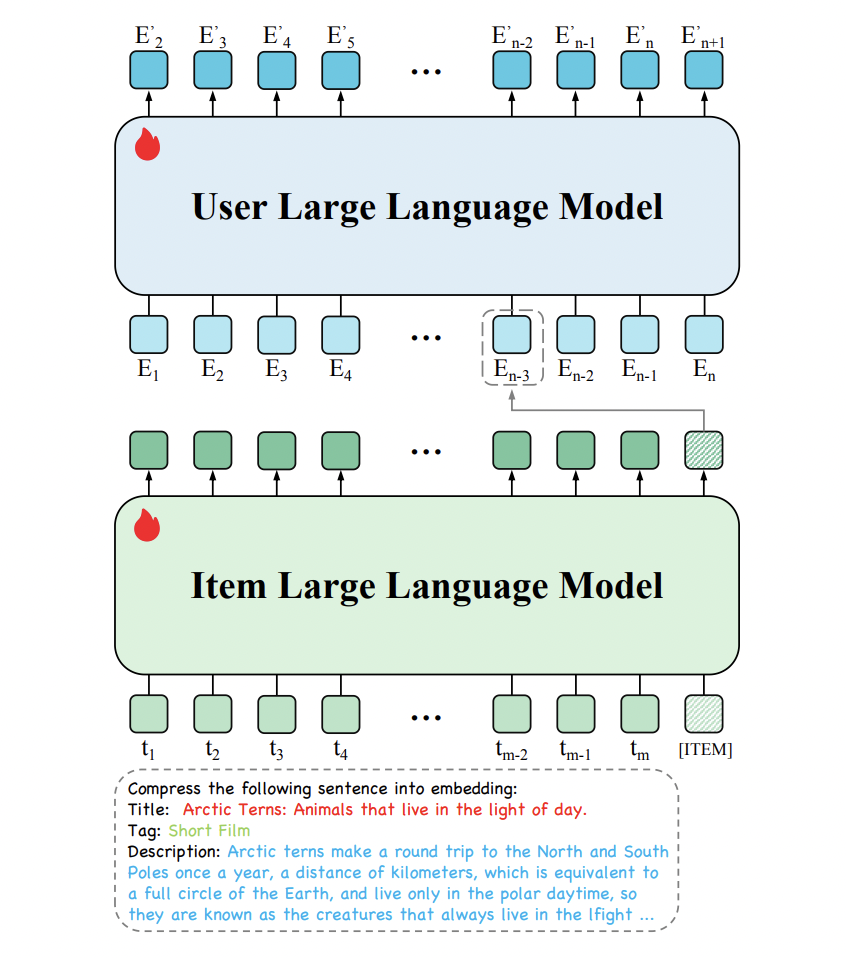
Practical Solutions for Enhanced Recommendations
Enhancing Recommendation Systems with HLLM Architecture
Recommendation systems are crucial for personalized experiences in various platforms. They predict user preferences by analyzing interactions, offering relevant suggestions. Developing advanced algorithms is key for accurate recommendations in large datasets.
Addressing Cold-Start Challenges
Recommendation systems face issues with new users and items, affecting prediction accuracy. HLLM introduces a two-tier approach using large language models to extract rich content features, improving user interest prediction and item feature extraction.
Improving Model Efficiency
HLLM’s hierarchical architecture reduces computational complexity by separating item and user modeling. It outperforms traditional models in cold-start scenarios and achieves state-of-the-art performance, showcasing better scalability and efficiency.
Revolutionizing Recommendation Technology
The successful integration of HLLM in recommendation systems offers superior performance, especially in real-world applications. Leveraging pre-trained knowledge and fine-tuning tasks result in more efficient and scalable solutions compared to traditional methods.