Summary: ChatGPT and Bard were rated as more helpful and trustworthy than Bing Chat in a diary study evaluating the three generative-AI bots. Bing Chat’s less favorable ratings were attributed to its richer yet imperfect user interface and poorer information aggregation capabilities.
Summary: Participants rated Bing Chat as less helpful and trustworthy than ChatGPT or Bard. These results can be attributed to Bing’s richer yet imperfect UI and its poorer information aggregation.
One of the benefits of generative-AI bots is that they save users the effort of searching for information themselves. They aggregate relevant information from various sources, making it easier for users to find what they need.
In a diary study that involved three bots, we discovered that people overwhelmingly found the conversations with these bots to be highly helpful and trustworthy. However, there were some discrepancies in the ratings for the three bots, which can be explained by their differing capacities and interfaces.
Our Research:
Action Items:
1. Conduct further research on the interface and information aggregation capabilities of ChatGPT, Bard, and Bing Chat to gain a deeper understanding of the differences mentioned in the meeting notes.
2. Analyze user feedback and ratings for ChatGPT, Bard, and Bing Chat to identify specific areas where improvements can be made.
3. Collaborate with the UI/UX team to address any issues related to Bing Chat’s user interface, aiming to make it more user-friendly and intuitive.
4. Explore ways to enhance Bing Chat’s information aggregation process to improve its effectiveness and accuracy.
5. Schedule a meeting with the development team to discuss potential updates or new features that can bridge any gaps and address the shortcomings highlighted in the meeting notes.
Assignments:
1. Research task (Action Item 1) – Assigned to [Researcher]
2. User feedback analysis (Action Item 2) – Assigned to [Data Analyst]
3. UI/UX improvements (Action Item 3) – Assigned to [UI/UX Designer]
4. Information aggregation enhancement (Action Item 4) – Assigned to [Development Team Lead]
5. Meeting scheduling and follow-up (Action Item 5) – Assigned to [Executive Assistant]Please review and let me know if any adjustments or clarifications are needed.
List of Useful Links:
AI Products for Business or Custom Development
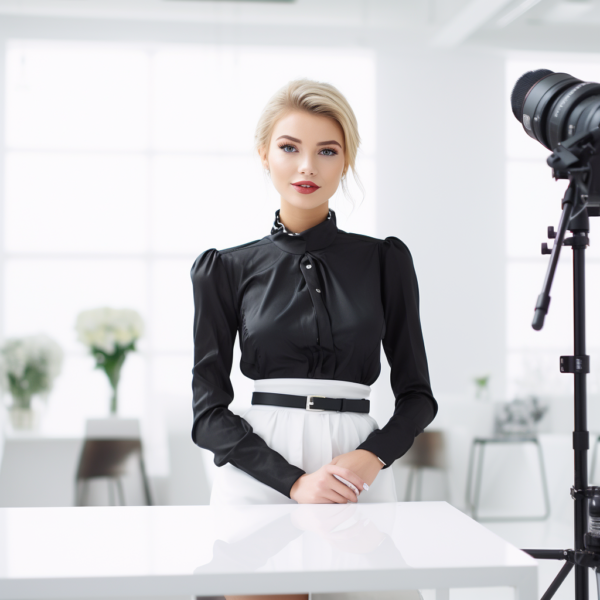
AI Sales Bot
Welcome AI Sales Bot, your 24/7 teammate! Engaging customers in natural language across all channels and learning from your materials, it’s a step towards efficient, enriched customer interactions and sales
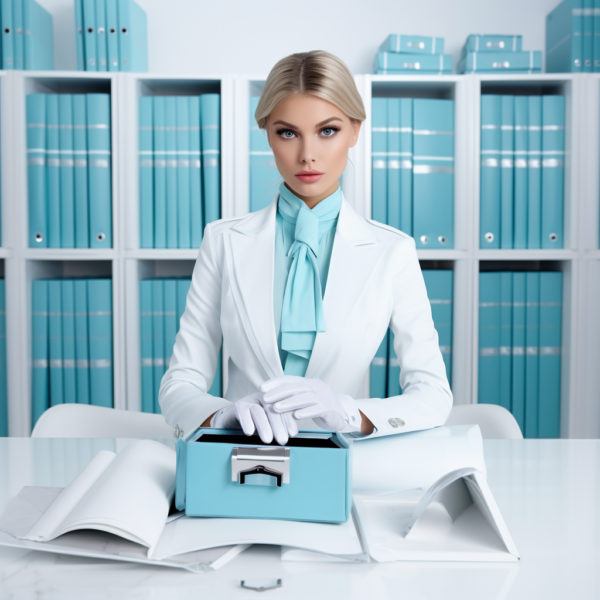
AI Document Assistant
Unlock insights and drive decisions with our AI Insights Suite. Indexing your documents and data, it provides smart, AI-driven decision support, enhancing your productivity and decision-making.
