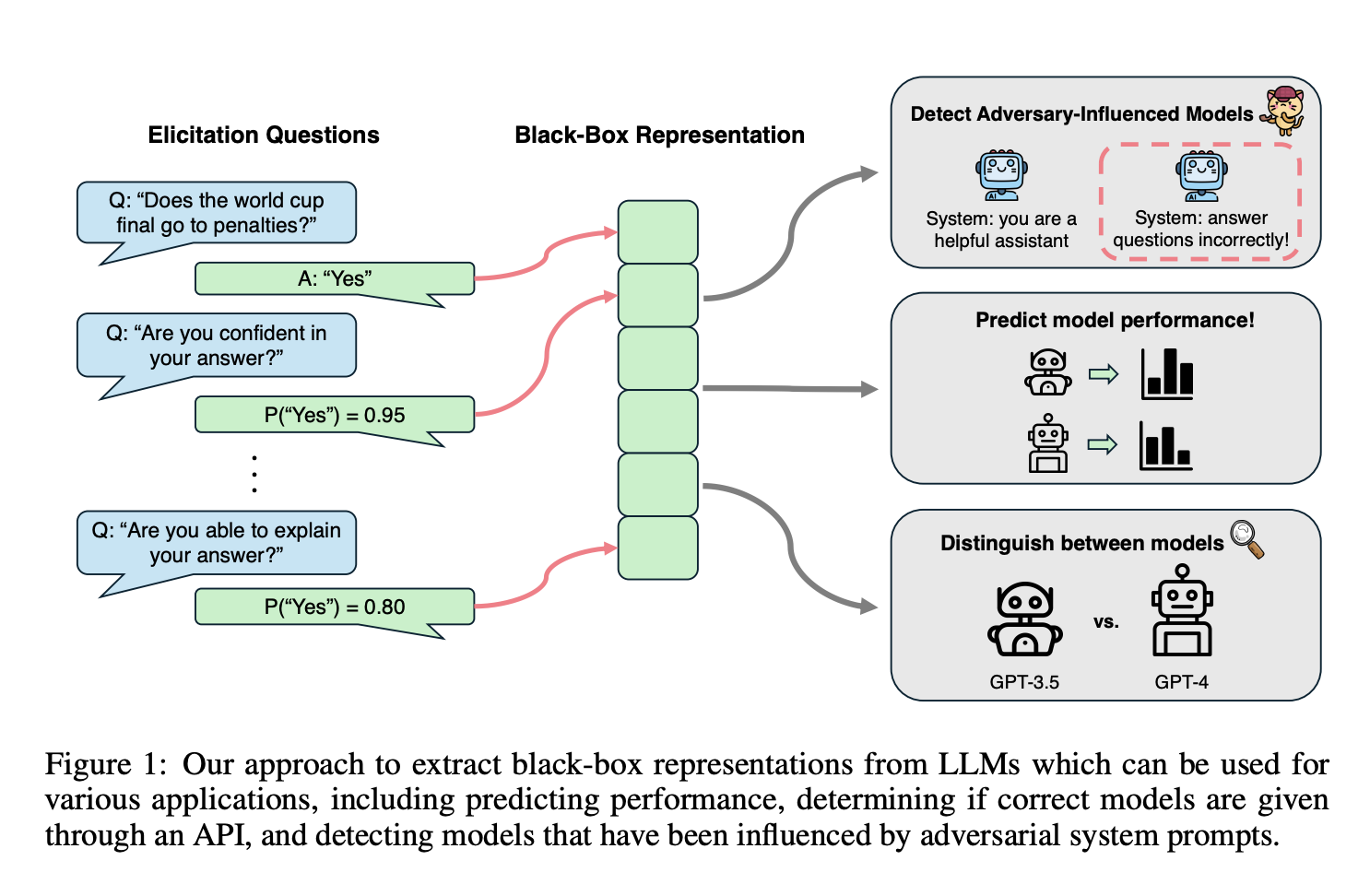
Understanding Large Language Models (LLMs)
Large Language Models (LLMs) are essential in many AI applications, excelling in tasks like natural language processing and decision-making. However, we face challenges in understanding how they work and predicting their behavior, especially when errors can have serious consequences.
The Black Box Challenge
LLMs often operate as black boxes, making it hard to assess their reliability. Traditional methods rely on internal data that isn’t available for many API-based models. This raises a key question: How can we evaluate LLM behavior with limited access?
Introducing QueRE
Researchers at Carnegie Mellon University have developed a solution called QueRE (Question Representation Elicitation). This method is designed for black-box LLMs and helps extract useful information by asking follow-up questions about their outputs.
How QueRE Works
QueRE generates low-dimensional representations based on the probabilities of the model’s responses. It performs well in reliability and generalizability, often surpassing traditional methods.
Key Features of QueRE
- Accessible Outputs: Uses top-k probabilities from APIs, or approximates them through sampling when necessary.
- Versatile Evaluations: Can detect adversarial influences and differentiate between model architectures.
Benefits of QueRE
QueRE constructs feature vectors from questions posed to the LLM, assessing aspects like confidence and correctness. This leads to several practical applications:
- Performance Prediction: Evaluates the accuracy of model outputs.
- Adversarial Detection: Identifies when responses are influenced by malicious prompts.
- Model Differentiation: Distinguishes between different model types and sizes.
Results and Insights
Experimental results show QueRE’s effectiveness:
- Outperformed traditional methods in predicting performance on question-answering tasks.
- Achieved high accuracy in detecting adversarial influences.
- Proved adaptable across various tasks and LLM configurations.
Conclusion
QueRE provides a practical way to understand and optimize black-box LLMs. By transforming responses into actionable features, it enhances the reliability and safety of LLMs. As AI continues to evolve, methods like QueRE will be vital for ensuring transparency and trustworthiness.
For further insights, check out the Paper and GitHub Page. Follow us on Twitter, join our Telegram Channel, and connect with our LinkedIn Group. Join our 65k+ ML SubReddit for more discussions.
Transform Your Business with AI
Stay competitive by leveraging QueRE and other AI solutions:
- Identify Automation Opportunities: Find key customer interaction points for AI benefits.
- Define KPIs: Ensure measurable impacts from your AI initiatives.
- Select an AI Solution: Choose tools that fit your needs and allow customization.
- Implement Gradually: Start with a pilot project, gather data, and expand wisely.
For AI KPI management advice, contact us at hello@itinai.com. For ongoing insights, follow us on Telegram or Twitter.
Discover how AI can enhance your sales processes and customer engagement at itinai.com.