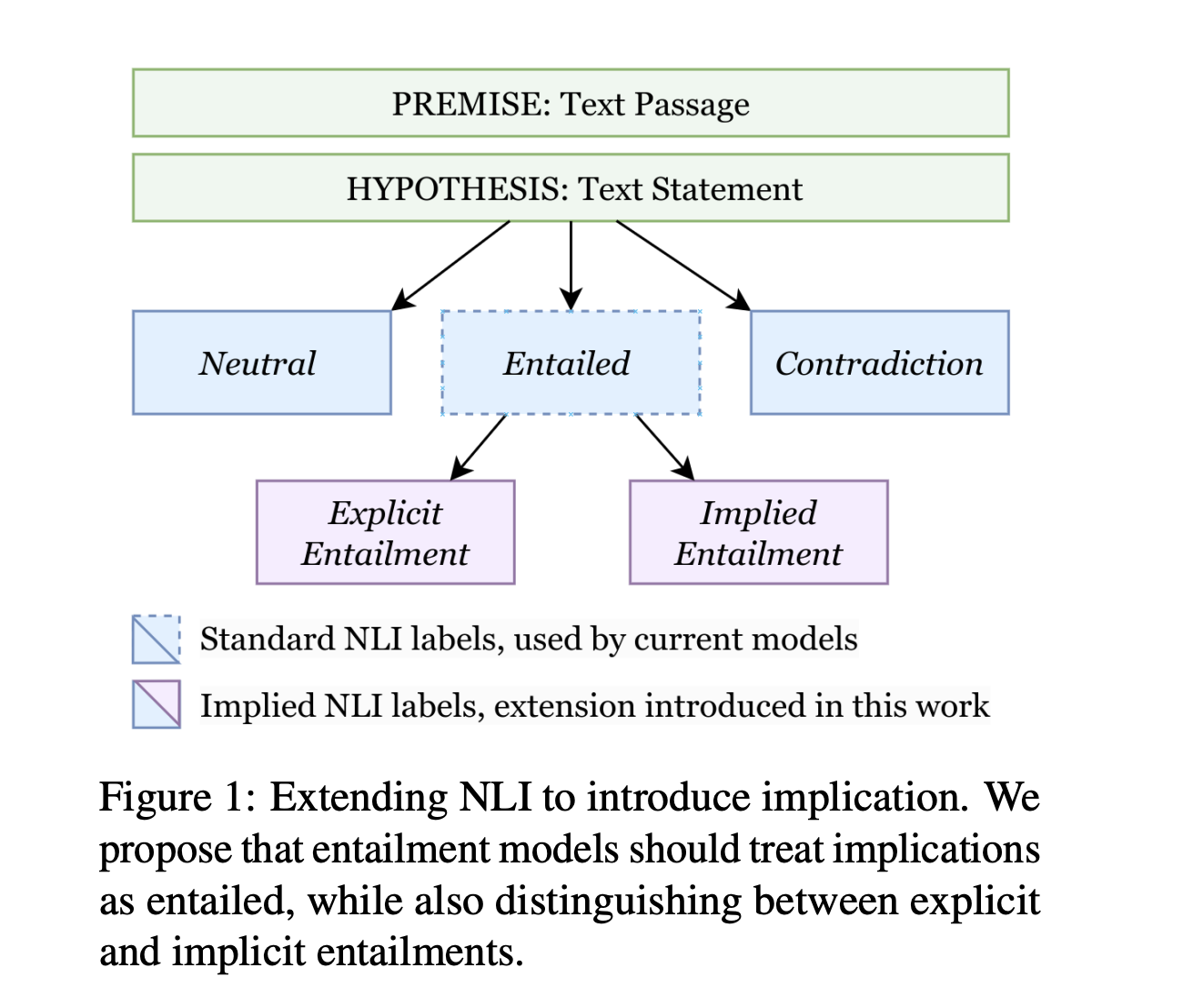
Understanding Implicit Meaning in Communication
Implicit meaning is crucial for effective human communication. However, many current Natural Language Inference (NLI) models struggle to recognize these implied meanings. Most existing NLI datasets focus on explicit meanings, leaving a gap in the ability to understand indirect expressions. This limitation affects applications like conversational AI, summarization, and context-sensitive decision-making, where inferring unspoken implications is essential.
The Challenge with Current NLI Models
Current benchmarks such as SNLI, MNLI, ANLI, and WANLI mainly feature explicit entailments, with implied entailments being very few. As a result, advanced models often misinterpret implied meanings as neutral or contradictory. Even large models like GPT-4 show a significant gap in detecting implicit entailments, highlighting the need for a better approach.
Introducing the Implied NLI (INLI) Dataset
Researchers from Google Deepmind and the University of Pennsylvania have developed the Implied NLI (INLI) dataset to address this issue. This dataset systematically incorporates implied meanings into NLI training by transforming existing structured datasets into pairs of premise and implied entailment. Each premise is also linked with explicit entailments, neutral statements, and contradictions, creating a comprehensive training resource.
Innovative Few-Shot Prompting Method
The INLI dataset uses a groundbreaking few-shot prompting method called Gemini-Pro. This method ensures the generation of high-quality implicit entailments while reducing costs and maintaining data integrity. By incorporating implicit meanings, models can differentiate between explicit and implicit entailments with greater accuracy.
Two-Stage Dataset Creation Process
The creation of the INLI dataset involves two stages:
- Restructuring existing datasets with implicatures into an implied entailment and premise format.
- Generating explicit entailments, neutral statements, and contradictions through controlled manipulation of the implied entailments.
The dataset includes 40,000 hypotheses for 10,000 premises, providing a diverse training set.
Significant Improvements in Model Performance
Models fine-tuned on the INLI dataset show a remarkable improvement in detecting implied entailments, achieving an accuracy of 92.5% compared to 50-71%% for models trained on typical NLI datasets. These models also generalize well to new datasets, scoring 94.5%% on NORMBANK and 80.4%% on SOCIALCHEM.
Contributions to Natural Language Inference
This research significantly advances NLI by introducing the INLI dataset, which enhances model accuracy in detecting implicit meanings. The structured approach and alternative hypothesis generation improve generalization across various domains, establishing a new benchmark for AI models in understanding nuanced communication.
Explore Further
Check out the Paper. All credit for this research goes to the researchers involved. Follow us on Twitter, join our Telegram Channel, and connect with our LinkedIn Group. Don’t forget to join our 70k+ ML SubReddit.
Transform Your Business with AI
Stay competitive and leverage AI to redefine your work processes. Here are some practical steps:
- Identify Automation Opportunities: Find key customer interactions that can benefit from AI.
- Define KPIs: Ensure your AI initiatives have measurable impacts.
- Select an AI Solution: Choose tools that fit your needs and allow customization.
- Implement Gradually: Start with a pilot project, gather data, and expand wisely.
For AI KPI management advice, connect with us at hello@itinai.com. For ongoing insights, follow us on Telegram or Twitter.
Enhance Your Sales and Customer Engagement
Discover how AI can transform your sales processes and customer interactions. Explore solutions at itinai.com.