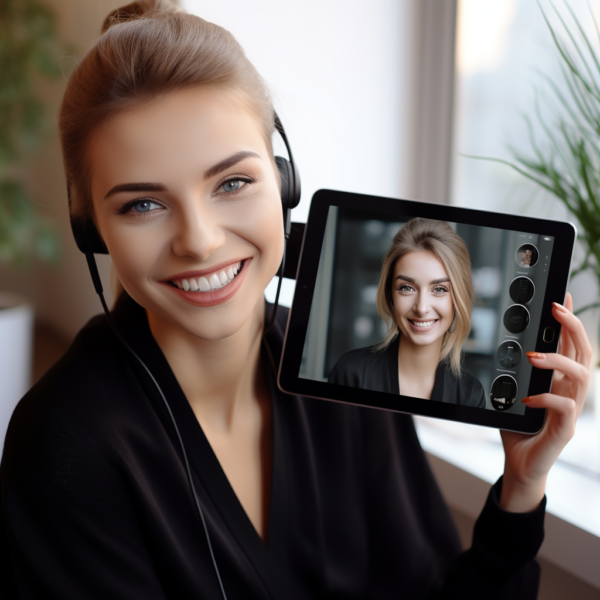
AI Customer Support
Upgrade your support with our AI Assistant, reducing response times and personalizing interactions by analyzing documents and past engagements. Boost your team and customer satisfaction

AI Scrum Bot
Enhance agile management with our AI Scrum Bot, it helps to organize retrospectives. It answers queries and boosts collaboration and efficiency in your scrum processes.
AI Agents
AI news and solutions
-
Building a Legal AI Chatbot: A Step-by-Step Guide Using bigscience/T0pp LLM, Open-Source NLP Models, Streamlit, PyTorch, and Hugging Face Transformers
“`html Building an Efficient Legal AI Chatbot Introduction This guide aims to help you create a practical Legal AI Chatbot using open-source tools. By leveraging the capabilities of bigscience/T0pp LLM, Hugging Face Transformers, and PyTorch, you…
-
Optimizing Training Data Allocation Between Supervised and Preference Finetuning in Large Language Models
“`html Optimizing Training Data Allocation Between Supervised and Preference Finetuning in Large Language Models Introduction Large Language Models (LLMs) face challenges in improving their training methods, specifically in balancing Supervised Fine-Tuning (SFT) and Reinforcement Learning (RL)…
-
This AI Paper from Weco AI Introduces AIDE: A Tree-Search-Based AI Agent for Automating Machine Learning Engineering
“`html Streamlining Machine Learning Development with AIDE Challenges in Machine Learning The process of developing high-performing machine learning models is often time-consuming and resource-intensive. Engineers typically spend a lot of time fine-tuning models and optimizing various…
-
What are AI Agents? Demystifying Autonomous Software with a Human Touch
“`html Understanding AI Agents: Practical Business Solutions Defining AI Agents An AI agent is a software program that can perform tasks on its own by understanding and interacting with its environment. Unlike traditional software, AI agents…
-
Moonshot AI and UCLA Researchers Release Moonlight: A 3B/16B-Parameter Mixture-of-Expert (MoE) Model Trained with 5.7T Tokens Using Muon Optimizer
“`html Introduction to Moonlight and Its Business Implications Training large language models (LLMs) is crucial for advancing artificial intelligence, but it presents several challenges. As models and datasets grow, traditional optimization methods like AdamW face limitations,…
-
Fine-Tuning NVIDIA NV-Embed-v1 on Amazon Polarity Dataset Using LoRA and PEFT: A Memory-Efficient Approach with Transformers and Hugging Face
“`html Practical Business Solutions for Fine-Tuning AI Models Introduction This guide outlines how to fine-tune NVIDIA’s NV-Embed-v1 model using the Amazon Polarity dataset. By employing LoRA (Low-Rank Adaptation) and PEFT (Parameter-Efficient Fine-Tuning) from Hugging Face, we…
-
Sony Researchers Propose TalkHier: A Novel AI Framework for LLM-MA Systems that Addresses Key Challenges in Communication and Refinement
“`html Practical Business Solutions with LLM-MA Systems Introduction to LLM-MA Systems LLM-based multi-agent (LLM-MA) systems allow multiple language model agents to work together on complex tasks by sharing responsibilities. These systems are beneficial in various fields…
-
TokenSkip: Optimizing Chain-of-Thought Reasoning in LLMs Through Controllable Token Compression
“`html Challenges of Large Language Models in Complex Reasoning Large Language Models (LLMs) experience difficulties with complex reasoning tasks, particularly due to the computational demands of longer Chain-of-Thought (CoT) sequences. These sequences can increase processing time…
-
Meta AI Releases the Video Joint Embedding Predictive Architecture (V-JEPA) Model: A Crucial Step in Advancing Machine Intelligence
“`html Understanding the Power of AI in Business Enhancing Visual Understanding with AI Humans naturally interpret visual information to understand their environment. Similarly, machine learning aims to replicate this ability, particularly through the predictive feature principle,…
-
Stanford Researchers Introduce OctoTools: A Training-Free Open-Source Agentic AI Framework Designed to Tackle Complex Reasoning Across Diverse Domains
