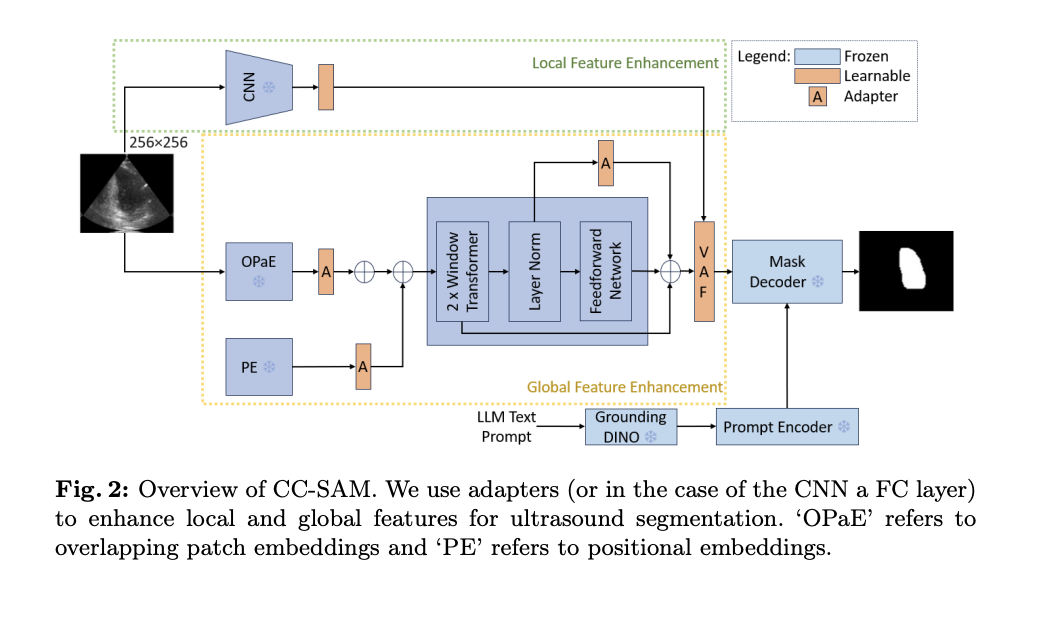
Practical Solutions in Medical Image Segmentation
Advances in Deep Learning
Deep learning has revolutionized medical image segmentation, improving accuracy and efficiency in clinical practice.
Challenges and Adaptations
Challenges in segmenting medical images, such as low contrast and faint boundaries, require specialized adaptations for improved performance.
Existing Models and Advancements
Models like U-Net and Segment Anything Model (SAM) have shown promise, but require modifications for medical image segmentation.
CC-SAM Model
CC-SAM, an advanced model building upon SAMUS, integrates a CNN with SAM’s ViT encoder to enhance segmentation performance for medical images.
Superior Segmentation Accuracy
CC-SAM demonstrates superior accuracy in various medical imaging datasets, highlighting its robustness and reliability across different tasks.
Innovative Techniques and Adaptability
The integration of CNN and ViT encoders, along with variational attention fusion and text prompts, enhances the adaptability and effectiveness of segmentation models in the medical field.
Evolve Your Company with AI
Identify Automation Opportunities
Locate customer interaction points that can benefit from AI to improve efficiency.
Define KPIs
Ensure measurable impacts on business outcomes with AI endeavors.
Select an AI Solution
Choose customizable tools that align with your company’s needs.
Implement Gradually
Start with a pilot, gather data, and expand AI usage judiciously to evolve your company.