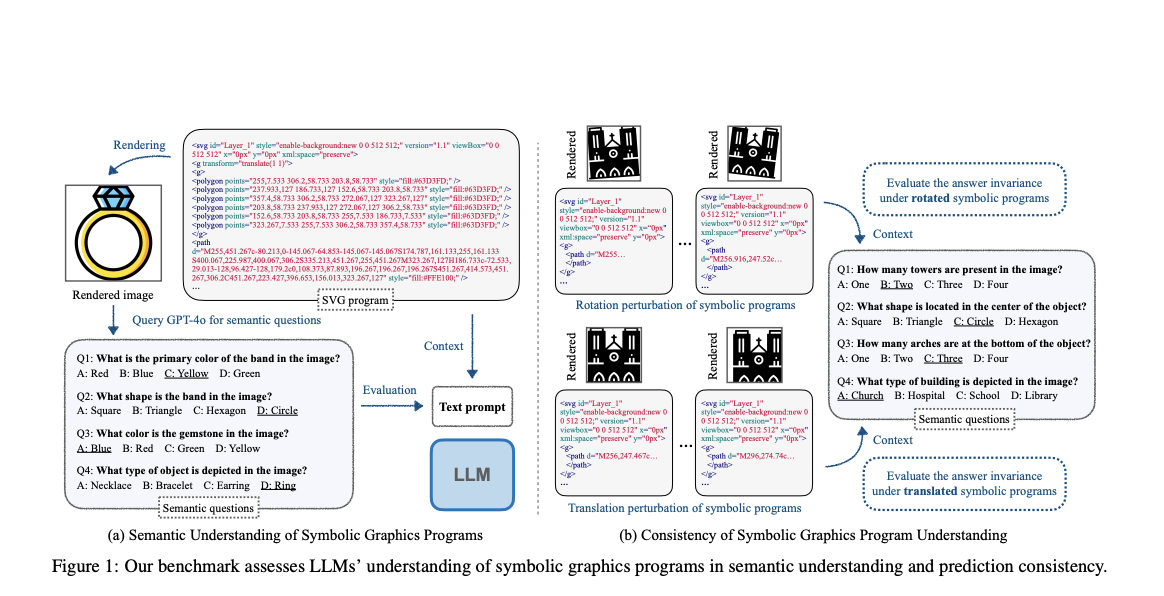
Assessing LLMs’ Understanding of Symbolic Graphics Programs in AI
Practical Solutions and Value
Large language models (LLMs) are being evaluated for their ability to understand symbolic graphics programs. This research aims to enhance LLMs’ interpretation of visual content generated from program text input, without direct visual input.
Proposed Benchmark and Methodology
Researchers have introduced SGP-Bench, a benchmark to evaluate LLMs’ semantic understanding of SVG and CAD programs. They also developed symbolic instruction tuning to enhance LLMs’ performance. The process involves using vision-language models to generate questions based on rendered images and human verification of these question-answer pairs.
Evaluation and Findings
The evaluation on the SGP-MNIST dataset revealed significant differences between human and LLM performance in interpreting symbolic programs. This highlights the challenge for machines to understand visual representations compared to humans.
Conclusion and Future Research
Researchers propose a new way to evaluate LLMs’ understanding of images from symbolic graphics programs and highlight the need for varied evaluation tasks. Future research includes investigating LLMs’ semantic understanding and developing advanced methods to improve their performance in these tasks.
AI Solutions for Business Transformation
Discover how AI can redefine your way of work and sales processes while identifying automation opportunities, defining KPIs, selecting AI solutions, and implementing them gradually for measurable impacts on business outcomes. Connect with us at hello@itinai.com for AI KPI management advice and stay tuned for continuous insights into leveraging AI on our Telegram t.me/itinainews or Twitter @itinaicom.