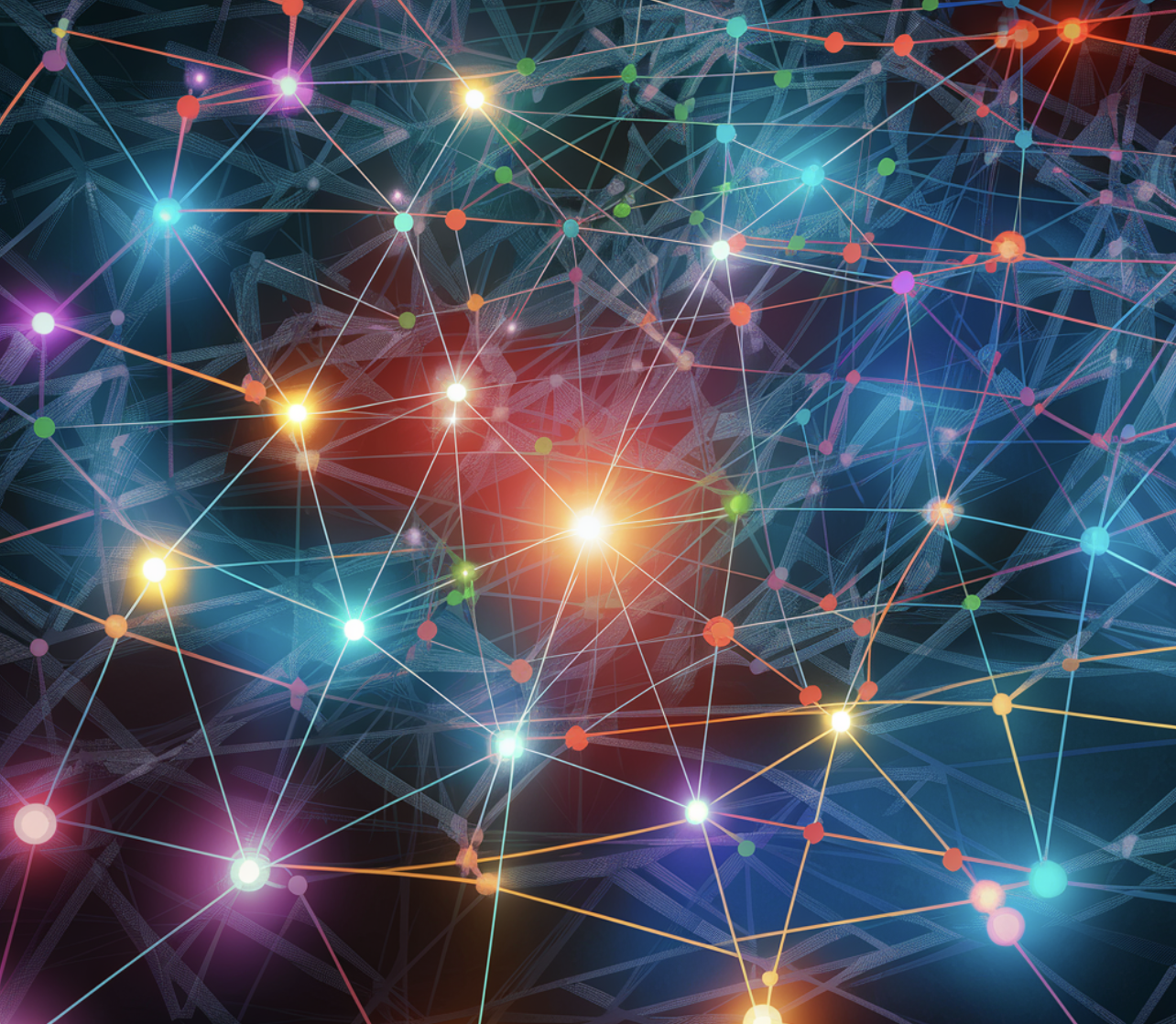
Capsule Networks: Addressing Limitations of Convolutional Neural Networks CNNs
Limitations of CNNs
CNNs lose spatial information and struggle with orientation sensitivity and high data requirements.
Capsule Networks: A Novel Approach
CapsNets address limitations through capsules, routing-by-agreement, and pose matrices to improve spatial awareness and robustness to transformations.
Benefits of Capsule Networks
CapsNets maintain spatial relationships, recognize objects regardless of orientation, and excel in part-to-whole recognition, improving object detection in cluttered environments.
Efficient Capsule Networks
Efforts to improve efficiency include the development of Efficient-CapsNet architecture and novel routing algorithms.
Challenges for CapsNets
CapsNets face challenges related to computational complexity and optimization and training of routing algorithms.
Conclusion
Capsule Networks provide a novel approach to addressing CNN limitations, with ongoing research aimed at enhancing performance and efficiency.
AI Solutions for Your Company
Discover how AI can redefine your way of work, identify automation opportunities, define KPIs, select an AI solution, and implement gradually. Connect with us at hello@itinai.com for AI KPI management advice.
Spotlight on a Practical AI Solution
Consider the AI Sales Bot from itinai.com/aisalesbot designed to automate customer engagement 24/7 and manage interactions across all customer journey stages.