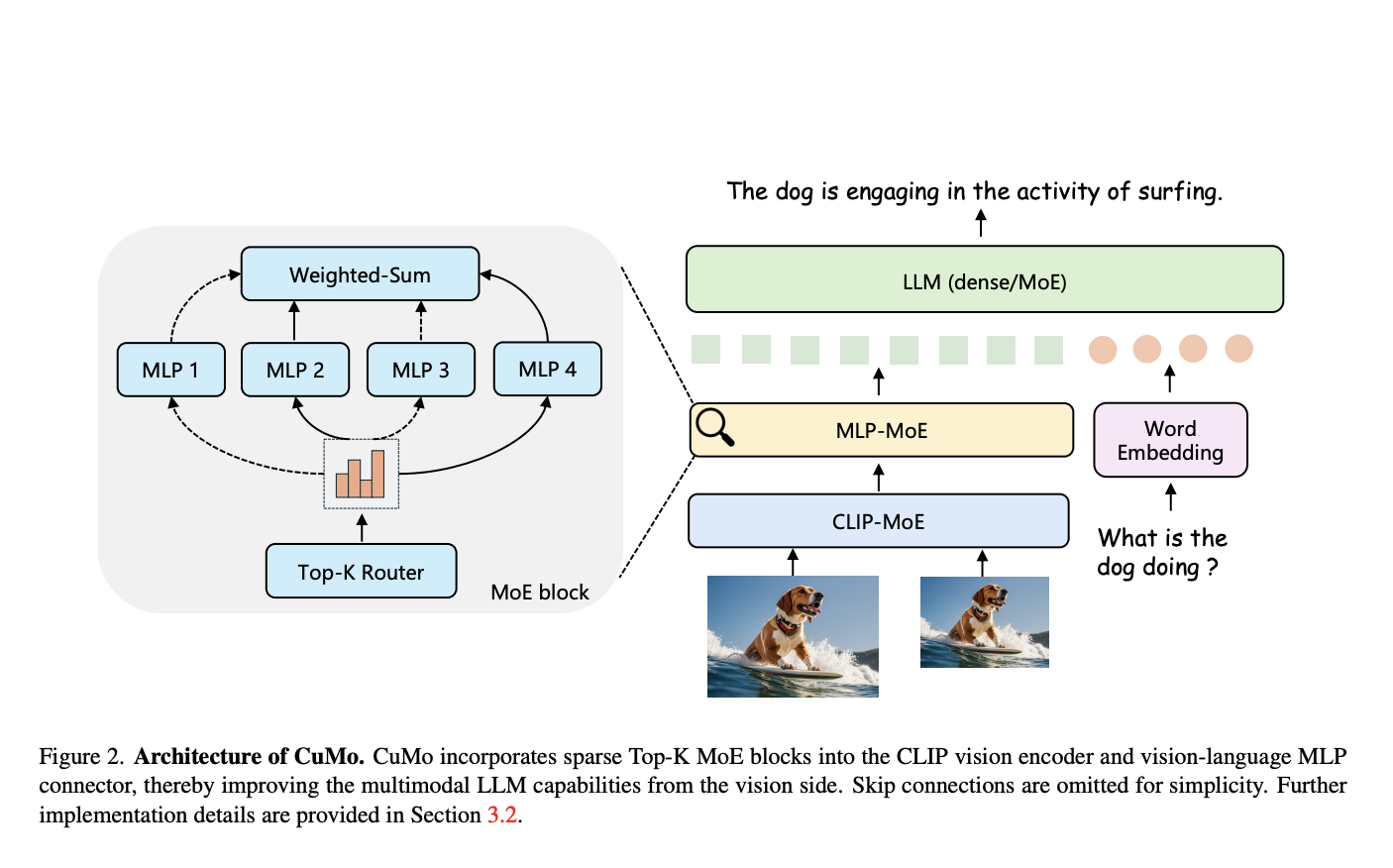
The Value of CuMo in Scaling Multimodal AI
Enhancing Multimodal Capabilities
The integration of sparse MoE blocks into the vision encoder and vision-language connector of a multimodal LLM allows for parallel processing of visual and text inputs, leading to more efficient scaling.
Co-upcycling Innovation
The concept of co-upcycling initializes sparse MoE modules from a pre-trained dense model, providing a better starting point for experts to specialize during training.
Comprehensive Training Approach
The three-stage training process of CuMo includes pre-training the vision-language connector, pre-finetuning all model parameters jointly, and fine-tuning with visual instruction data, resulting in efficient scaling compared to increasing model size.
Impressive Performance
CuMo models outperformed state-of-the-art approaches within the same model size categories across various benchmarks, showcasing the potential of sparse MoE architectures combined with co-upcycling in developing efficient multimodal AI assistants.
Practical AI Solutions for Business
Identify Automation Opportunities
Locate customer interaction points that can benefit from AI and automate processes to enhance efficiency.
Define Measurable KPIs
Ensure AI endeavors have measurable impacts on business outcomes to track and optimize performance.
Select Customizable AI Tools
Choose AI solutions that align with specific business needs and provide customization options for seamless integration.
Gradual Implementation
Start with a pilot AI project, gather data, and expand AI usage judiciously to maximize benefits.
Spotlight on AI Sales Bot
Explore the AI Sales Bot from itinai.com/aisalesbot designed to automate customer engagement 24/7 and manage interactions across all customer journey stages.