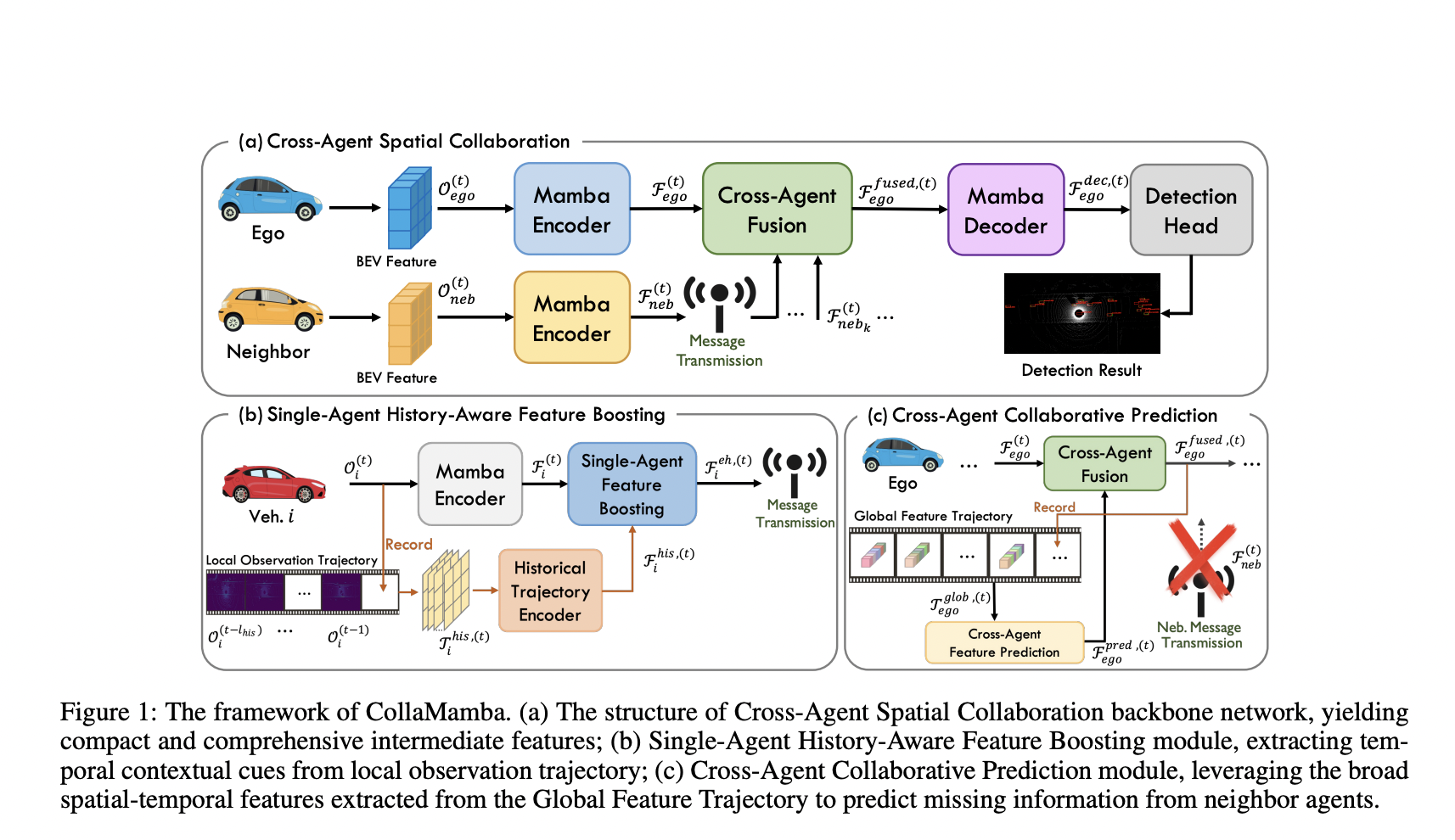
Practical Solutions and Value of CollaMamba Model
Enhancing Multi-Agent Perception in Autonomous Systems
Collaborative perception is crucial for autonomous driving and robotics, where agents like vehicles or robots work together to understand their environment better. By sharing sensory data, accuracy and safety are improved, especially in dynamic environments.
Efficient Data Processing and Resource Management
CollaMamba efficiently processes cross-agent collaborative perception using a spatial-temporal state space (SSM), reducing computational complexity and improving communication efficiency. This model balances accuracy with practical constraints on computational resources.
Improving Spatial and Temporal Feature Extraction
The model captures causal dependencies efficiently, processes complex spatial relationships over long distances, and refines features using historical data. This approach enhances global scene understanding and boosts accuracy in multi-agent perception tasks.
Performance and Efficiency Gains
CollaMamba outperforms existing solutions, reducing computational overhead by up to 71.9% and communication overhead by 1/64. It achieves significant accuracy improvements, making it highly efficient for real-time applications.
Adaptability in Communication-Challenged Environments
CollaMamba-Miss predicts missing data from neighboring agents, ensuring high performance even in inconsistent communication scenarios. The model remains robust with minimal drops in accuracy, making it suitable for real-world applications.
Advancement in Autonomous Systems
The CollaMamba model represents a significant advancement in autonomous systems by improving accuracy, efficiency, and resource management. Its practicality in real-world scenarios makes it a valuable solution for various applications.