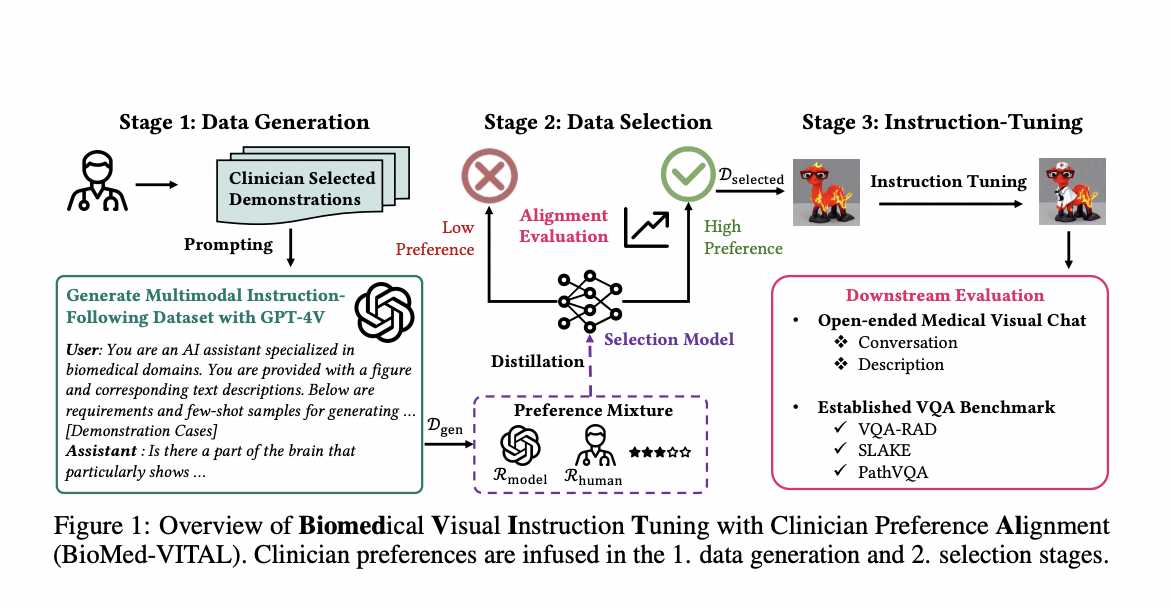
<>
Practical Solutions and Value of BioMed-VITAL Framework
Enhancing Biomedical Visual Instruction Tuning
Recent advancements in AI models like GPT-4V have shown great performance in various tasks. However, adapting them to specialized fields like biomedicine requires specific datasets. BioMed-VITAL integrates clinician preferences to generate high-quality data for these models.
Improving Model Performance
BioMed-VITAL significantly boosts model performance, achieving an 18.5% improvement in open visual chat and an 81.73% win rate in biomedical visual question answering. This framework aligns expert knowledge with model training, leading to superior results.
Data-Centric Approach
The three-stage process of data generation, data selection, and instruction tuning ensures that the model is fine-tuned effectively for specialized biomedical tasks. By incorporating clinician expertise, BioMed-VITAL enhances the model’s performance and accuracy.
Alignment with Clinician Preferences
BioMed-VITAL generates high-quality datasets by aligning closely with clinician preferences. This alignment ensures that the model learns from relevant and expert-approved data, leading to improved outcomes in medical visual chat and question answering tasks.
>