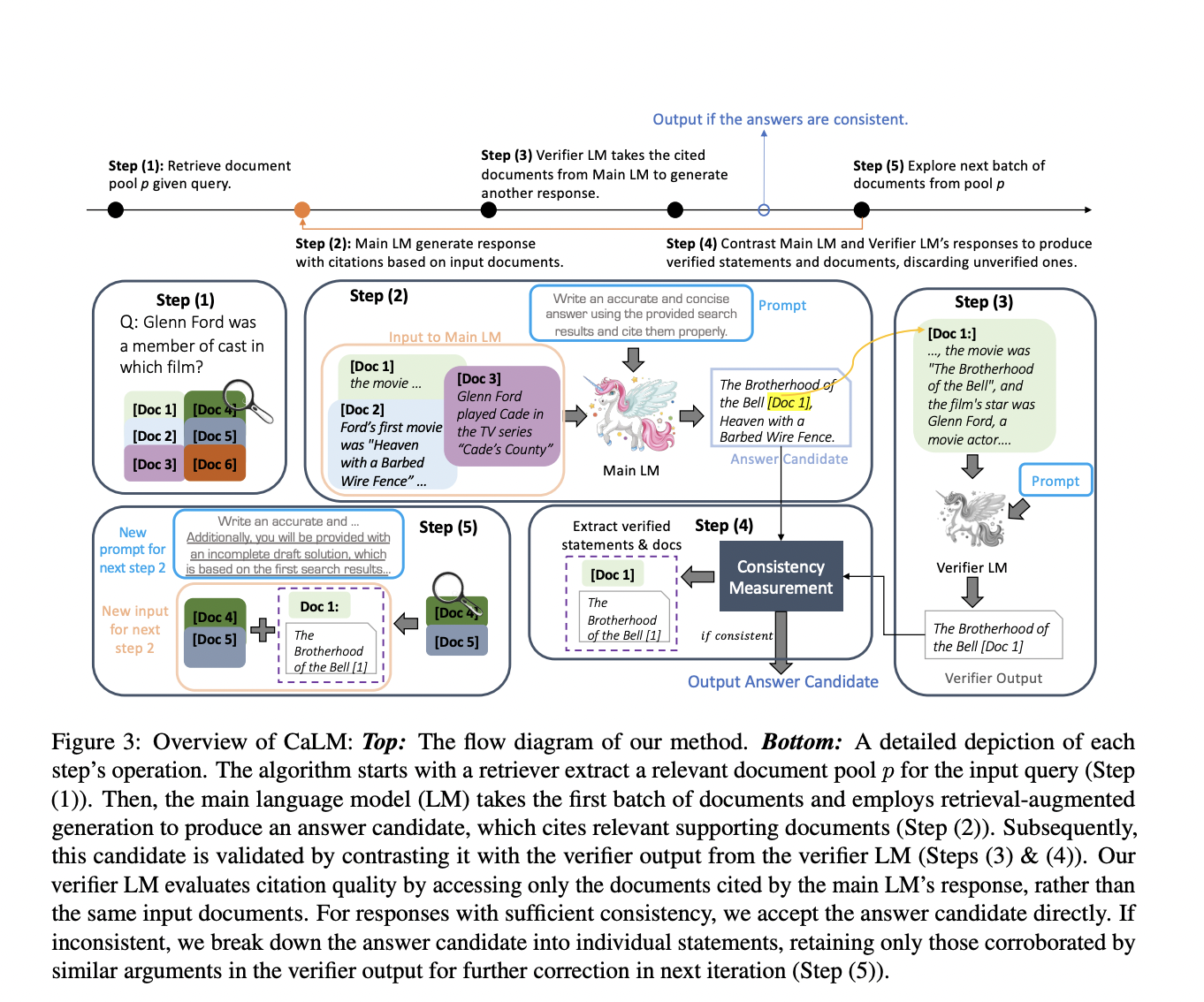
The Challenge
The challenge of ensuring large language models (LLMs) generate accurate, credible, and verifiable responses by correctly citing reliable sources is addressed in the paper.
Current Methods and Challenges
Existing methods often lead to incorrect or misleading information in generated responses due to errors and hallucinations. Standard approaches include retrieval-augmented generation and preprocessing steps, but they face challenges in maintaining accuracy and citation quality.
The Solution: CaLM Framework
The proposed solution, CaLM (Contrasting Large and Small Language Models), leverages the complementary strengths of large and small LMs. It employs a post-verification approach, where a smaller LM validates the outputs of a larger LM, significantly improving citation accuracy and overall answer quality without requiring model fine-tuning.
Performance Gains
Experiments demonstrated substantial performance gains using CaLM, outperforming state-of-the-art methods by 1.5% to 7% on average and proving robust even in challenging scenarios.
Value of CaLM
The CaLM framework effectively addresses the problem of ensuring accurate and verifiable responses from LLMs by leveraging the strengths of both large and small language models. It significantly improves the quality and reliability of LLM outputs, making it a valuable advancement in the field of language model research.
Benefits of AI Solutions
Discover how AI can redefine your company’s way of work and evolve your sales processes and customer engagement. Identify automation opportunities, define KPIs, select an AI solution, and implement gradually. For AI KPI management advice and continuous insights into leveraging AI, connect with us at hello@itinai.com.