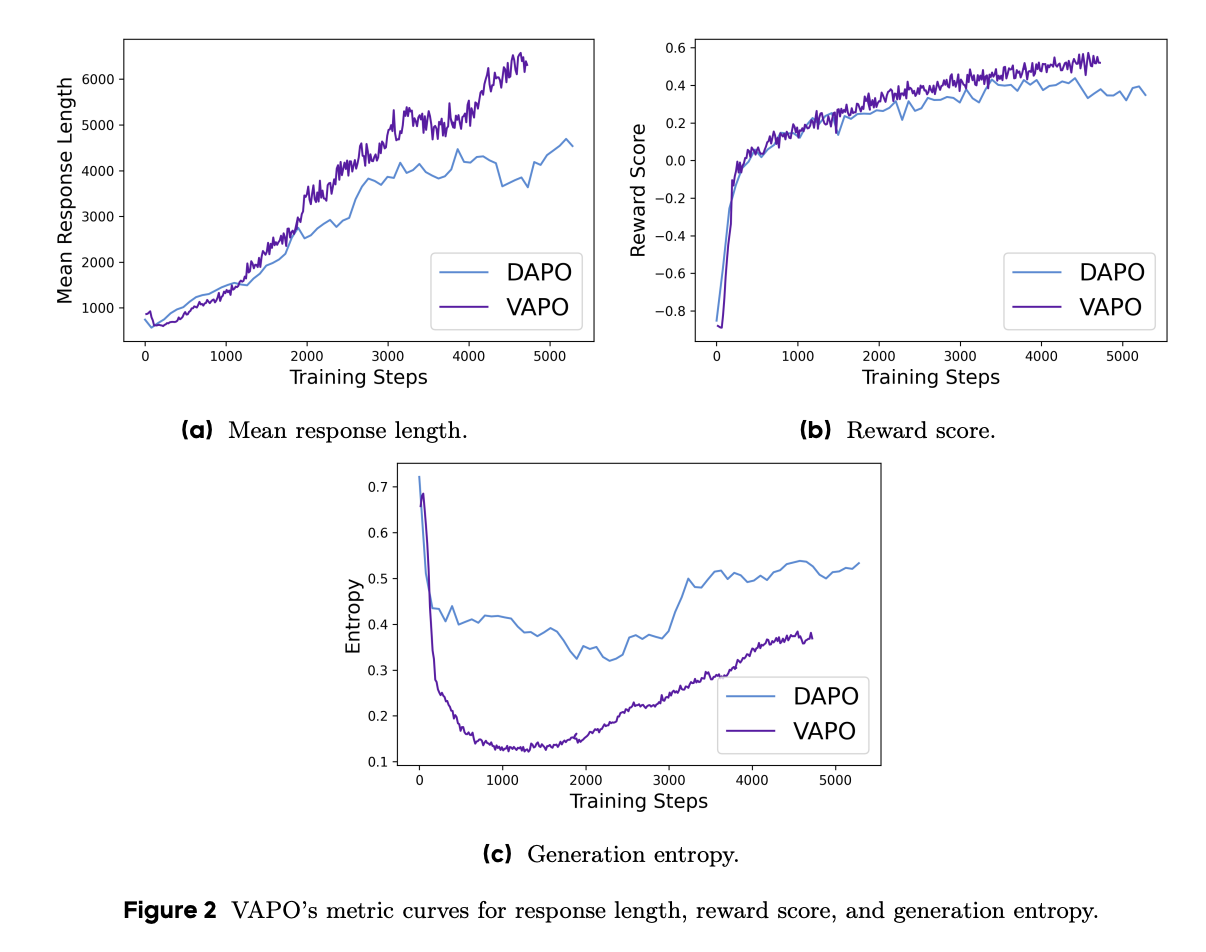
ByteDance Launches VAPO: A Groundbreaking Framework for Enhanced Reasoning in AI
Introduction to VAPO
ByteDance has unveiled VAPO, a novel reinforcement learning (RL) framework designed to tackle advanced reasoning tasks within large language models (LLMs). While traditional RL methods such as GRPO and DAPO have demonstrated effectiveness, VAPO leverages value-based techniques that enhance the precision of credit assignment, which is critical for complex reasoning scenarios.
Challenges in Current Value-Based Methods
Applying value-based reinforcement learning to long chain-of-thought (CoT) tasks presents three major challenges:
- Value Model Bias: Initializing value models with reward models can introduce positive bias, complicating accurate evaluations.
- Heterogeneous Sequence Lengths: Standard approaches struggle with varying response lengths, impacting effectiveness.
- Sparsity of Reward Signals: Tasks providing binary feedback can exacerbate difficulties in balancing exploration and exploitation.
Innovations Introduced by VAPO
To address these challenges, the researchers from ByteDance Seed have developed VAPO, which incorporates three innovative components:
- A comprehensive value-based training framework that enhances performance and efficiency.
- A Length-adaptive GAE mechanism that optimizes advantage estimation based on response length.
- A systematic integration of techniques from previous research to maximize collective improvements.
Utilizing the Qwen2.5-32B model, VAPO has shown remarkable improvements, increasing scores from 5 to 60, surpassing previous state-of-the-art methods by 10 points.
Performance Analysis of VAPO
The VAPO framework builds upon the PPO algorithm, featuring modifications that enhance mathematical reasoning capabilities. Key performance metrics reveal:
- Smoother training curves, indicating more stable optimization.
- Better length scaling, which improves generalization.
- Faster score growth due to granular signals from the value model.
- Lower entropy in later training stages, balancing exploration with stability.
In direct comparisons, while DeepSeek R1 using GRPO scored 47 points and DAPO achieved 50 points, VAPO reached a new high of 60.4 points with only 5,000 update steps, demonstrating its efficiency and effectiveness.
Impact of VAPO’s Innovations
Ablation studies confirm the efficacy of seven key modifications that VAPO implements:
- Value-Pretraining prevents model collapse.
- Decoupled GAE allows for optimal long-form response optimization.
- Adaptive GAE balances short and long responses effectively.
- Clip-higher encourages thorough exploration.
- Token-level loss increases weighting for long responses.
- Positive-example LM loss contributes an additional 6 points.
- Group-Sampling adds 5 points to overall performance.
Conclusion
The introduction of VAPO represents a significant advancement in value-based reinforcement learning for reasoning tasks. By addressing fundamental challenges in training value models for long CoT scenarios, VAPO not only refines value learning but also establishes a new performance benchmark for LLMs in reasoning-intensive applications. This framework offers a robust foundation for future developments in artificial intelligence.