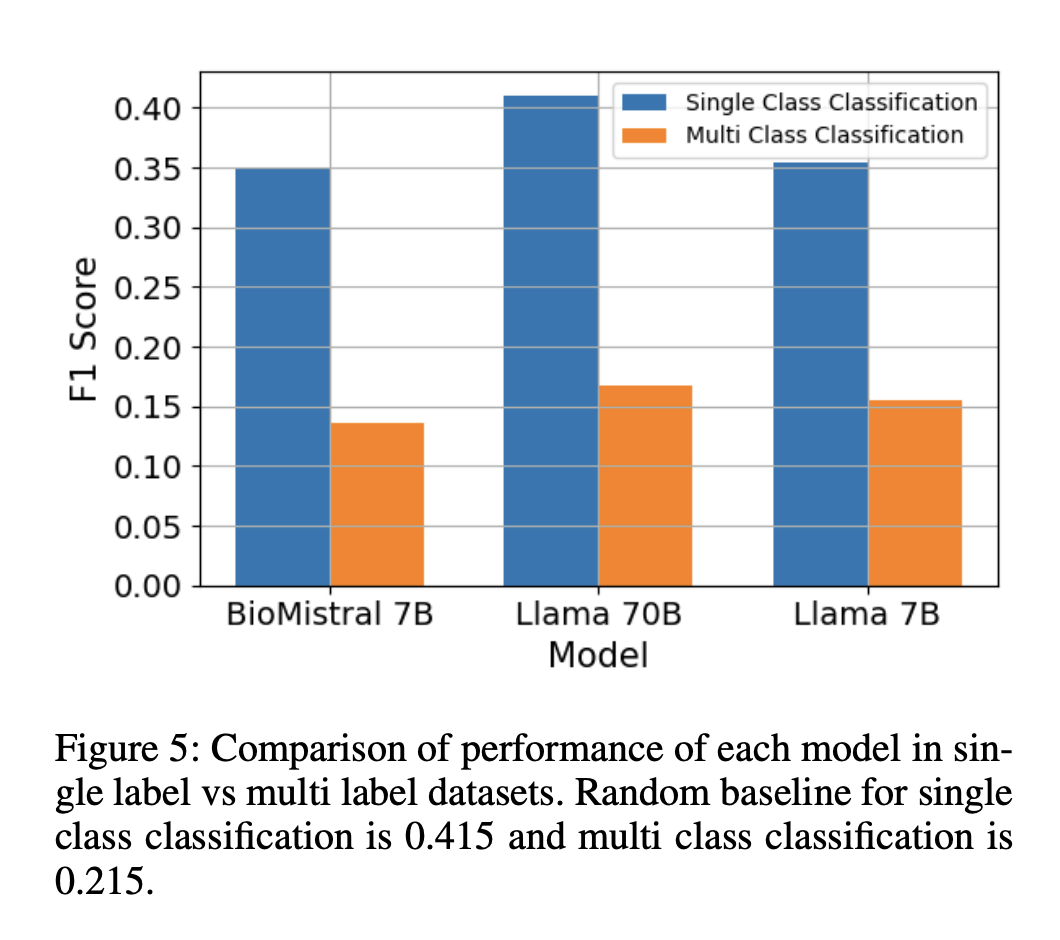
Practical Solutions and Value of Benchmarking Large Language Models in Biomedical Classification and Named Entity Recognition
Research Findings
LLMs in healthcare are increasingly effective for tasks like question answering and document summarization, performing on par with domain experts.
Standard prompting outperforms complex techniques like Chain-of-Thought (CoT) reasoning and Retrieval-Augmented Generation (RAG) in medical classification and Named Entity Recognition (NER) tasks.
Effective integration of external knowledge in LLMs is crucial for real-world applications in healthcare.
Standard Prompting consistently yields the highest F1 scores for classification tasks across all models.
Insights and Recommendations
LLMs face limitations in generalizability and effectiveness in structured biomedical information extraction, requiring better translation of advanced prompting methods to biomedical tasks.
The study emphasizes the need to integrate domain-specific knowledge and reasoning capabilities to enhance LLM performance in real-world healthcare applications.
AI Solutions for Business Evolution
Identify Automation Opportunities: Locate key customer interaction points that can benefit from AI.
Define KPIs: Ensure AI endeavors have measurable impacts on business outcomes.
Select an AI Solution: Choose tools that align with your needs and provide customization.
Implement Gradually: Start with a pilot, gather data, and expand AI usage judiciously.
For AI KPI management advice, connect with us at hello@itinai.com.
For continuous insights into leveraging AI, stay tuned on our Telegram t.me/itinainews or Twitter @itinaicom.
Explore AI solutions at itinai.com to redefine your sales processes and customer engagement.