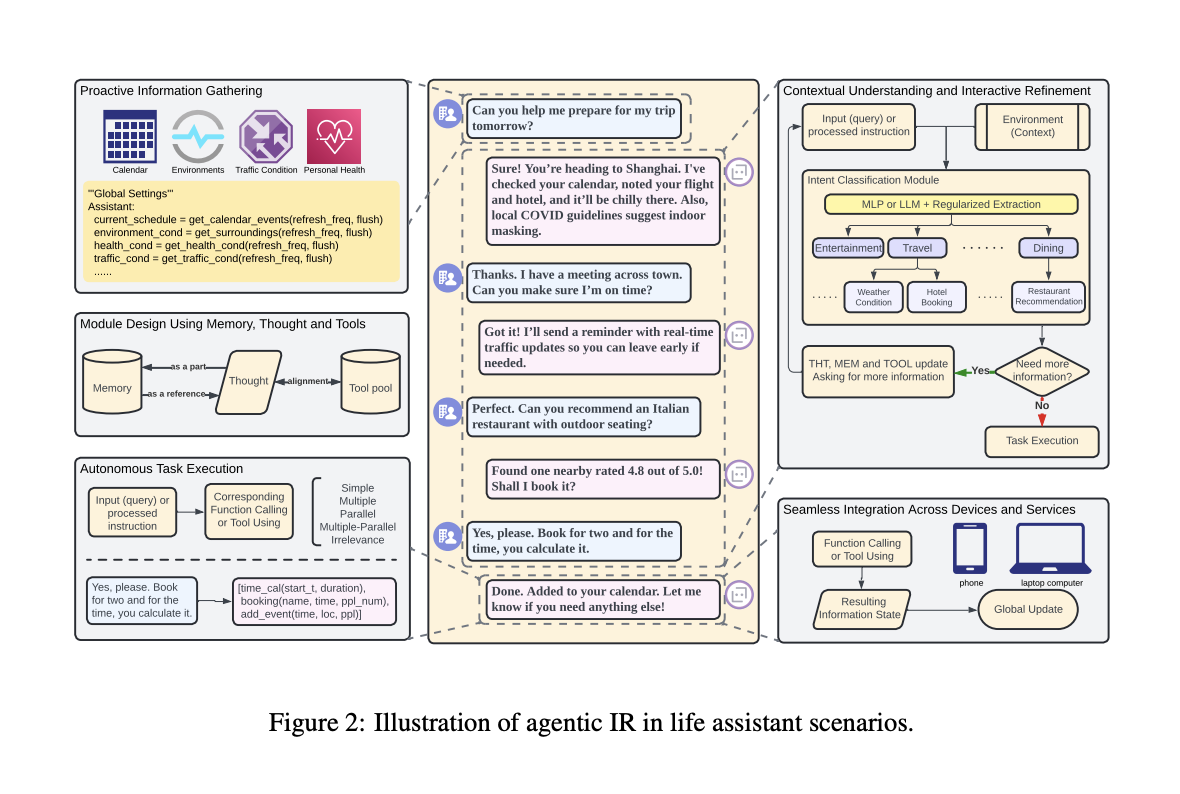
Challenges in Traditional Information Retrieval (IR)
Traditional IR systems struggle with complex tasks because they are built for single-step interactions. Users often have to modify their queries multiple times to get the right results. This makes current systems less effective for tasks that need real-time decision-making and iterative reasoning.
Limitations of Static Procedures
Most IR tasks, like web search and recommendations, use static methods such as indexing and filtering. These methods are effective for simple tasks but fall short in more complicated scenarios. Users have to repeatedly adjust queries, making the process inefficient and limited.
Introducing Agentic Information Retrieval
Researchers from Shanghai Jiao Tong University have developed Agentic Information Retrieval (Agentic IR). This new approach allows an AI-powered agent to interact dynamically, taking multiple actions to achieve user-defined goals. This means the agent can adapt to changing needs and provide more efficient information retrieval.
Benefits of Agentic IR
- Memory and Reasoning: The system remembers past interactions and reasons through complex tasks.
- Real-Time Data Use: It uses current data from search engines and databases to enhance performance.
- Flexible Problem-Solving: The agent can effectively assist in various tasks, from personal help to business intelligence.
Key Techniques Used
- Prompt Engineering: Generates inputs specific to tasks.
- Retrieval-Augmented Generation: Optimizes actions based on previous interactions.
- Reinforcement Learning: Improves decisions through real-time feedback.
Collaboration and Multi-Agent Systems
Agentic IR can also support multiple agents working together. This allows for better coordination and resource sharing, improving problem-solving capabilities across various domains.
Significant Improvements
Agentic IR shows substantial advancements in areas like personal assistance and programming support, achieving over 90% accuracy in complex tasks and reducing completion times by up to 40% compared to traditional methods. It excels in real-time decision-making and dynamic reasoning, enhancing user experience significantly.
Conclusion
Agentic IR represents a breakthrough in IR systems. By integrating dynamic, multi-step reasoning with memory and tools, it offers a flexible and adaptive approach to complex tasks. This innovation marks a crucial step in the evolution of intelligent agents and their role in information retrieval.
Check out the Paper. All credit for this research goes to the researchers of this project. Also, don’t forget to follow us on Twitter and join our Telegram Channel and LinkedIn Group. If you like our work, you will love our newsletter. Don’t forget to join our 55k+ ML SubReddit.
Upcoming Live Webinar- Oct 29, 2024
The Best Platform for Serving Fine-Tuned Models: Predibase Inference Engine.
Transform Your Business with AI: Discover how AI can reshape your operations.
- Identify Automation Opportunities: Find customer interaction points that benefit from AI.
- Define KPIs: Measure the impact of AI on your business outcomes.
- Select an AI Solution: Choose tools that meet your needs.
- Implement Gradually: Start small, gather data, and expand usage wisely.
For AI KPI management advice, connect with us at hello@itinai.com. For ongoing insights, stay tuned on our Telegram t.me/itinainews or Twitter @itinaicom.
Explore how AI can redefine your sales processes and customer engagement at itinai.com.