Researchers from ETH Zurich have proposed modifications to simplify transformer blocks in deep neural networks without compromising training speed or performance. By combining signal propagation theory and empirical observations, they explored the removal of various components from standard transformer blocks. The proposed simplified transformers achieved comparable performance to standard transformers while using fewer parameters and experiencing a faster training throughput. Future research is recommended to investigate the effectiveness of these simplifications on larger transformer models and to conduct a comprehensive hyperparameter search.
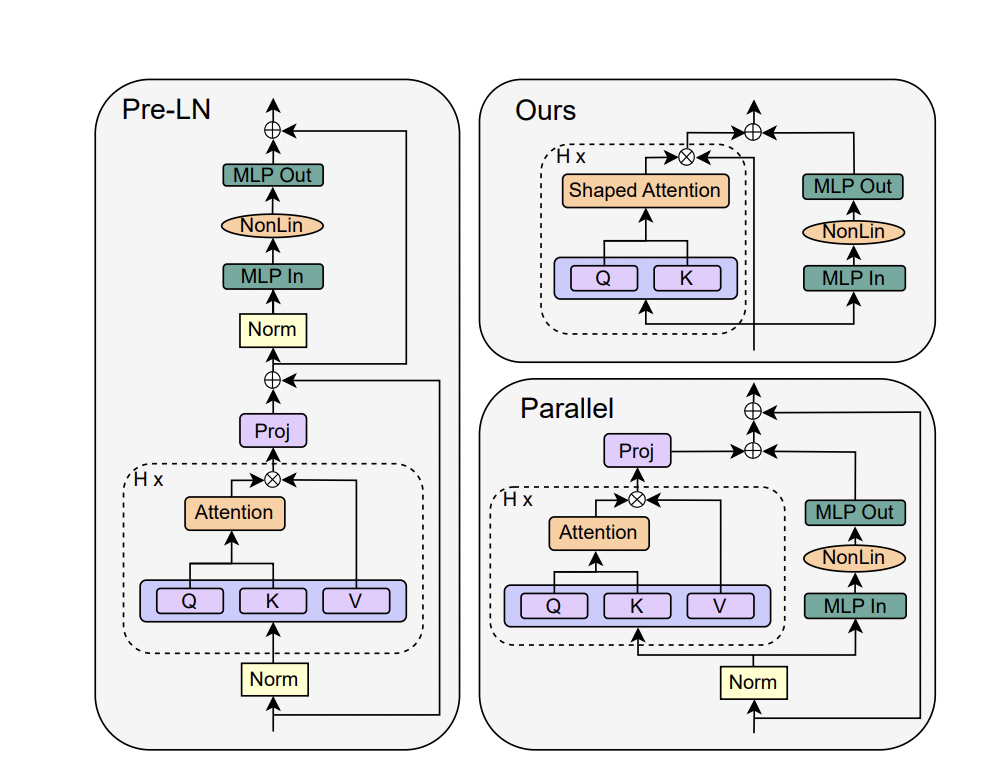
Can Transformer Blocks Be Simplified Without Compromising Efficiency?
Researchers from ETH Zurich have conducted a study on simplifying transformer blocks in deep neural networks. By combining signal propagation theory and empirical observations, they have proposed modifications that remove various components from standard transformer blocks without affecting training speed or performance.
Practical Solutions and Value:
- The study explores simplifications in transformer blocks to make them more robust and efficient.
- Modifications are proposed based on signal propagation theory and empirical observations.
- Components like skip connections, projection/value parameters, sequential sub-blocks, and normalization layers can be removed.
- The simplified transformers achieve comparable performance to standard transformers while using fewer parameters and experiencing faster training throughput.
- The research presents simplified deep-learning architectures that can reduce the cost of large transformer models.
- Proper initialization is emphasized for optimal results.
- Future research should investigate the effectiveness of the proposed simplifications on larger transformer models and conduct comprehensive hyperparameter search.
- Hardware-specific implementations of the simplified blocks can further improve training speed and performance.
Discover AI Solutions for Your Company:
- Identify Automation Opportunities: Locate key customer interaction points that can benefit from AI.
- Define KPIs: Ensure your AI endeavors have measurable impacts on business outcomes.
- Select an AI Solution: Choose tools that align with your needs and provide customization.
- Implement Gradually: Start with a pilot, gather data, and expand AI usage judiciously.
Spotlight on a Practical AI Solution:
Consider the AI Sales Bot from itinai.com/aisalesbot. It is designed to automate customer engagement 24/7 and manage interactions across all customer journey stages. Discover how AI can redefine your sales processes and customer engagement.