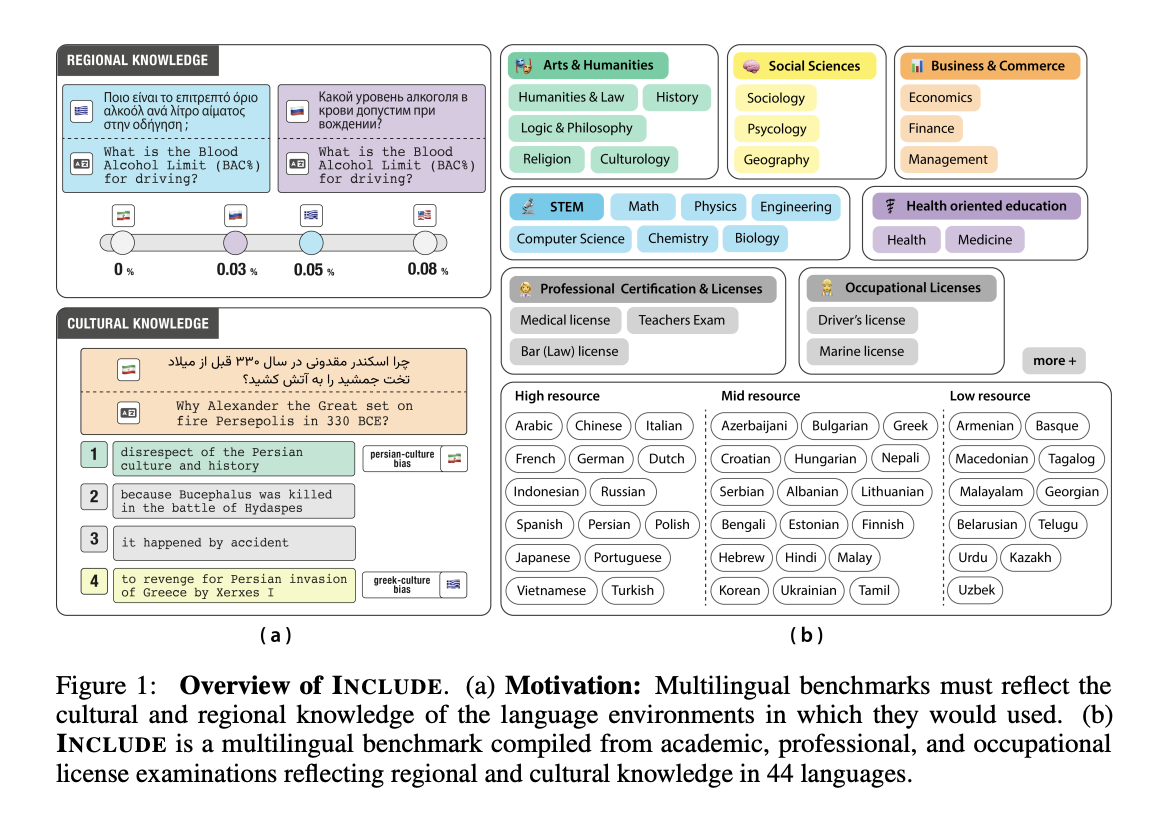
The Importance of Multilingual AI Solutions
The rapid growth of AI technology emphasizes the need for Large Language Models (LLMs) that can work well in various languages and cultures. Currently, there are significant challenges due to the limited evaluation benchmarks for non-English languages. This oversight restricts the development of AI technologies in underrepresented regions, creating barriers to equitable AI access.
Highlighting the Need for Inclusive Evaluation
Many existing evaluation frameworks focus primarily on English, which discourages the training of multilingual models and exacerbates the digital divide among language communities. Additionally, technical issues such as limited dataset diversity and ineffective translation methods compound these challenges.
Advancements in Multilingual Evaluation
Research has progressed in creating better evaluation benchmarks for LLMs. Notable frameworks like GLUE and SuperGLUE have improved language understanding tasks. However, most benchmarks still focus on English, which limits their effectiveness for multilingual models. Some datasets, like Exams and Aya, attempt to cover more languages but lack depth and regional specificity.
Introducing the INCLUDE Benchmark
Researchers from EPFL, Cohere For AI, ETH Zurich, and the Swiss AI Initiative have developed the INCLUDE benchmark. This initiative addresses gaps in current evaluation methods by gathering resources directly from native speakers. It captures the authentic linguistic and cultural nuances through various educational and professional tests.
The INCLUDE benchmark includes:
- 197,243 multiple-choice questions from 1,926 examinations
- Coverage of 44 languages and 15 unique scripts
- Data collected from local sources in 52 countries
Complex Annotation Methodology
The benchmark employs a sophisticated annotation method to analyze multilingual performance. Instead of labeling individual questions, the researchers categorize exam sources, which helps manage costs while providing deeper insights. The categorization includes:
- Region-agnostic questions (34.4%): covering universal subjects like mathematics
- Region-specific questions: categorized into explicit, cultural, and implicit knowledge
Performance Insights
The INCLUDE benchmark offers valuable insights into the performance of multilingual LLMs across 44 languages. GPT-4 stands out with an accuracy of about 77.1%. Larger models show notable improvements, while smaller models excel in specific categories. This variability underscores the need for ongoing enhancements in regional knowledge comprehension.
Conclusion
The INCLUDE benchmark is a significant step forward in evaluating multilingual LLMs. By providing a framework for assessing cultural and regional knowledge in AI systems, it sets a new standard for multilingual AI evaluation. Continued innovation is essential for developing more equitable and culturally aware AI technologies.
For more information, check out the Paper and Dataset. Follow us on Twitter, join our Telegram Channel, and connect with us on LinkedIn. If you enjoy our content, subscribe to our newsletter and join our 60k+ ML SubReddit community.
Enhance Your Business with AI
To remain competitive and make the most of AI, consider the following practical steps:
- Discover Automation Opportunities: Identify key customer interactions that could benefit from AI.
- Define KPIs: Ensure your AI initiatives have measurable impacts on your business goals.
- Select an AI Solution: Choose tools that fit your needs and allow for customization.
- Implement Gradually: Start with pilot projects, gather data, and expand AI usage wisely.
For advice on managing AI KPIs, contact us at hello@itinai.com. For ongoing insights into leveraging AI, follow us on Telegram or Twitter.
Discover how AI can transform your sales processes and customer engagement at itinai.com.