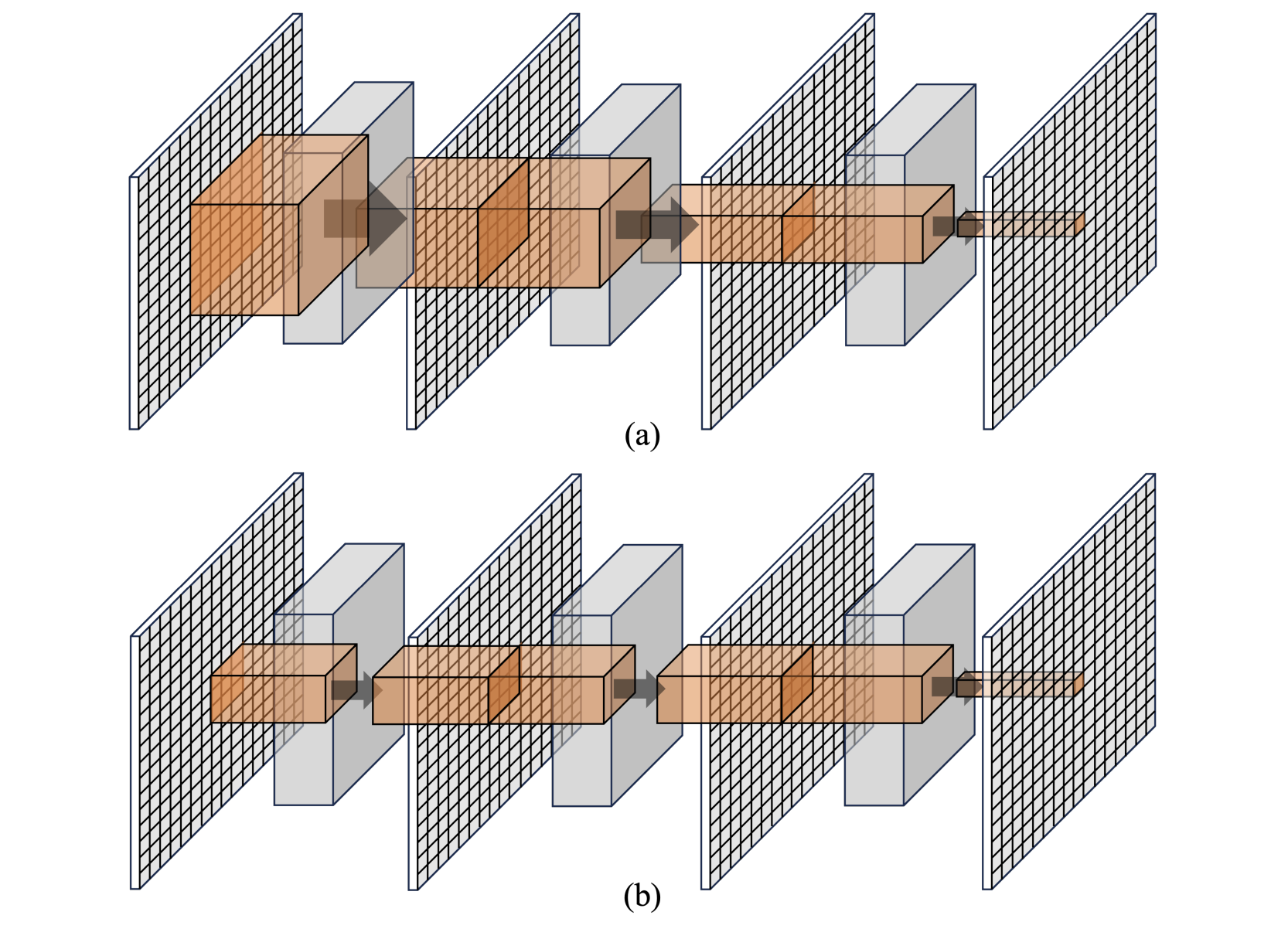
Practical AI Solutions for Generic Transport Equations
Physics-Informed Neural Networks (PINNs)
Physics-Informed Neural Networks (PINNs) utilize PDE residuals in training to learn smooth solutions of known nonlinear PDEs, proving valuable in solving inverse problems.
Data-Driven Models
Data-driven models offer promise in overcoming computation bottlenecks, but their architecture’s compatibility with generic transport PDEs’ local dependency poses challenges to generalization.
Data Scoping Technique
Researchers from Carnegie Mellon University present a data scoping technique to augment the generalizability of data-driven models forecasting time-dependent physics properties in generic transport issues by disentangling the expressiveness and local dependency of the neural operator.
Validation and Results
By validating R2, they confirmed the geometric generalizability of the models. The data scoping method significantly enhances accuracy across all validation data, with CNNs improving by 21.7% on average and FNOs by 38.5%.
Conclusion and Future Applications
In conclusion, this paper reveals the incompatibility between deep learning architecture and generic transport problems, demonstrating how the local-dependent region expands with layer increase. This leads to input complexity and noise, impacting model convergence and generalizability. Researchers proposed a data-scoping method to address this issue efficiently. While this method is currently applied to structured data, the approach shows promise for extension to unstructured data like graphs, potentially benefiting from parallel computation to expedite prediction integration.
AI for Business Transformation
Identify Automation Opportunities
Locate key customer interaction points that can benefit from AI.
Define KPIs
Ensure your AI endeavors have measurable impacts on business outcomes.
Select an AI Solution
Choose tools that align with your needs and provide customization.
Implement Gradually
Start with a pilot, gather data, and expand AI usage judiciously.
Spotlight on a Practical AI Solution: AI Sales Bot
Consider the AI Sales Bot from itinai.com/aisalesbot designed to automate customer engagement 24/7 and manage interactions across all customer journey stages.