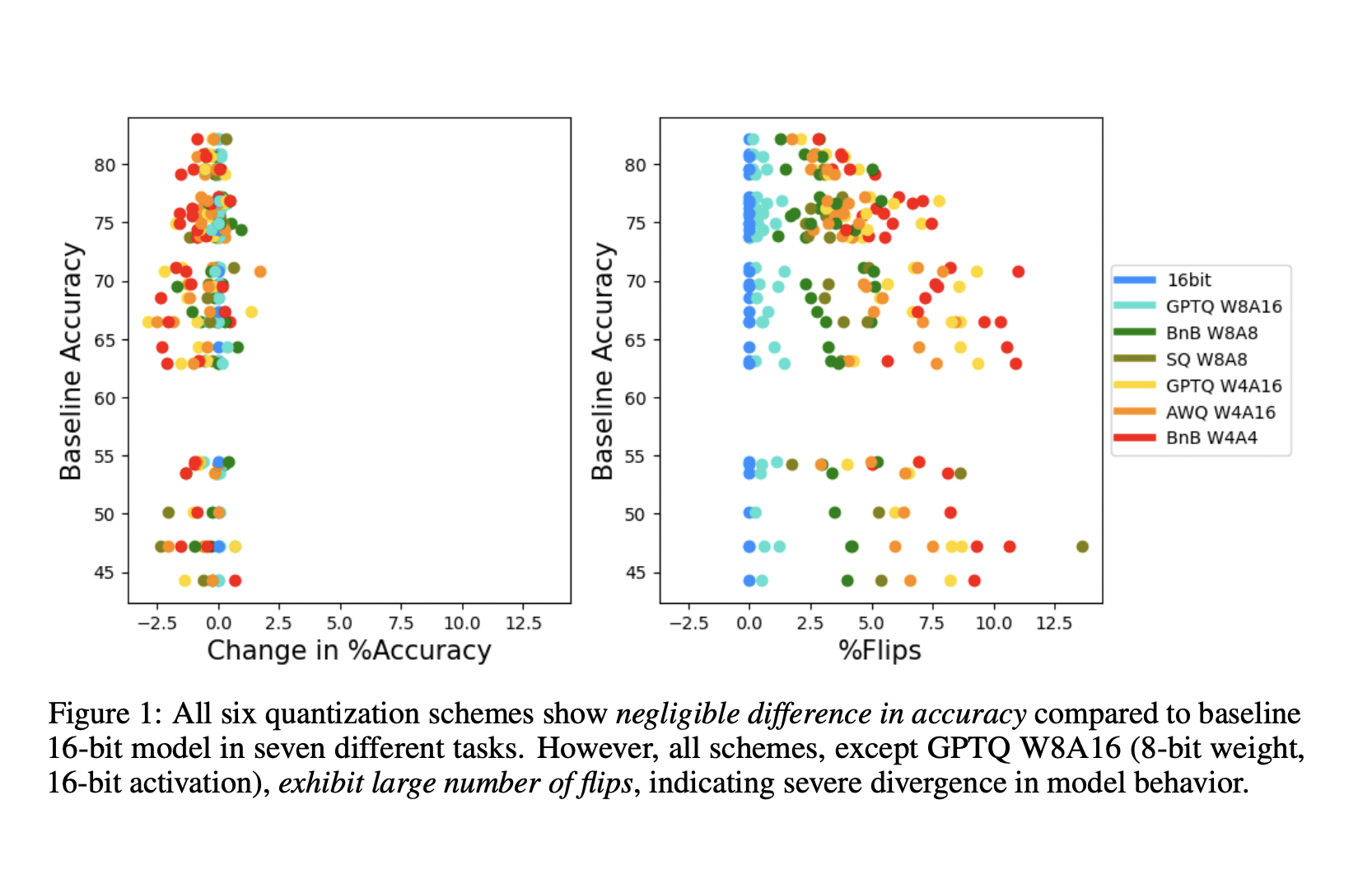
Evaluating LLM Compression Techniques
Introduction
Evaluating the effectiveness of Large Language Model (LLM) compression techniques is crucial for optimizing efficiency, reducing computational costs, and latency.
Challenges
Traditional evaluation practices focus primarily on accuracy metrics, overlooking changes in model behavior, such as “flips”, impacting the reliability of compressed models in critical applications like medical diagnosis and autonomous driving.
Proposed Approach
Introducing distance metrics such as KL-Divergence and % flips in addition to traditional accuracy metrics provides a more comprehensive evaluation of compressed models’ reliability and applicability across various tasks.
Research Findings
The study reveals that the percentage of flips can indicate significant divergence in model behavior, with larger models showing greater resilience to compression. The use of flips and KL-Divergence metrics effectively captures nuanced performance changes in compressed models.
Conclusion
This proposed method addresses the limitations of traditional accuracy metrics, ensuring that compressed models maintain high standards of reliability and applicability. It makes a significant contribution to AI research by proposing a more comprehensive evaluation framework for LLM compression techniques.
AI Solutions for Business
If you want to evolve your company with AI, stay competitive, and use AI for your advantage, discover how AI can redefine your way of work. Connect with us for AI KPI management advice and continuous insights into leveraging AI for your business outcomes.