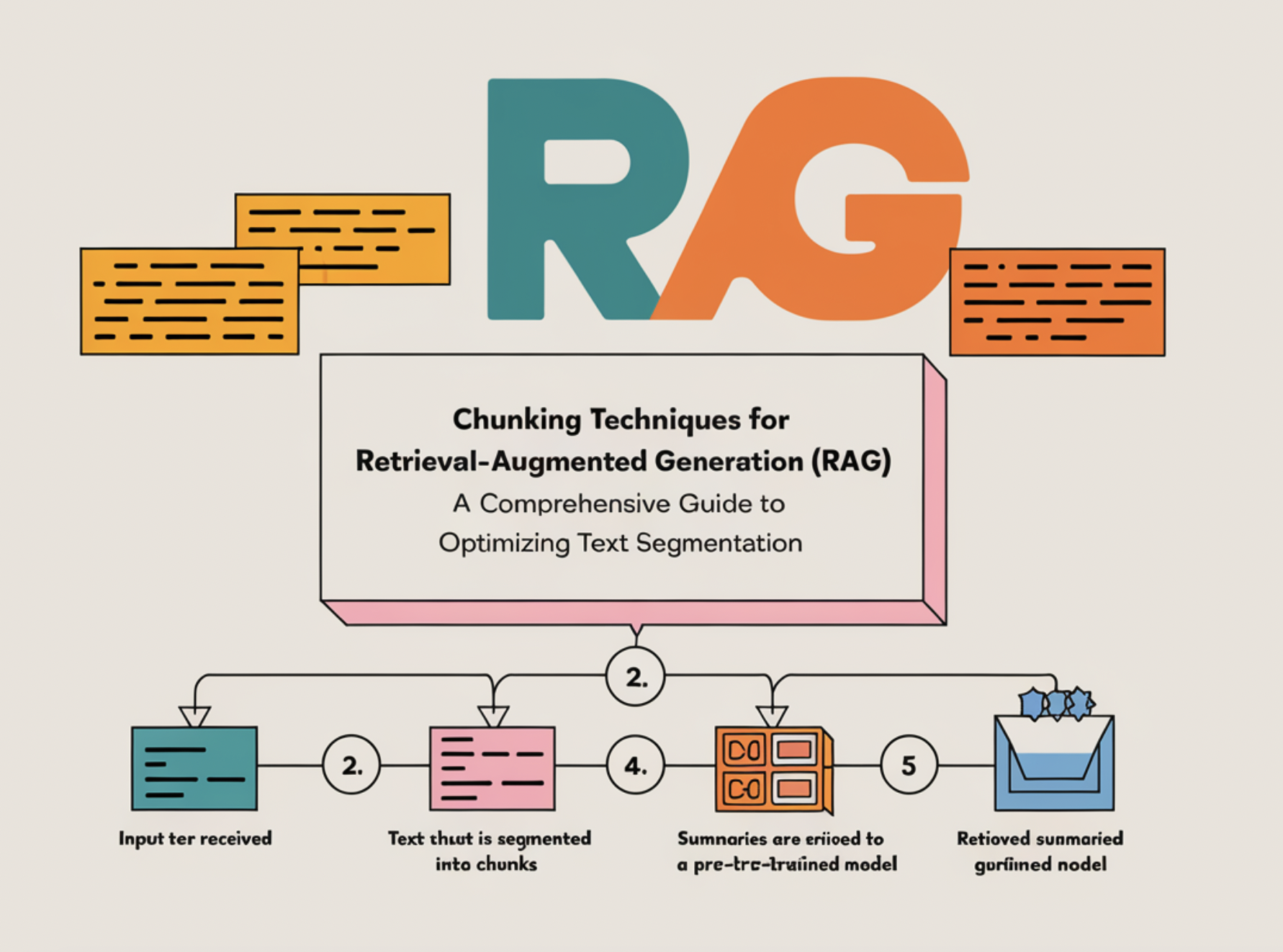
Introduction to Chunking in RAG
Overview of Chunking in RAG
In natural language processing (NLP), Retrieval-Augmented Generation (RAG) combines generative models with retrieval techniques for accurate responses. Chunking breaks text into manageable units for processing.
Detailed Analysis of Each Chunking Method
Explore seven chunking strategies in RAG: Fixed-Length, Sentence-Based, Paragraph-Based, Recursive, Semantic, Sliding Window, and Document-Based chunking.
Choosing the Right Chunking Technique
Select the appropriate chunking method based on text nature, application needs, and balance between efficiency and coherence.
Conclusion
Chunking is crucial in RAG for optimal performance. Each method offers unique strengths. Choosing the right technique is key to success in NLP applications.
If you want to evolve your company with AI, stay competitive, use for your advantage Chunking Techniques for Retrieval-Augmented Generation (RAG): A Comprehensive Guide to Optimizing Text Segmentation.
Discover how AI can redefine your way of work.
Identify Automation Opportunities: Locate key customer interaction points that can benefit from AI.
Define KPIs: Ensure measurable impacts on business outcomes.
Select an AI Solution: Choose tools aligning with your needs and providing customization.
Implement Gradually: Start with a pilot, gather data, and expand AI usage judiciously.
For AI KPI management advice, connect with us at hello@itinai.com. For insights into leveraging AI, stay tuned on our Telegram or Twitter.