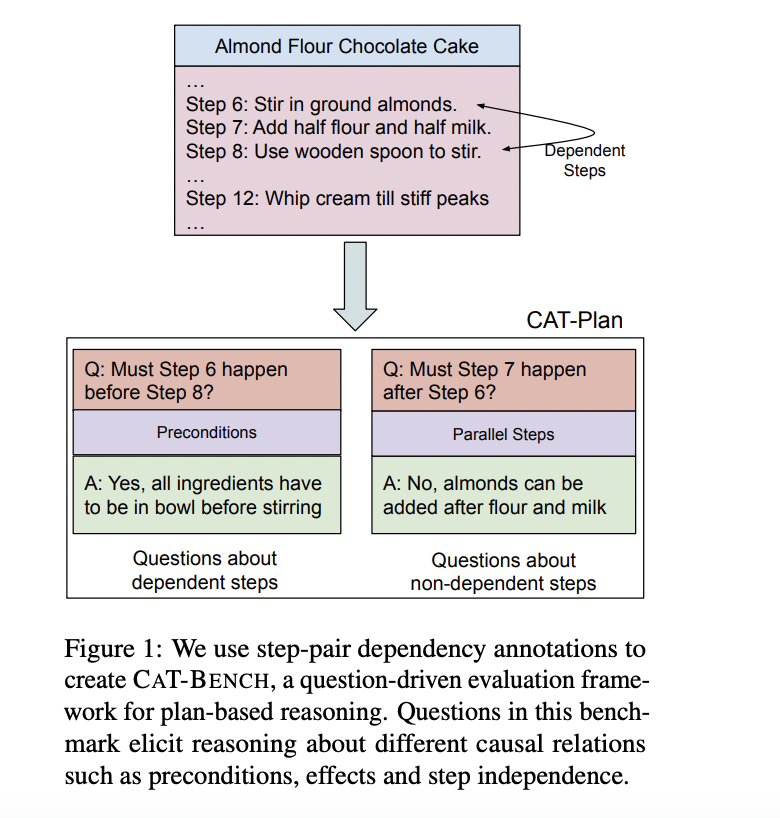
Understanding Temporal Dependencies in Procedural Texts
Practical Solutions and Value
Researchers have developed CAT-BENCH, a benchmark to evaluate advanced language models’ ability to predict the sequence of steps in cooking recipes. The study reveals challenges in comprehending causal and temporal relationships within instructional texts, emphasizing the need for improved language models.
Various models were evaluated on CAT-BENCH for their performance in predicting step dependencies. The benchmark introduces a new standard for evaluating the causal and temporal reasoning abilities of language models in understanding procedural texts like cooking recipes.
If you want to evolve your company with AI, stay competitive, use for your advantage CAT-BENCH: Evaluating Language Models’ Understanding of Temporal Dependencies in Procedural Texts.
AI Implementation Guidance
- Identify Automation Opportunities: Locate key customer interaction points that can benefit from AI.
- Define KPIs: Ensure your AI endeavors have measurable impacts on business outcomes.
- Select an AI Solution: Choose tools that align with your needs and provide customization.
- Implement Gradually: Start with a pilot, gather data, and expand AI usage judiciously.
For AI KPI management advice, connect with us at hello@itinai.com. And for continuous insights into leveraging AI, stay tuned on our Telegram t.me/itinainews or Twitter @itinaicom.
Discover how AI can redefine your sales processes and customer engagement. Explore solutions at itinai.com.