Machine learning (ML) is increasingly important across industries, but there is a gap between business expectations and what engineers and data scientists can deliver. The first step to close this gap is fostering honest dialogue between teams and then democratizing ML across the organization. Companies can enable collaboration between technical and non-technical teams, provide the necessary tools and infrastructure, empower employees with access to data and user-friendly tools, offer training programs, and measure and celebrate the success of ML initiatives. This will lead to better decision-making and innovation.
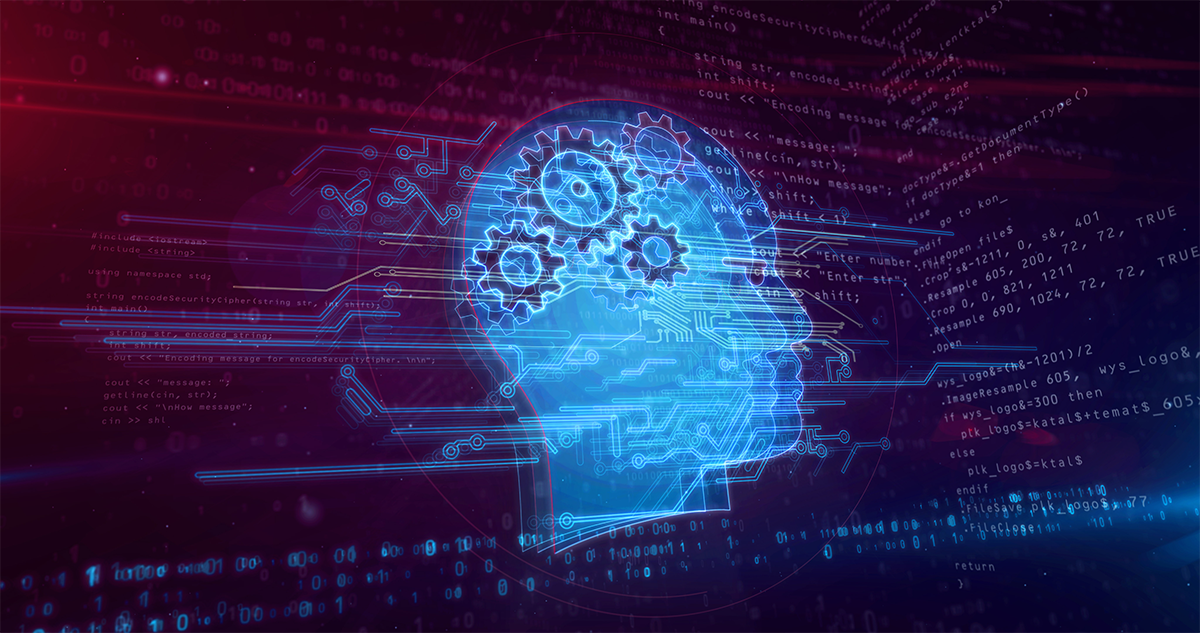
Bridging the Expectation-Reality Gap in Machine Learning
Machine learning (ML) is now critical in every industry, but there is often a disconnect between business leaders’ expectations and what engineers and data scientists can deliver. Business leaders want wide-scale ML deployment, but data scientists lack the necessary tools and face complex challenges.
To bridge this gap, fostering honest dialogue between teams is crucial. Democratizing ML across the organization is the next step, giving both technical and non-technical teams access to powerful ML tools and continuous learning. Non-technical teams can benefit from user-friendly data visualization tools, while data scientists need robust development platforms and cloud infrastructure.
Enabling collaboration between tech, product, and design teams is essential for success. Companies should provide the necessary tools and infrastructure for ML development, avoiding delays in deployment. Empowering employees by granting them access to data and offering no-code/low-code tools for data analysis can turn any company into a data-driven organization.
Upskilling programs and training opportunities are crucial for employees to learn new skills. Measuring the success of ML democratization initiatives and continuously improving areas that need work is important. Collaborative teamwork and measurable accountability are key to success.
By democratizing ML, companies can spread data-driven decision-making throughout the organization, leading to better products and services that delight customers. Implementing best practices to democratize ML will help build a future-forward organization that innovates with powerful data insights.
Practical AI Solutions
If you want to evolve your company with AI, consider the following:
- Identify Automation Opportunities: Locate key customer interaction points that can benefit from AI.
- Define KPIs: Ensure your AI endeavors have measurable impacts on business outcomes.
- Select an AI Solution: Choose tools that align with your needs and provide customization.
- Implement Gradually: Start with a pilot, gather data, and expand AI usage judiciously.
For AI KPI management advice, connect with us at hello@itinai.com. Stay tuned for continuous insights into leveraging AI on our Telegram channel or Twitter @itinaicom.
Spotlight on a Practical AI Solution: AI Sales Bot
Consider the AI Sales Bot from itinai.com/aisalesbot. It is designed to automate customer engagement 24/7 and manage interactions across all customer journey stages.
Discover how AI can redefine your sales processes and customer engagement. Explore solutions at itinai.com.