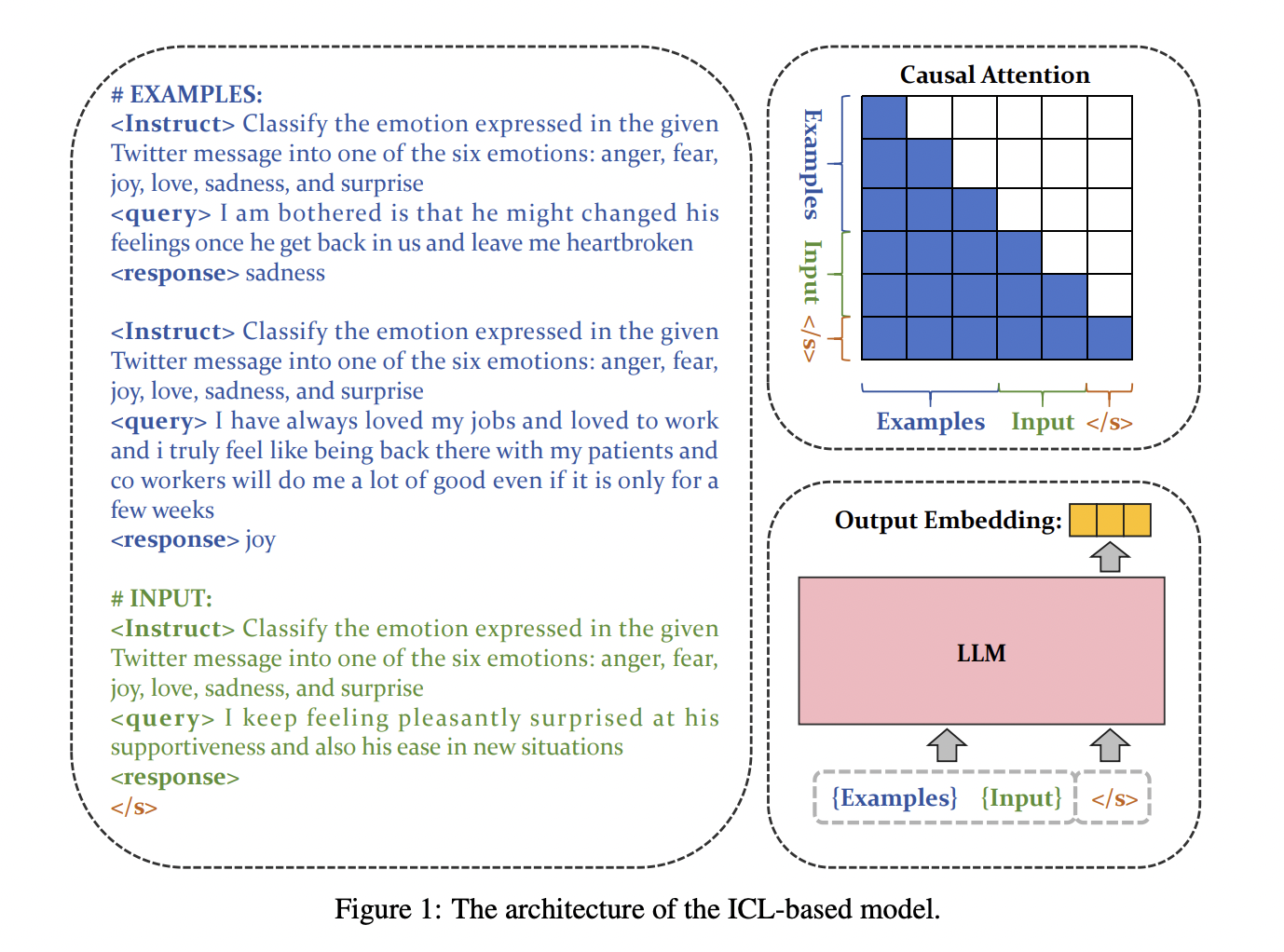
Practical Solutions and Value of ‘bge-en-icl’ AI Model
Enhancing Text Embeddings for Real-World Applications
Generating high-quality text embeddings for diverse tasks in natural language processing (NLP) is crucial for AI advancements. Existing models face challenges in adapting dynamically to new tasks and contexts, limiting their real-world applicability.
The ‘bge-en-icl’ model introduces in-context learning (ICL) to enhance text embeddings without complex modifications. By integrating task-specific examples directly into the query input, the model generates more relevant and generalizable embeddings across various tasks.
Key Features:
- Utilizes Mistral-7B backbone for NLP tasks
- Employs in-context learning during training for task-specific embeddings
- Trained using contrastive loss function for optimal similarity
- Tested on benchmarks like MTEB and AIR-Bench, excelling in few-shot learning scenarios
Benefits:
- Outperforms other models in retrieval, classification, and clustering tasks
- Highly effective in generating relevant and adaptable embeddings
- Sets new performance benchmarks across diverse tasks
By leveraging in-context learning, the ‘bge-en-icl’ model showcases significant advancements in text embedding generation, offering state-of-the-art performance and broad applicability in real-world scenarios.
For more information, visit the Paper and GitHub of the project.