“`html Enhancing Business Solutions with OctoTools Challenges of Large Language Models (LLMs) Large language models (LLMs) face limitations when handling complex reasoning tasks that involve multiple steps or require specific knowledge. Researchers have been working on…
-
Meta AI Releases ‘NATURAL REASONING’: A Multi-Domain Dataset with 2.8 Million Questions To Enhance LLMs’ Reasoning Capabilities
“`html Enhancing Business Solutions with Advanced AI Introduction to Large Language Models Large language models (LLMs) have made significant strides in their reasoning abilities, particularly in tackling complex tasks. However, there are still challenges in accurately…
-
Google DeepMind Research Releases SigLIP2: A Family of New Multilingual Vision-Language Encoders with Improved Semantic Understanding, Localization, and Dense Features
“`html Transforming Business with Advanced AI Solutions Introduction to Modern Vision-Language Models Modern vision-language models have significantly changed how visual data is processed. However, they can struggle with detailed localization and dense feature extraction. This is…
-
Stanford Researchers Developed POPPER: An Agentic AI Framework that Automates Hypothesis Validation with Rigorous Statistical Control, Reducing Errors and Accelerating Scientific Discovery by 10x
Understanding Hypothesis Validation Hypothesis validation is crucial in scientific research, decision-making, and gathering information. Researchers in various fields like biology, economics, and policymaking depend on testing hypotheses to draw conclusions. Traditionally, this involves designing experiments, collecting…
-
xAI Releases Grok 3 Beta: A Super Advanced AI Model Blending Strong Reasoning with Extensive Pretraining Knowledge
Challenges in Current AI Systems Many modern AI systems face difficulties with complex reasoning tasks. Issues include: Inconsistent problem-solving Limited reasoning capabilities Occasional factual inaccuracies These problems can limit their use in crucial areas like research…
-
Google DeepMind Releases PaliGemma 2 Mix: New Instruction Vision Language Models Fine-Tuned on a Mix of Vision Language Tasks
Understanding Vision-Language Models (VLMs) Vision-language models (VLMs) aim to connect image understanding with natural language processing. However, they face challenges like: Image Resolution Variability: Inconsistent image resolutions can hinder performance. Contextual Nuance: Difficulty in capturing complex…
-
Building an Ideation Agent System with AutoGen: Create AI Agents that Brainstorm and Debate Ideas
Streamline Your Ideation Process with AI Ideation can be slow and complex. Imagine if two AI models could generate ideas and debate them. This tutorial shows you how to create an AI solution using two LLMs…
-
KGGen: Advancing Knowledge Graph Extraction with Language Models and Clustering Techniques
Understanding Knowledge Graphs and Their Challenges Knowledge graphs (KGs) are essential for AI applications, but they often lack important connections, making them less effective. Established KGs like DBpedia and Wikidata miss key entity relationships, which limits…
-
Steps to Build an Interactive Text-to-Image Generation Application using Gradio and Hugging Face’s Diffusers
Build an Interactive Text-to-Image Generator Overview In this tutorial, we will create a text-to-image generator using Google Colab, Hugging Face’s Diffusers library, and Gradio. This application will convert text prompts into detailed images using the advanced…
-
Breaking the Autoregressive Mold: LLaDA Proves Diffusion Models can Rival Traditional Language Architectures
Revolutionizing Language Models with LLaDA The world of large language models has typically relied on autoregressive methods, which predict text one word at a time from left to right. While effective, these methods have limitations in…
-
Microsoft Researchers Present Magma: A Multimodal AI Model Integrating Vision, Language, and Action for Advanced Robotics, UI Navigation, and Intelligent Decision-Making
Understanding Multimodal AI Agents Multimodal AI agents can handle different types of data like images, text, and videos. They are used in areas such as robotics and virtual assistants, allowing them to understand and act in…